16.1 Traveling Waves
Learning objectives.
By the end of this section, you will be able to:
- Describe the basic characteristics of wave motion
- Define the terms wavelength, amplitude, period, frequency, and wave speed
- Explain the difference between longitudinal and transverse waves, and give examples of each type
- List the different types of waves
We saw in Oscillations that oscillatory motion is an important type of behavior that can be used to model a wide range of physical phenomena. Oscillatory motion is also important because oscillations can generate waves, which are of fundamental importance in physics. Many of the terms and equations we studied in the chapter on oscillations apply equally well to wave motion ( (Figure) ).
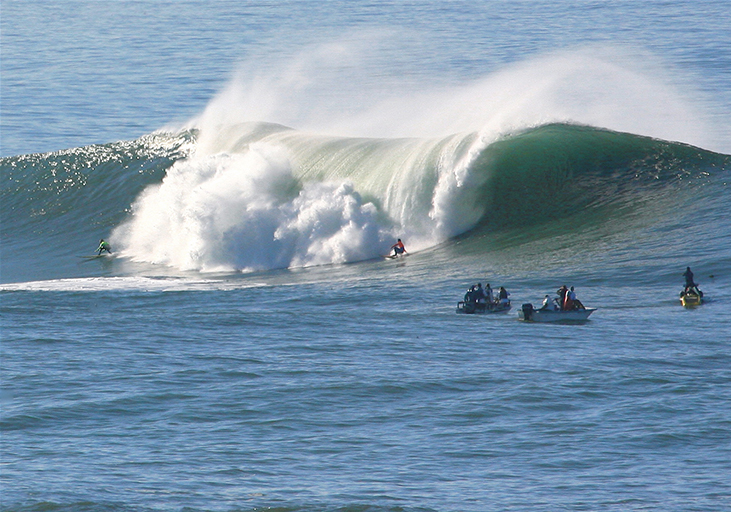
Figure 16.2 From the world of renewable energy sources comes the electric power-generating buoy. Although there are many versions, this one converts the up-and-down motion, as well as side-to-side motion, of the buoy into rotational motion in order to turn an electric generator, which stores the energy in batteries.
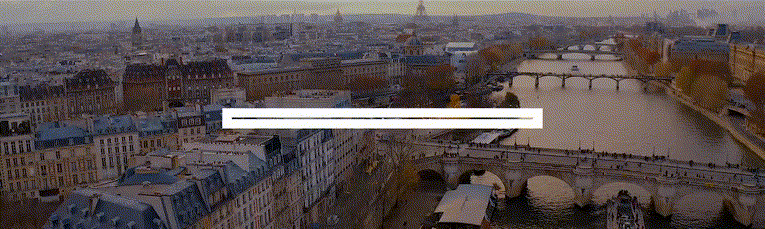
Types of Waves
A wave is a disturbance that propagates, or moves from the place it was created. There are three basic types of waves: mechanical waves, electromagnetic waves, and matter waves.
Basic mechanical waves are governed by Newton’s laws and require a medium. A medium is the substance a mechanical waves propagates through, and the medium produces an elastic restoring force when it is deformed. Mechanical waves transfer energy and momentum, without transferring mass. Some examples of mechanical waves are water waves, sound waves, and seismic waves. The medium for water waves is water; for sound waves, the medium is usually air. (Sound waves can travel in other media as well; we will look at that in more detail in Sound .) For surface water waves, the disturbance occurs on the surface of the water, perhaps created by a rock thrown into a pond or by a swimmer splashing the surface repeatedly. For sound waves, the disturbance is a change in air pressure, perhaps created by the oscillating cone inside a speaker or a vibrating tuning fork. In both cases, the disturbance is the oscillation of the molecules of the fluid. In mechanical waves, energy and momentum transfer with the motion of the wave, whereas the mass oscillates around an equilibrium point. (We discuss this in Energy and Power of a Wave .) Earthquakes generate seismic waves from several types of disturbances, including the disturbance of Earth’s surface and pressure disturbances under the surface. Seismic waves travel through the solids and liquids that form Earth. In this chapter, we focus on mechanical waves.
Electromagnetic waves are associated with oscillations in electric and magnetic fields and do not require a medium. Examples include gamma rays, X-rays, ultraviolet waves, visible light, infrared waves, microwaves, and radio waves. Electromagnetic waves can travel through a vacuum at the speed of light, [latex] v=c=2.99792458\,×\,{10}^{8}\,\text{m/s}. [/latex] For example, light from distant stars travels through the vacuum of space and reaches Earth. Electromagnetic waves have some characteristics that are similar to mechanical waves; they are covered in more detail in Electromagnetic Waves in volume 2 of this text.
Matter waves are a central part of the branch of physics known as quantum mechanics. These waves are associated with protons, electrons, neutrons, and other fundamental particles found in nature. The theory that all types of matter have wave-like properties was first proposed by Louis de Broglie in 1924. Matter waves are discussed in Photons and Matter Waves in the third volume of this text.
Mechanical Waves
Mechanical waves exhibit characteristics common to all waves, such as amplitude, wavelength, period, frequency, and energy. All wave characteristics can be described by a small set of underlying principles.
The simplest mechanical waves repeat themselves for several cycles and are associated with simple harmonic motion. These simple harmonic waves can be modeled using some combination of sine and cosine functions. For example, consider the simplified surface water wave that moves across the surface of water as illustrated in (Figure) . Unlike complex ocean waves, in surface water waves, the medium, in this case water, moves vertically, oscillating up and down, whereas the disturbance of the wave moves horizontally through the medium. In (Figure) , the waves causes a seagull to move up and down in simple harmonic motion as the wave crests and troughs (peaks and valleys) pass under the bird. The crest is the highest point of the wave, and the trough is the lowest part of the wave. The time for one complete oscillation of the up-and-down motion is the wave’s period T . The wave’s frequency is the number of waves that pass through a point per unit time and is equal to [latex] f=1\text{/}T. [/latex] The period can be expressed using any convenient unit of time but is usually measured in seconds; frequency is usually measured in hertz (Hz), where [latex] 1\,{\text{Hz}=1\,\text{s}}^{-1}. [/latex]
The length of the wave is called the wavelength and is represented by the Greek letter lambda [latex] (\lambda ) [/latex], which is measured in any convenient unit of length, such as a centimeter or meter. The wavelength can be measured between any two similar points along the medium that have the same height and the same slope. In (Figure) , the wavelength is shown measured between two crests. As stated above, the period of the wave is equal to the time for one oscillation, but it is also equal to the time for one wavelength to pass through a point along the wave’s path.
The amplitude of the wave ( A ) is a measure of the maximum displacement of the medium from its equilibrium position. In the figure, the equilibrium position is indicated by the dotted line, which is the height of the water if there were no waves moving through it. In this case, the wave is symmetrical, the crest of the wave is a distance [latex] \text{+}A [/latex] above the equilibrium position, and the trough is a distance [latex] \text{−}A [/latex] below the equilibrium position. The units for the amplitude can be centimeters or meters, or any convenient unit of distance.
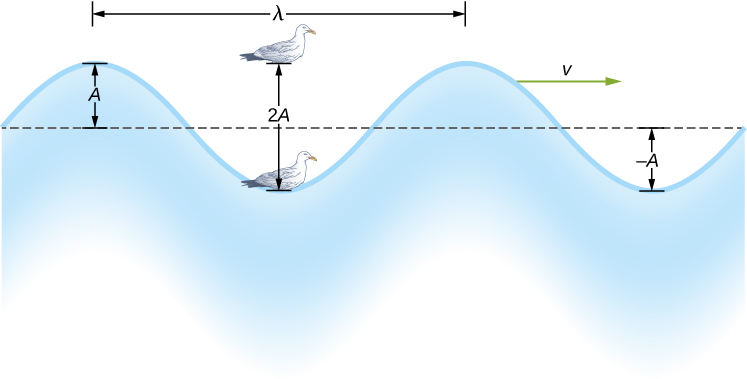
Figure 16.3 An idealized surface water wave passes under a seagull that bobs up and down in simple harmonic motion. The wave has a wavelength [latex] \lambda [/latex], which is the distance between adjacent identical parts of the wave. The amplitude A of the wave is the maximum displacement of the wave from the equilibrium position, which is indicated by the dotted line. In this example, the medium moves up and down, whereas the disturbance of the surface propagates parallel to the surface at a speed v.
The water wave in the figure moves through the medium with a propagation velocity [latex] \overset{\to }{v}. [/latex] The magnitude of the wave velocity is the distance the wave travels in a given time, which is one wavelength in the time of one period, and the wave speed is the magnitude of wave velocity. In equation form, this is
This fundamental relationship holds for all types of waves. For water waves, v is the speed of a surface wave; for sound, v is the speed of sound; and for visible light, v is the speed of light.
Transverse and Longitudinal Waves
We have seen that a simple mechanical wave consists of a periodic disturbance that propagates from one place to another through a medium. In (Figure) (a), the wave propagates in the horizontal direction, whereas the medium is disturbed in the vertical direction. Such a wave is called a transverse wave . In a transverse wave, the wave may propagate in any direction, but the disturbance of the medium is perpendicular to the direction of propagation. In contrast, in a longitudinal wave or compressional wave, the disturbance is parallel to the direction of propagation. (Figure) (b) shows an example of a longitudinal wave. The size of the disturbance is its amplitude A and is completely independent of the speed of propagation v .
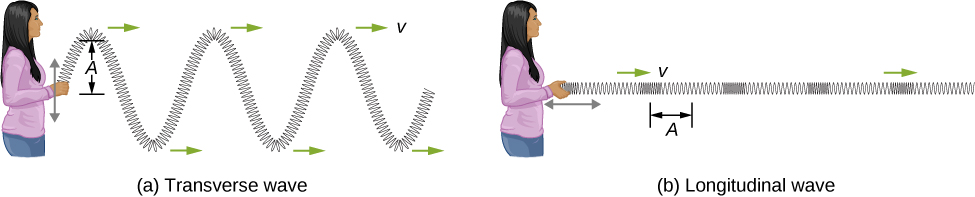
Figure 16.4 (a) In a transverse wave, the medium oscillates perpendicular to the wave velocity. Here, the spring moves vertically up and down, while the wave propagates horizontally to the right. (b) In a longitudinal wave, the medium oscillates parallel to the propagation of the wave. In this case, the spring oscillates back and forth, while the wave propagates to the right.
A simple graphical representation of a section of the spring shown in (Figure) (b) is shown in (Figure) . (Figure) (a) shows the equilibrium position of the spring before any waves move down it. A point on the spring is marked with a blue dot. (Figure) (b) through (g) show snapshots of the spring taken one-quarter of a period apart, sometime after the end of` the spring is oscillated back and forth in the x -direction at a constant frequency. The disturbance of the wave is seen as the compressions and the expansions of the spring. Note that the blue dot oscillates around its equilibrium position a distance A , as the longitudinal wave moves in the positive x -direction with a constant speed. The distance A is the amplitude of the wave. The y -position of the dot does not change as the wave moves through the spring. The wavelength of the wave is measured in part (d). The wavelength depends on the speed of the wave and the frequency of the driving force.
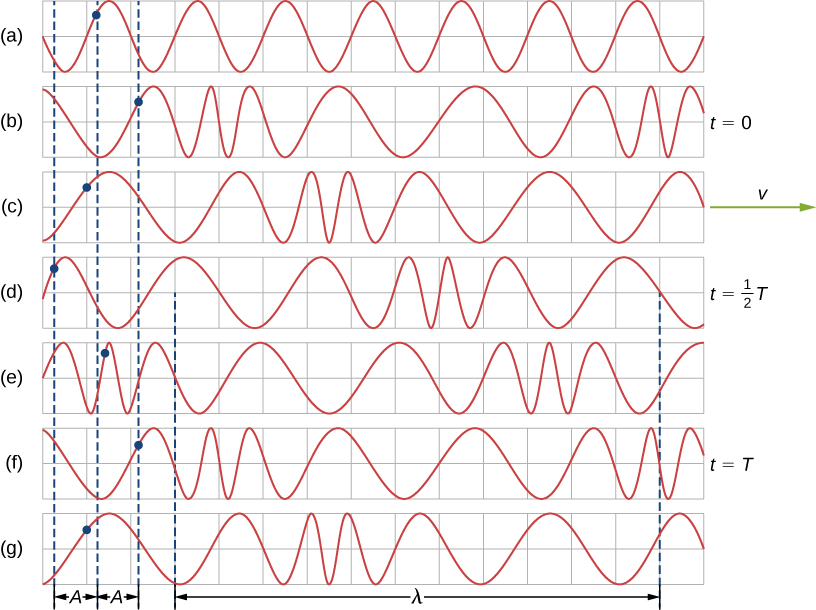
Figure 16.5 (a) This is a simple, graphical representation of a section of the stretched spring shown in (Figure)(b), representing the spring’s equilibrium position before any waves are induced on the spring. A point on the spring is marked by a blue dot. (b–g) Longitudinal waves are created by oscillating the end of the spring (not shown) back and forth along the x-axis. The longitudinal wave, with a wavelength [latex] \lambda [/latex], moves along the spring in the +x-direction with a wave speed v. For convenience, the wavelength is measured in (d). Note that the point on the spring that was marked with the blue dot moves back and forth a distance A from the equilibrium position, oscillating around the equilibrium position of the point.
Waves may be transverse, longitudinal, or a combination of the two. Examples of transverse waves are the waves on stringed instruments or surface waves on water, such as ripples moving on a pond. Sound waves in air and water are longitudinal. With sound waves, the disturbances are periodic variations in pressure that are transmitted in fluids. Fluids do not have appreciable shear strength, and for this reason, the sound waves in them are longitudinal waves. Sound in solids can have both longitudinal and transverse components, such as those in a seismic wave. Earthquakes generate seismic waves under Earth’s surface with both longitudinal and transverse components (called compressional or P-waves and shear or S-waves, respectively). The components of seismic waves have important individual characteristics—they propagate at different speeds, for example. Earthquakes also have surface waves that are similar to surface waves on water. Ocean waves also have both transverse and longitudinal components.
Wave on a String
A student takes a 30.00-m-long string and attaches one end to the wall in the physics lab. The student then holds the free end of the rope, keeping the tension constant in the rope. The student then begins to send waves down the string by moving the end of the string up and down with a frequency of 2.00 Hz. The maximum displacement of the end of the string is 20.00 cm. The first wave hits the lab wall 6.00 s after it was created. (a) What is the speed of the wave? (b) What is the period of the wave? (c) What is the wavelength of the wave?
- The speed of the wave can be derived by dividing the distance traveled by the time.
- The period of the wave is the inverse of the frequency of the driving force.
- The wavelength can be found from the speed and the period [latex] v=\lambda \text{/}T. [/latex]
- The first wave traveled 30.00 m in 6.00 s: [latex] v=\frac{30.00\,\text{m}}{6.00\,\text{s}}=5.00\frac{\text{m}}{\text{s}}. [/latex]
- The period is equal to the inverse of the frequency: [latex] T=\frac{1}{f}=\frac{1}{2.00\,{\text{s}}^{-1}}=0.50\,\text{s}. [/latex]
- The wavelength is equal to the velocity times the period: [latex] \lambda =vT=5.00\frac{\text{m}}{\text{s}}(0.50\,\text{s})=2.50\,\text{m}. [/latex]
Significance
The frequency of the wave produced by an oscillating driving force is equal to the frequency of the driving force.
Check Your Understanding
When a guitar string is plucked, the guitar string oscillates as a result of waves moving through the string. The vibrations of the string cause the air molecules to oscillate, forming sound waves. The frequency of the sound waves is equal to the frequency of the vibrating string. Is the wavelength of the sound wave always equal to the wavelength of the waves on the string?
The wavelength of the waves depends on the frequency and the velocity of the wave. The frequency of the sound wave is equal to the frequency of the wave on the string. The wavelengths of the sound waves and the waves on the string are equal only if the velocities of the waves are the same, which is not always the case. If the speed of the sound wave is different from the speed of the wave on the string, the wavelengths are different. This velocity of sound waves will be discussed in Sound .
Characteristics of a Wave
A transverse mechanical wave propagates in the positive x -direction through a spring (as shown in (Figure) (a)) with a constant wave speed, and the medium oscillates between [latex] \text{+}A [/latex] and [latex] \text{−}A [/latex] around an equilibrium position. The graph in (Figure) shows the height of the spring ( y ) versus the position ( x ), where the x -axis points in the direction of propagation. The figure shows the height of the spring versus the x -position at [latex] t=0.00\,\text{s} [/latex] as a dotted line and the wave at [latex] t=3.00\,\text{s} [/latex] as a solid line. (a) Determine the wavelength and amplitude of the wave. (b) Find the propagation velocity of the wave. (c) Calculate the period and frequency of the wave.
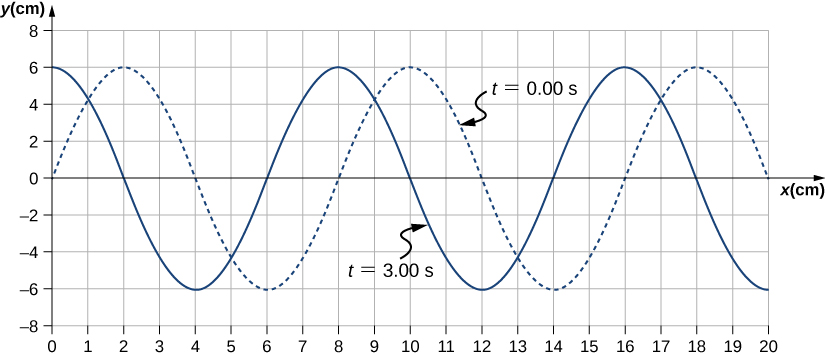
Figure 16.6 A transverse wave shown at two instants of time.
- The amplitude and wavelength can be determined from the graph.
- Since the velocity is constant, the velocity of the wave can be found by dividing the distance traveled by the wave by the time it took the wave to travel the distance.
- The period can be found from [latex] v=\frac{\lambda }{T} [/latex] and the frequency from [latex] f=\frac{1}{T}. [/latex]

Figure 16.7 Characteristics of the wave marked on a graph of its displacement.
- The distance the wave traveled from time [latex] t=0.00\,\text{s} [/latex] to time [latex] t=3.00\,\text{s} [/latex] can be seen in the graph. Consider the red arrow, which shows the distance the crest has moved in 3 s. The distance is [latex] 8.00\,\text{cm}-2.00\,\text{cm}=6.00\,\text{cm}. [/latex] The velocity is [latex] v=\frac{\text{Δ}x}{\text{Δ}t}=\frac{8.00\,\text{cm}-2.00\,\text{cm}}{3.00\,\text{s}-0.00\,\text{s}}=2.00\,\text{cm/s}. [/latex]
- The period is [latex] T=\frac{\lambda }{v}=\frac{8.00\,\text{cm}}{2.00\,\text{cm/s}}=4.00\,\text{s} [/latex] and the frequency is [latex] f=\frac{1}{T}=\frac{1}{4.00\,\text{s}}=0.25\,\text{Hz}. [/latex]
Note that the wavelength can be found using any two successive identical points that repeat, having the same height and slope. You should choose two points that are most convenient. The displacement can also be found using any convenient point.
The propagation velocity of a transverse or longitudinal mechanical wave may be constant as the wave disturbance moves through the medium. Consider a transverse mechanical wave: Is the velocity of the medium also constant?
- A wave is a disturbance that moves from the point of origin with a wave velocity v .
- A wave has a wavelength [latex] \lambda [/latex], which is the distance between adjacent identical parts of the wave. Wave velocity and wavelength are related to the wave’s frequency and period by [latex] v=\frac{\lambda }{T}=\lambda f. [/latex]
- Mechanical waves are disturbances that move through a medium and are governed by Newton’s laws.
- Electromagnetic waves are disturbances in the electric and magnetic fields, and do not require a medium.
- Matter waves are a central part of quantum mechanics and are associated with protons, electrons, neutrons, and other fundamental particles found in nature.
- A transverse wave has a disturbance perpendicular to the wave’s direction of propagation, whereas a longitudinal wave has a disturbance parallel to its direction of propagation.
Conceptual Questions
Give one example of a transverse wave and one example of a longitudinal wave, being careful to note the relative directions of the disturbance and wave propagation in each.
A sinusoidal transverse wave has a wavelength of 2.80 m. It takes 0.10 s for a portion of the string at a position x to move from a maximum position of [latex] y=0.03\,\text{m} [/latex] to the equilibrium position [latex] y=0. [/latex] What are the period, frequency, and wave speed of the wave?
What is the difference between propagation speed and the frequency of a mechanical wave? Does one or both affect wavelength? If so, how?
Propagation speed is the speed of the wave propagating through the medium. If the wave speed is constant, the speed can be found by [latex] v=\frac{\lambda }{T}=\lambda f. [/latex] The frequency is the number of wave that pass a point per unit time. The wavelength is directly proportional to the wave speed and inversely proportional to the frequency.
Consider a stretched spring, such as a slinky. The stretched spring can support longitudinal waves and transverse waves. How can you produce transverse waves on the spring? How can you produce longitudinal waves on the spring?
Consider a wave produced on a stretched spring by holding one end and shaking it up and down. Does the wavelength depend on the distance you move your hand up and down?
No, the distance you move your hand up and down will determine the amplitude of the wave. The wavelength will depend on the frequency you move your hand up and down, and the speed of the wave through the spring.
A sinusoidal, transverse wave is produced on a stretched spring, having a period T . Each section of the spring moves perpendicular to the direction of propagation of the wave, in simple harmonic motion with an amplitude A . Does each section oscillate with the same period as the wave or a different period? If the amplitude of the transverse wave were doubled but the period stays the same, would your answer be the same?
An electromagnetic wave, such as light, does not require a medium. Can you think of an example that would support this claim?
Storms in the South Pacific can create waves that travel all the way to the California coast, 12,000 km away. How long does it take them to travel this distance if they travel at 15.0 m/s?
Waves on a swimming pool propagate at 0.75 m/s. You splash the water at one end of the pool and observe the wave go to the opposite end, reflect, and return in 30.00 s. How far away is the other end of the pool?
[latex] 2d=vt⇒d=11.25\,\text{m} [/latex]
Wind gusts create ripples on the ocean that have a wavelength of 5.00 cm and propagate at 2.00 m/s. What is their frequency?
How many times a minute does a boat bob up and down on ocean waves that have a wavelength of 40.0 m and a propagation speed of 5.00 m/s?
Scouts at a camp shake the rope bridge they have just crossed and observe the wave crests to be 8.00 m apart. If they shake the bridge twice per second, what is the propagation speed of the waves?
What is the wavelength of the waves you create in a swimming pool if you splash your hand at a rate of 2.00 Hz and the waves propagate at a wave speed of 0.800 m/s?
[latex] v=f\lambda ⇒\lambda =0.400\,\text{m} [/latex]
What is the wavelength of an earthquake that shakes you with a frequency of 10.0 Hz and gets to another city 84.0 km away in 12.0 s?
Radio waves transmitted through empty space at the speed of light [latex] (v=c=3.00\,×\,{10}^{8}\,\text{m/s}) [/latex] by the Voyager spacecraft have a wavelength of 0.120 m. What is their frequency?
Your ear is capable of differentiating sounds that arrive at each ear just 0.34 ms apart, which is useful in determining where low frequency sound is originating from. (a) Suppose a low-frequency sound source is placed to the right of a person, whose ears are approximately 18 cm apart, and the speed of sound generated is 340 m/s. How long is the interval between when the sound arrives at the right ear and the sound arrives at the left ear? (b) Assume the same person was scuba diving and a low-frequency sound source was to the right of the scuba diver. How long is the interval between when the sound arrives at the right ear and the sound arrives at the left ear, if the speed of sound in water is 1500 m/s? (c) What is significant about the time interval of the two situations?
(a) Seismographs measure the arrival times of earthquakes with a precision of 0.100 s. To get the distance to the epicenter of the quake, geologists compare the arrival times of S- and P-waves, which travel at different speeds. If S- and P-waves travel at 4.00 and 7.20 km/s, respectively, in the region considered, how precisely can the distance to the source of the earthquake be determined? (b) Seismic waves from underground detonations of nuclear bombs can be used to locate the test site and detect violations of test bans. Discuss whether your answer to (a) implies a serious limit to such detection. (Note also that the uncertainty is greater if there is an uncertainty in the propagation speeds of the S- and P-waves.)
a. The P-waves outrun the S-waves by a speed of [latex] v=3.20\,\text{km/s;} [/latex] therefore, [latex] \text{Δ}d=0.320\,\text{km}. [/latex] b. Since the uncertainty in the distance is less than a kilometer, our answer to part (a) does not seem to limit the detection of nuclear bomb detonations. However, if the velocities are uncertain, then the uncertainty in the distance would increase and could then make it difficult to identify the source of the seismic waves.
A Girl Scout is taking a 10.00-km hike to earn a merit badge. While on the hike, she sees a cliff some distance away. She wishes to estimate the time required to walk to the cliff. She knows that the speed of sound is approximately 343 meters per second. She yells and finds that the echo returns after approximately 2.00 seconds. If she can hike 1.00 km in 10 minutes, how long would it take her to reach the cliff?
A quality assurance engineer at a frying pan company is asked to qualify a new line of nonstick-coated frying pans. The coating needs to be 1.00 mm thick. One method to test the thickness is for the engineer to pick a percentage of the pans manufactured, strip off the coating, and measure the thickness using a micrometer. This method is a destructive testing method. Instead, the engineer decides that every frying pan will be tested using a nondestructive method. An ultrasonic transducer is used that produces sound waves with a frequency of [latex] f=25\,\text{kHz}. [/latex] The sound waves are sent through the coating and are reflected by the interface between the coating and the metal pan, and the time is recorded. The wavelength of the ultrasonic waves in the coating is 0.076 m. What should be the time recorded if the coating is the correct thickness (1.00 mm)?
- OpenStax University Physics. Authored by : OpenStax CNX. Located at : https://cnx.org/contents/[email protected]:Gofkr9Oy@15 . License : CC BY: Attribution . License Terms : Download for free at http://cnx.org/contents/[email protected]
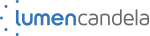
Privacy Policy
Forgot password? New user? Sign up
Existing user? Log in
Understanding Basic Traveling Waves
Already have an account? Log in here.
After learning the basics of periodic motion, it's time to take up the study of oscillations to the next level. By the next level, I mean the dependence of the motion of the considered particle on not just the time variable, but also on the distance variable. Let's dive right into the topic by first differentiating waves.
Types of Waves
What do we mean by travelling waves, equation representing a travelling wave.
We all have heard about various kinds of of waves traveling around us. Starting from the most familiar waves of light and sound, to the complex matter waves, all of them follow a common feature, i.e. oscillation of energy.
That is what a wave is.
A wave is just the phenomenon of oscillation of energy, using various properties of a medium such as physical, electro-magnetic, etc. For example, sound waves and light waves are both the carriers of energy, but a sound wave propagates through pressure variations, whereas a light wave travels by making use of electro-magnetic phenomena, which we'll discuss shortly.
Differentiating waves on alignment of propagation with respect to oscillation:
- Longitudinal waves: The type of waves that move in such a way that the oscillation of energy is along the direction of motion of the wave is defined as a longitudinal wave . Such kind of wave can be viewed by imagining two friends, who are walking forward, one ahead of the other, such that they continue tossing a ball between each other. In this, their motion can be seen as the motion of a wave and the ball acts as the packet of energy. These waves are also known as pressure waves .
- Transverse waves: The type of waves that move in such a way that the oscillation of energy is perpendicular to the direction of the motion of the wave is defined as transverse wave. Such kind of wave can be pictured by imagining the same two friends, walking forward, but one beside another, such that they continue tossing a ball between each other. In this, their motion can be seen as the motion of a wave and the ball acts as the packet of energy.
Imagine stretching a string and fixing both its ends on two points. Now grab the mid point of the string and pull it down, before letting it go. Chances are that you'll see the mid point of the string oscillating with an amplitude, with the end points fixed at their respective positions. These kind of waves are what we call the standing waves .
Now, for the next experiment, get into a hall with your friend and call out to him. If you shout loud enough and your friend hears well, chances are that he'll hear your call. Your voice reached him by the motion of sound waves, i.e. travelling waves . Had the sound waves been stationary, your voice would have never reached him.
We all have read about the basic mathematical equation that governs the trajectory or the position of a particle in SHM, or in other words, a particle belonging to a stationary wave. It is given by \(y(t)=a\sin \omega t \), where \(a\) represents the maximum displacement of the particle from the mean position ( amplitude ), and \(\omega\) represents the angular frequency for the SHM.
Now, since a travelling wave also moves forward while changing with time, a similar equation in case of a travelling wave must definitely include a function of both the direction of propagation (let it be \(z\)) and time. So, we get: \(y\left( z,t \right) =a\sin [z,t]\), where the symbols have their usual meanings.
To find this function that will explain the oscillation of the particle, we use the basic property of linearity of the function, i.e. the function enclosed inside the \(\sin\) block must be a linear function of \(z\) and \(t,\) or else the oscillating graph would lose its shape and the wave would compress or stretch out in different locations.
So, let that be given by \(\Phi \left( z,t \right)=\alpha z+\beta t\), but since we assume the wave to be travelling towards \(z=+\infty\), \(\alpha\) and \(\beta\) must have opposite signs. Therefore,
\[\Phi \left( z,t \right)=|\alpha |z-|\beta |t.\]
Also, we already know that this function must have the dimensions of radians , so \(\beta\) has the dimensions of \(T^{-1}\) and \(\alpha\) has the dimensions of \(L^{-1}\). As it turns out, \(\alpha\) is given by a constant \(k\) that is known as the wave-number for the wave, and equals \(\frac{2\pi}{\lambda}\), where \(\lambda\) is the wavelength of the wave. On the other hand, \(\beta\) is our age old friend \(\omega\) which equals \(2\pi\nu\), where \(\nu\) is the frequency.
So, we end up with: \(y\left( z,t \right) =a\sin ({k z-\omega t}). \)
Note: If the wave travels towards \(-\infty\), the function would change to \(y\left( z,t \right) =a\sin ({k z+\omega t}) .\)
Problem Loading...
Note Loading...
Set Loading...
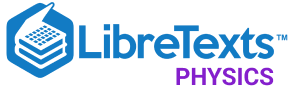
- school Campus Bookshelves
- menu_book Bookshelves
- perm_media Learning Objects
- login Login
- how_to_reg Request Instructor Account
- hub Instructor Commons
- Download Page (PDF)
- Download Full Book (PDF)
- Periodic Table
- Physics Constants
- Scientific Calculator
- Reference & Cite
- Tools expand_more
- Readability
selected template will load here
This action is not available.
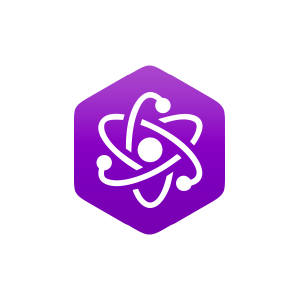
- Last updated
- Save as PDF
- Page ID 17096
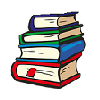
learning objectives
- Describe process of energy and mass transfer during wave motion
Vibrations and waves are extremely important phenomena in physics. In nature, oscillations are found everywhere. From the jiggling of atoms to the large oscillations of sea waves, we find examples of vibrations in almost every physical system. In physics a wave can be thought of as a disturbance or oscillation that travels through space-time, accompanied by a transfer of energy. Wave motion transfers energy from one point to another, often with no permanent displacement of the particles of the medium —that is, with little or no associated mass transport. They consist, instead, of oscillations or vibrations around almost fixed locations.
The emphasis of the last point highlights an important misconception of waves. Waves transfer energy not mass. An easy way to see this is to imagine a floating ball a few yards out to sea. As the waves propagate (i.e., travel) towards the shore, the ball will not come towards the shore. It may come to shore eventually due to the tides, current or wind, but the waves themselves will not carry the ball with them. A wave only moves mass perpendicular to the direction of propagation—in this case up and down, as illustrated in the figure below:
Wave motion : The point along the axis is analogous to the floating ball at sea. We notice that while it moves up and down it does not move in the direction of the wave’s propagation.
A wave can be transverse or longitudinal depending on the direction of its oscillation. Transverse waves occur when a disturbance causes oscillations perpendicular (at right angles) to the propagation (the direction of energy transfer). Longitudinal waves occur when the oscillations are parallel to the direction of propagation. While mechanical waves can be both transverse and longitudinal, all electromagnetic waves are transverse. Sound, for example, is a longitudinal wave.
The description of waves is closely related to their physical origin for each specific instance of a wave process. For example, acoustics is distinguished from optics in that sound waves are related to a mechanical rather than an electromagnetic (light) wave transfer caused by vibration. Therefore, concepts such as mass, momentum, inertia or elasticity become crucial in describing acoustic (as distinct from optic) wave processes. This difference in origin introduces certain wave characteristics particular to the properties of the medium involved. In this chapter we will closely examine the difference between longitudinal and transverse waves along with some of the properties they possess. We will also learn how waves are fundamental in describing motion of many applicable physical systems.
The Wave Equation : A brief introduction to the wave equation, discussing wave velocity, frequency, wavelength, and period.
Transverse Waves
Transverse waves propagate through media with a speed →vwv→w orthogonally to the direction of energy transfer.
- Describe properties of the transverse wave
A transverse wave is a moving wave that consists of oscillations occurring perpendicular (or right angled) to the direction of energy transfer. If a transverse wave is moving in the positive x -direction, its oscillations are in up and down directions that lie in the y–z plane. Light is an example of a transverse wave. For transverse waves in matter, the displacement of the medium is perpendicular to the direction of propagation of the wave. A ripple on a pond and a wave on a string are easily visualized transverse waves.
Transverse waves are waves that are oscillating perpendicularly to the direction of propagation. If you anchor one end of a ribbon or string and hold the other end in your hand, you can create transverse waves by moving your hand up and down. Notice though, that you can also launch waves by moving your hand side-to-side. This is an important point. There are two independent directions in which wave motion can occur. In this case, these are the y and z directions mentioned above. depicts the motion of a transverse wave. Here we observe that the wave is moving in t and oscillating in the x-y plane. A wave can be thought as comprising many particles (as seen in the figure) which oscillate up and down. In the figure we observe this motion to be in x-y plane (denoted by the red line in the figure). As time passes the oscillations are separated by units of time. The result of this separation is the sine curve we expect when we plot position versus time.
Sine Wave : The direction of propagation of this wave is along the t axis.
When a wave travels through a medium–i.e., air, water, etc., or the standard reference medium (vacuum)–it does so at a given speed: this is called the speed of propagation. The speed at which the wave propagates is denoted and can be found using the following formula:
\[\mathrm{v=fλ}\]
where v is the speed of the wave, f is the frequency , and is the wavelength. The wavelength spans crest to crest while the amplitude is 1/2 the total distance from crest to trough. Transverse waves have their applications in many areas of physics. Examples of transverse waves include seismic S (secondary) waves, and the motion of the electric (E) and magnetic (M) fields in an electromagnetic plane waves, which both oscillate perpendicularly to each other as well as to the direction of energy transfer. Therefore an electromagnetic wave consists of two transverse waves, visible light being an example of an electromagnetic wave.
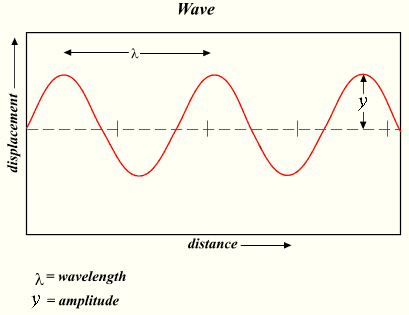
Wavelength and Amplitude : The wavelength is the distance between adjacent crests. The amplitude is the 1/2 the distance from crest to trough.
Two Types of Waves: Longitudinal vs. Transverse : Even ocean waves!
Longitudinal Waves
Longitudinal waves, sometimes called compression waves, oscillate in the direction of propagation.
- Give properties and provide examples of the longitudinal wave
Longitudinal waves have the same direction of vibration as their direction of travel. This means that the movement of the medium is in the same direction as the motion of the wave. Some longitudinal waves are also called compressional waves or compression waves. An easy experiment for observing longitudinal waves involves taking a Slinky and holding both ends. After compressing and releasing one end of the Slinky (while still holding onto the end), a pulse of more concentrated coils will travel to the end of the Slinky.
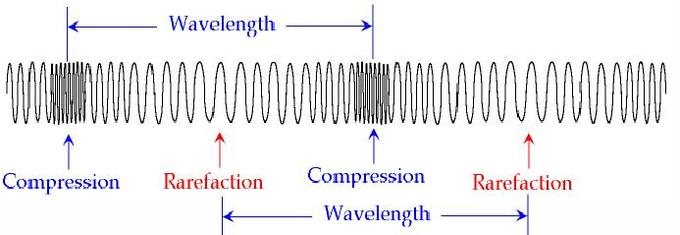
Longitudinal Waves : A compressed Slinky is an example of a longitudinal wave. The wve propagates in the same direction of oscillation.
Like transverse waves, longitudinal waves do not displace mass. The difference is that each particle which makes up the medium through which a longitudinal wave propagates oscillates along the axis of propagation. In the example of the Slinky, each coil will oscillate at a point but will not travel the length of the Slinky. It is important to remember that energy, in this case in the form of a pulse, is being transmitted and not the displaced mass.
Longitudinal waves can sometimes also be conceptualized as pressure waves. The most common pressure wave is the sound wave. Sound waves are created by the compression of a medium, usually air. Longitudinal sound waves are waves of alternating pressure deviations from the equilibrium pressure, causing local regions of compression and rarefaction. Matter in the medium is periodically displaced by a sound wave, and thus oscillates. When people make a sound, whether it is through speaking or hitting something, they are compressing the air particles to some significant amount. By doing so, they create transverse waves. When people hear sounds, their ears are sensitive to the pressure differences and interpret the waves as different tones.
Water Waves
Water waves can be commonly observed in daily life, and comprise both transverse and longitudinal wave motion.
- Describe particle movement in water waves
Water waves, which can be commonly observed in our daily lives, are of specific interest to physicists. Describing detailed fluid dynamics in water waves is beyond the scope of introductory physics courses. Although we often observe water wave propagating in 2D, in this atom we will limit our discussion to 1D propagation.
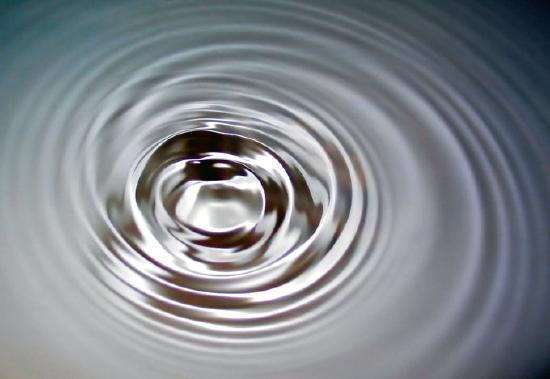
Water waves : Surface waves in water
The uniqueness of water waves is found in the observation that they comprise both transverse and longitudinal wave motion. As a result, the particles composing the wave move in clockwise circular motion, as seen in. Oscillatory motion is highest at the surface and diminishes exponentially with depth. Waves are generated by wind passing over the surface of the sea. As long as the waves propagate slower than the wind speed just above the waves, there is an energy transfer from the wind to the waves. Both air pressure differences between the upwind and the lee side of a wave crest, as well as friction on the water surface by the wind (making the water to go into the shear stress), contribute to the growth of the waves.
In the case of monochromatic linear plane waves in deep water, particles near the surface move in circular paths, creating a combination of longitudinal (back and forth) and transverse (up and down) wave motions. When waves propagate in shallow water (where the depth is less than half the wavelength ), the particle trajectories are compressed into ellipses. As the wave amplitude (height) increases, the particle paths no longer form closed orbits; rather, after the passage of each crest, particles are displaced slightly from their previous positions, a phenomenon known as Stokes drift.
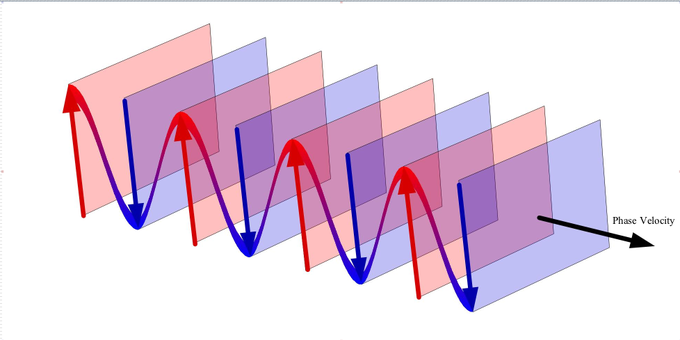
Plane wave : We see a wave propagating in the direction of the phase velocity. The wave can be thought to be made up of planes orthogonal to the direction of the phase velocity.
Since water waves transport energy, attempts to generate power from them have been made by utilizing the physical motion of such waves. Although larger waves are more powerful, wave power is also determined by wave speed, wavelength, and water density. Deep water corresponds with a water depth larger than half the wavelength, as is a common case in the sea and ocean. In deep water, longer-period waves propagate faster and transport their energy faster. The deep-water group velocity is half the phase velocity. In shallow water for wavelengths larger than about twenty times the water depth (as often found near the coast), the group velocity is equal to the phase velocity. These methods have proven viable in some cases but do not provide a fully sustainable form of renewable energy to date.
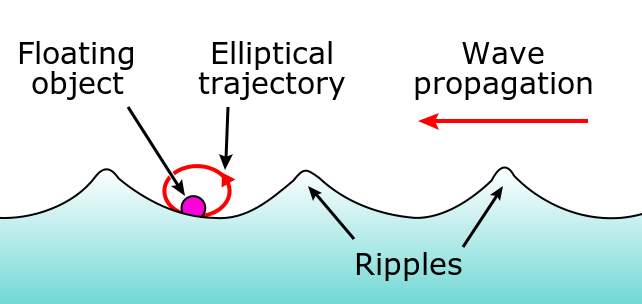
Water waves : The motion water waves causes particles to follow clockwise circular motion. This is a result of the wave having both transverse and longitudinal properties.
Wavelength, Freqency in Relation to Speed
Waves are defined by its frequency, wavelength, and amplitude among others. They also have two kinds of velocity: phase and group velocity.
- Identify major characteristic properties of waves
Characteristics of Waves
Waves have certain characteristic properties which are observable at first notice. The first property to note is the amplitude. The amplitude is half of the distance measured from crest to trough. We also observe the wavelength, which is the spatial period of the wave (e.g. from crest to crest or trough to trough). We denote the wavelength by the Greek letter λλ.
The frequency of a wave is the number of cycles per unit time — one can think of it as the number of crests which pass a fixed point per unit time. Mathematically, we make the observation that,
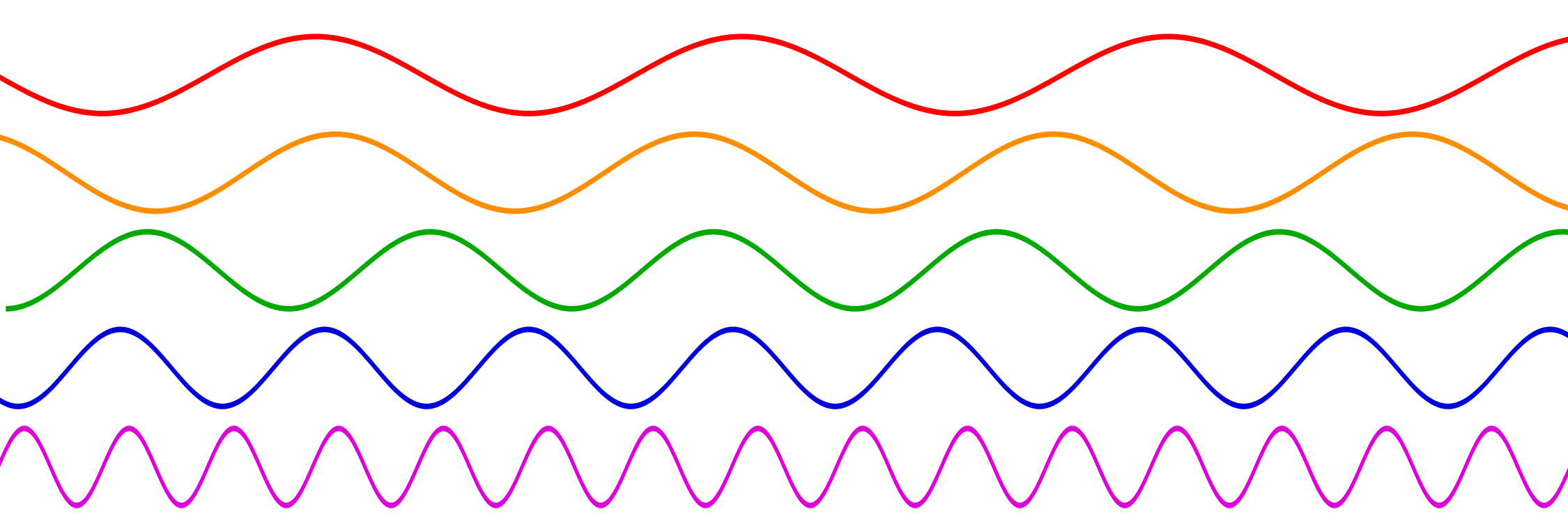
Frequencies of different sine waves. : The red wave has a low frequency sine there is very little repetition of cycles. Conversely we say that the purple wave has a high frequency. Note that time increases along the horizontal.
\[\mathrm{f=\dfrac{1}{T}}\]
where T is the period of oscillation. Frequency and wavelength can also be related-* with respects to a “speed” of a wave. In fact,
\[\mathrm{v=fλ}]
where v is called the wave speed, or more commonly,the phase velocity, the rate at which the phase of the wave propagates in space. This is the velocity at which the phase of any one frequency component of the wave travels. For such a component, any given phase of the wave (for example, the crest) will appear to travel at the phase velocity.
Finally, the group velocity of a wave is the velocity with which the overall shape of the waves’ amplitudes — known as the modulation or envelope of the wave — propagates through space. In, one may see that the overall shape (or “envelope”) propagates to the right, while the phase velocity is negative.
Fig 2 : This shows a wave with the group velocity and phase velocity going in different directions. (The group velocity is positive and the phase velocity is negative. )
Energy Transportation
Waves transfer energy which can be used to do work.
- Relate direction of energy and wave transportation
Energy transportion is essential to waves. It is a common misconception that waves move mass. Waves carry energy along an axis defined to be the direction of propagation. One easy example is to imagine that you are standing in the surf and you are hit by a significantly large wave, and once you are hit you are displaced (unless you hold firmly to your ground!). In this sense the wave has done work (it applied a force over a distance). Since work is done over time, the energy carried by a wave can be used to generate power.
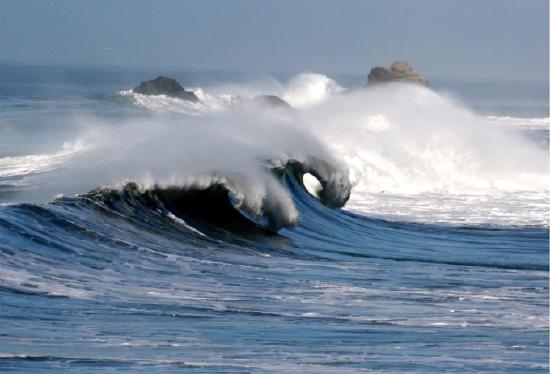
Water Wave : Waves that are more massive or have a greater velocity transport more energy.
Similarly we find that electromagnetic waves carry energy. Electromagnetic radiation (EMR) carries energy—sometimes called radiant energy—through space continuously away from the source (this is not true of the near-field part of the EM field). Electromagnetic waves can be imagined as a self-propagating transverse oscillating wave of electric and magnetic fields. EMR also carries both momentum and angular momentum. These properties may all be imparted to matter with which it interacts (through work). EMR is produced from other types of energy when created, and it is converted to other types of energy when it is destroyed. The photon is the quantum of the electromagnetic interaction, and is the basic “unit” or constituent of all forms of EMR. The quantum nature of light becomes more apparent at high frequencies (or high photon energy). Such photons behave more like particles than lower-frequency photons do.
Electromagnetic Wave : Electromagnetic waves can be imagined as a self-propagating transverse oscillating wave of electric and magnetic fields. This 3D diagram shows a plane linearly polarized wave propagating from left to right.
In general, there is a relation of waves which states that the velocity (\(\mathrm{v}\)) of a wave is proportional to the frequency (\(\mathrm{f}\)) times the wavelength (\(\mathrm{λ}\)):
We also know that classical momentum pp is given by p=mvp=mv which relates to force via Newton’s second law: \(\mathrm{F=\dfrac{dp}{dt}}\)
EM waves with higher frequencies carry more energy. This is a direct result of the equations above. Since \(\mathrm{v∝f}\) we find that higher frequencies imply greater velocity. If velocity is increased then we have greater momentum which implies a greater force (it gets a little bit tricky when we talk about particles moving close to the speed of light, but this observation holds in the classical sense). Since energy is the ability of an object to do work, we find that for \(\mathrm{W=Fd}\) a greater force correlates to more energy transfer. Again, this is an easy phenomenon to experience empirically; just stand in front of a faster wave and feel the difference!
- A wave can be thought of as a disturbance or oscillation that travels through space-time, accompanied by a transfer of energy.
- The direction a wave propagates is perpendicular to the direction it oscillates for transverse waves.
- A wave does not move mass in the direction of propagation; it transfers energy.
- Transverse waves oscillate in the z-y plane but travel along the x axis.
- A transverse wave has a speed of propagation given by the equation \(\mathrm{v = fλ}\).
- The direction of energy transfer is perpendicular to the motion of the wave.
- While longitudinal waves oscillate in the direction of propagation, they do not displace mass since the oscillations are small and involve an equilibrium position.
- The longitudinal ‘waves’ can be conceptualized as pulses that transfer energy along the axis of propagation.
- Longitudinal waves can be conceptualized as pressure waves characterized by compression and rarefaction.
- The particles which make up a water wave move in circular paths.
- If the waves move slower than the wind above them, energy is transfered from the wind to the waves.
- The oscillations are greatest on the surface of the wave and become weaker deeper in the fluid.
- The wavelength is the spatial period of the wave.
- The frequency of a wave refers to the number of cycles per unit time and is not to be confused with angular frequency.
- The phase velocity can be expressed as the product of wavelength and frequency.
- Waves which are more massive transfer more energy.
- Waves with greater velocities transfer more energy.
- Energy of a wave is transported in the direction of the waves transportation.
- medium : The material or empty space through which signals, waves or forces pass.
- direction of propagation : The axis along which the wave travels.
- wave : A moving disturbance in the energy level of a field.
- wavelength : The length of a single cycle of a wave, as measured by the distance between one peak or trough of a wave and the next; it is often designated in physics as λ, and corresponds to the velocity of the wave divided by its frequency.
- trough : A long, narrow depression between waves or ridges.
- speed of propagation : The speed at which a wave moves through a medium.
- crest : The ridge or top of a wave.
- transverse wave : Any wave in which the direction of disturbance is perpendicular to the direction of travel.
- rarefaction : a reduction in the density of a material, especially that of a fluid
- Longitudinal : Running in the direction of the long axis of a body.
- compression : to increase in density; the act of compressing, or the state of being compressed; compaction
- phase velocity : The velocity of propagation of a pure sine wave of infinite extent and infinitesimal amplitude.
- group velocity : The propagation velocity of the envelope of a modulated travelling wave, which is considered as the propagation velocity of information or energy contained in it.
- plane wave : A constant-frequency wave whose wavefronts (surfaces of constant phase) are infinite parallel planes of constant peak-to-peak amplitude normal to the phase velocity vector.
- wave speed : The absolute value of the velocity at which the phase of any one frequency component of the wave travels.
- frequency : The quotient of the number of times n a periodic phenomenon occurs over the time t in which it occurs: \(\mathrm{f = \frac{n}{t}}\).
- energy : A quantity that denotes the ability to do work and is measured in a unit dimensioned in mass × distance²/time² (ML²/T²) or the equivalent.
- power : A measure of the rate of doing work or transferring energy.
- work : A measure of energy expended in moving an object; most commonly, force times displacement. No work is done if the object does not move.
LICENSES AND ATTRIBUTIONS
CC LICENSED CONTENT, SHARED PREVIOUSLY
- Curation and Revision. Provided by : Boundless.com. License : CC BY-SA: Attribution-ShareAlike
CC LICENSED CONTENT, SPECIFIC ATTRIBUTION
- Waves. Provided by : Wikipedia. Located at : en.Wikipedia.org/wiki/Waves . License : CC BY-SA: Attribution-ShareAlike
- Wave. Provided by : Wikipedia. Located at : en.Wikipedia.org/wiki/Wave . License : CC BY-SA: Attribution-ShareAlike
- direction of propagation. Provided by : Wikipedia. Located at : en.Wikipedia.org/wiki/direction%20of%20propagation . License : CC BY-SA: Attribution-ShareAlike
- wave. Provided by : Wiktionary. Located at : en.wiktionary.org/wiki/wave . License : CC BY-SA: Attribution-ShareAlike
- medium. Provided by : Wiktionary. Located at : en.wiktionary.org/wiki/medium . License : CC BY-SA: Attribution-ShareAlike
- Simple harmonic motion animation. Provided by : Wikipedia. Located at : en.Wikipedia.org/wiki/File:Simple_harmonic_motion_animation.gif . License : Public Domain: No Known Copyright
- The Wave Equation. Located at : http://www.youtube.com/watch?v=jEEPp0mBCdg . License : Public Domain: No Known Copyright . License Terms : Standard YouTube license
- crest. Provided by : Wiktionary. Located at : en.wiktionary.org/wiki/crest . License : CC BY-SA: Attribution-ShareAlike
- Transverse wave. Provided by : Wikipedia. Located at : en.Wikipedia.org/wiki/Transverse_wave . License : CC BY-SA: Attribution-ShareAlike
- Boundless. Provided by : Boundless Learning. Located at : www.boundless.com//physics/definition/speed-of-propagation . License : CC BY-SA: Attribution-ShareAlike
- wavelength. Provided by : Wiktionary. Located at : en.wiktionary.org/wiki/wavelength . License : CC BY-SA: Attribution-ShareAlike
- trough. Provided by : Wiktionary. Located at : en.wiktionary.org/wiki/trough . License : CC BY-SA: Attribution-ShareAlike
- transverse wave. Provided by : Wiktionary. Located at : en.wiktionary.org/wiki/transverse_wave . License : CC BY-SA: Attribution-ShareAlike
- Wave. Provided by : Wikipedia. Located at : simple.Wikipedia.org/wiki/File:Wave.png . License : Public Domain: No Known Copyright
- Two Types of Waves: Longitudinal vs. Transverse. Located at : http://www.youtube.com/watch?v=PxB8-BVO82g . License : Public Domain: No Known Copyright . License Terms : Standard YouTube license
- ComplexSinInATimeAxe. Provided by : Wikipedia. Located at : en.Wikipedia.org/wiki/File:ComplexSinInATimeAxe.gif . License : Public Domain: No Known Copyright
- Longitudinal wave. Provided by : Wikipedia. Located at : en.Wikipedia.org/wiki/Longitudinal_wave . License : CC BY-SA: Attribution-ShareAlike
- Sound waves. Provided by : Wikipedia. Located at : en.Wikipedia.org/wiki/Sound_waves%23Longitudinal_and_transverse_waves . License : CC BY-SA: Attribution-ShareAlike
- Longitudinal. Provided by : Wiktionary. Located at : en.wiktionary.org/wiki/Longitudinal . License : CC BY-SA: Attribution-ShareAlike
- compression. Provided by : Wiktionary. Located at : en.wiktionary.org/wiki/compression . License : CC BY-SA: Attribution-ShareAlike
- rarefaction. Provided by : Wiktionary. Located at : en.wiktionary.org/wiki/rarefaction . License : CC BY-SA: Attribution-ShareAlike
- funwaves - 5.nLongitudinal wave. Provided by : Wikispaces. Located at : http://funwaves.wikispaces.com/5.+Longitudinal+wave . License : CC BY-SA: Attribution-ShareAlike
- Wave power. Provided by : Wikipedia. Located at : en.Wikipedia.org/wiki/Wave_power . License : CC BY-SA: Attribution-ShareAlike
- Wind wave. Provided by : Wikipedia. Located at : en.Wikipedia.org/wiki/Wind_wave . License : CC BY-SA: Attribution-ShareAlike
- Wave power. Provided by : Wikipedia. Located at : http://en.Wikipedia.org/wiki/Wave_power . License : CC BY-SA: Attribution-ShareAlike
- plane wave. Provided by : Wikipedia. Located at : en.Wikipedia.org/wiki/plane%20wave . License : CC BY-SA: Attribution-ShareAlike
- phase velocity. Provided by : Wiktionary. Located at : en.wiktionary.org/wiki/phase_velocity . License : CC BY-SA: Attribution-ShareAlike
- group velocity. Provided by : Wiktionary. Located at : en.wiktionary.org/wiki/group_velocity . License : CC BY-SA: Attribution-ShareAlike
- Wave. Provided by : Wikipedia. Located at : en.Wikipedia.org/wiki/Wave . License : CC BY: Attribution
- Plane Wave Oblique View. Provided by : Wikimedia. Located at : commons.wikimedia.org/wiki/File:Plane_Wave_Oblique_View.jpg . License : Public Domain: No Known Copyright
- Elliptical trajectory on ripples. Provided by : Wikipedia. Located at : en.Wikipedia.org/wiki/File:Elliptical_trajectory_on_ripples.svg . License : Public Domain: No Known Copyright
- Frequency. Provided by : Wikipedia. Located at : en.Wikipedia.org/wiki/Frequency . License : CC BY-SA: Attribution-ShareAlike
- Phase velocity. Provided by : Wikipedia. Located at : en.Wikipedia.org/wiki/Phase_velocity . License : CC BY-SA: Attribution-ShareAlike
- Group velocity. Provided by : Wikipedia. Located at : en.Wikipedia.org/wiki/Group_velocity . License : CC BY-SA: Attribution-ShareAlike
- wave speed. Provided by : Wikipedia. Located at : en.Wikipedia.org/wiki/wave%20speed . License : CC BY-SA: Attribution-ShareAlike
- frequency. Provided by : Wiktionary. Located at : en.wiktionary.org/wiki/frequency . License : CC BY-SA: Attribution-ShareAlike
- Group velocity. Provided by : Wikipedia. Located at : en.Wikipedia.org/wiki/Group_velocity . License : CC BY: Attribution
- Sine waves different frequencies. Provided by : Wikipedia. Located at : en.Wikipedia.org/wiki/File:Sine_waves_different_frequencies.svg . License : Public Domain: No Known Copyright
- Electromagnetic radiation. Provided by : Wikipedia. Located at : en.Wikipedia.org/wiki/Electromagnetic_radiation . License : CC BY-SA: Attribution-ShareAlike
- Electromagnetic radiation. Provided by : Wikipedia. Located at : http://en.Wikipedia.org/wiki/Electromagnetic_radiation . License : CC BY-SA: Attribution-ShareAlike
- energy. Provided by : Wiktionary. Located at : en.wiktionary.org/wiki/energy . License : CC BY-SA: Attribution-ShareAlike
- work. Provided by : Wiktionary. Located at : en.wiktionary.org/wiki/work . License : CC BY-SA: Attribution-ShareAlike
- Boundless. Provided by : Boundless Learning. Located at : www.boundless.com//sociology/definition/power . License : CC BY-SA: Attribution-ShareAlike
- Wave. Provided by : Wikipedia. Located at : http://simple.Wikipedia.org/wiki/File:Wave.png . License : Public Domain: No Known Copyright
- ComplexSinInATimeAxe. Provided by : Wikipedia. Located at : http://en.Wikipedia.org/wiki/File:ComplexSinInATimeAxe.gif . License : Public Domain: No Known Copyright
- Elliptical trajectory on ripples. Provided by : Wikipedia. Located at : http://en.Wikipedia.org/wiki/File:Elliptical_trajectory_on_ripples.svg . License : Public Domain: No Known Copyright
- Waves in pacifica 1. Provided by : Wikipedia. Located at : en.Wikipedia.org/wiki/File:Waves_in_pacifica_1.jpg . License : Public Domain: No Known Copyright
- Electromagneticwave3Dfromside. Provided by : Wikipedia. Located at : en.Wikipedia.org/wiki/File:Electromagneticwave3Dfromside.gif . License : CC BY: Attribution
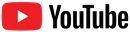
- TPC and eLearning
- Read Watch Interact
- What's NEW at TPC?
- Practice Review Test
- Teacher-Tools
- Subscription Selection
- Seat Calculator
- Ad Free Account
- Edit Profile Settings
- Classes (Version 2)
- Student Progress Edit
- Task Properties
- Export Student Progress
- Task, Activities, and Scores
- Metric Conversions Questions
- Metric System Questions
- Metric Estimation Questions
- Significant Digits Questions
- Proportional Reasoning
- Acceleration
- Distance-Displacement
- Dots and Graphs
- Graph That Motion
- Match That Graph
- Name That Motion
- Motion Diagrams
- Pos'n Time Graphs Numerical
- Pos'n Time Graphs Conceptual
- Up And Down - Questions
- Balanced vs. Unbalanced Forces
- Change of State
- Force and Motion
- Mass and Weight
- Match That Free-Body Diagram
- Net Force (and Acceleration) Ranking Tasks
- Newton's Second Law
- Normal Force Card Sort
- Recognizing Forces
- Air Resistance and Skydiving
- Solve It! with Newton's Second Law
- Which One Doesn't Belong?
- Component Addition Questions
- Head-to-Tail Vector Addition
- Projectile Mathematics
- Trajectory - Angle Launched Projectiles
- Trajectory - Horizontally Launched Projectiles
- Vector Addition
- Vector Direction
- Which One Doesn't Belong? Projectile Motion
- Forces in 2-Dimensions
- Being Impulsive About Momentum
- Explosions - Law Breakers
- Hit and Stick Collisions - Law Breakers
- Case Studies: Impulse and Force
- Impulse-Momentum Change Table
- Keeping Track of Momentum - Hit and Stick
- Keeping Track of Momentum - Hit and Bounce
- What's Up (and Down) with KE and PE?
- Energy Conservation Questions
- Energy Dissipation Questions
- Energy Ranking Tasks
- LOL Charts (a.k.a., Energy Bar Charts)
- Match That Bar Chart
- Words and Charts Questions
- Name That Energy
- Stepping Up with PE and KE Questions
- Case Studies - Circular Motion
- Circular Logic
- Forces and Free-Body Diagrams in Circular Motion
- Gravitational Field Strength
- Universal Gravitation
- Angular Position and Displacement
- Linear and Angular Velocity
- Angular Acceleration
- Rotational Inertia
- Balanced vs. Unbalanced Torques
- Getting a Handle on Torque
- Torque-ing About Rotation
- Properties of Matter
- Fluid Pressure
- Buoyant Force
- Sinking, Floating, and Hanging
- Pascal's Principle
- Flow Velocity
- Bernoulli's Principle
- Balloon Interactions
- Charge and Charging
- Charge Interactions
- Charging by Induction
- Conductors and Insulators
- Coulombs Law
- Electric Field
- Electric Field Intensity
- Polarization
- Case Studies: Electric Power
- Know Your Potential
- Light Bulb Anatomy
- I = ∆V/R Equations as a Guide to Thinking
- Parallel Circuits - ∆V = I•R Calculations
- Resistance Ranking Tasks
- Series Circuits - ∆V = I•R Calculations
- Series vs. Parallel Circuits
- Equivalent Resistance
- Period and Frequency of a Pendulum
- Pendulum Motion: Velocity and Force
- Energy of a Pendulum
- Period and Frequency of a Mass on a Spring
- Horizontal Springs: Velocity and Force
- Vertical Springs: Velocity and Force
- Energy of a Mass on a Spring
- Decibel Scale
- Frequency and Period
- Closed-End Air Columns
- Name That Harmonic: Strings
- Rocking the Boat
- Wave Basics
- Matching Pairs: Wave Characteristics
- Wave Interference
- Waves - Case Studies
- Color Addition and Subtraction
- Color Filters
- If This, Then That: Color Subtraction
- Light Intensity
- Color Pigments
- Converging Lenses
- Curved Mirror Images
- Law of Reflection
- Refraction and Lenses
- Total Internal Reflection
- Who Can See Who?
- Formulas and Atom Counting
- Atomic Models
- Bond Polarity
- Entropy Questions
- Cell Voltage Questions
- Heat of Formation Questions
- Reduction Potential Questions
- Oxidation States Questions
- Measuring the Quantity of Heat
- Hess's Law
- Oxidation-Reduction Questions
- Galvanic Cells Questions
- Thermal Stoichiometry
- Molecular Polarity
- Quantum Mechanics
- Balancing Chemical Equations
- Bronsted-Lowry Model of Acids and Bases
- Classification of Matter
- Collision Model of Reaction Rates
- Density Ranking Tasks
- Dissociation Reactions
- Complete Electron Configurations
- Elemental Measures
- Enthalpy Change Questions
- Equilibrium Concept
- Equilibrium Constant Expression
- Equilibrium Calculations - Questions
- Equilibrium ICE Table
- Intermolecular Forces Questions
- Ionic Bonding
- Lewis Electron Dot Structures
- Limiting Reactants
- Line Spectra Questions
- Mass Stoichiometry
- Measurement and Numbers
- Metals, Nonmetals, and Metalloids
- Metric Estimations
- Metric System
- Molarity Ranking Tasks
- Mole Conversions
- Name That Element
- Names to Formulas
- Names to Formulas 2
- Nuclear Decay
- Particles, Words, and Formulas
- Periodic Trends
- Precipitation Reactions and Net Ionic Equations
- Pressure Concepts
- Pressure-Temperature Gas Law
- Pressure-Volume Gas Law
- Chemical Reaction Types
- Significant Digits and Measurement
- States Of Matter Exercise
- Stoichiometry Law Breakers
- Stoichiometry - Math Relationships
- Subatomic Particles
- Spontaneity and Driving Forces
- Gibbs Free Energy
- Volume-Temperature Gas Law
- Acid-Base Properties
- Energy and Chemical Reactions
- Chemical and Physical Properties
- Valence Shell Electron Pair Repulsion Theory
- Writing Balanced Chemical Equations
- Mission CG1
- Mission CG10
- Mission CG2
- Mission CG3
- Mission CG4
- Mission CG5
- Mission CG6
- Mission CG7
- Mission CG8
- Mission CG9
- Mission EC1
- Mission EC10
- Mission EC11
- Mission EC12
- Mission EC2
- Mission EC3
- Mission EC4
- Mission EC5
- Mission EC6
- Mission EC7
- Mission EC8
- Mission EC9
- Mission RL1
- Mission RL2
- Mission RL3
- Mission RL4
- Mission RL5
- Mission RL6
- Mission KG7
- Mission RL8
- Mission KG9
- Mission RL10
- Mission RL11
- Mission RM1
- Mission RM2
- Mission RM3
- Mission RM4
- Mission RM5
- Mission RM6
- Mission RM8
- Mission RM10
- Mission LC1
- Mission RM11
- Mission LC2
- Mission LC3
- Mission LC4
- Mission LC5
- Mission LC6
- Mission LC8
- Mission SM1
- Mission SM2
- Mission SM3
- Mission SM4
- Mission SM5
- Mission SM6
- Mission SM8
- Mission SM10
- Mission KG10
- Mission SM11
- Mission KG2
- Mission KG3
- Mission KG4
- Mission KG5
- Mission KG6
- Mission KG8
- Mission KG11
- Mission F2D1
- Mission F2D2
- Mission F2D3
- Mission F2D4
- Mission F2D5
- Mission F2D6
- Mission KC1
- Mission KC2
- Mission KC3
- Mission KC4
- Mission KC5
- Mission KC6
- Mission KC7
- Mission KC8
- Mission AAA
- Mission SM9
- Mission LC7
- Mission LC9
- Mission NL1
- Mission NL2
- Mission NL3
- Mission NL4
- Mission NL5
- Mission NL6
- Mission NL7
- Mission NL8
- Mission NL9
- Mission NL10
- Mission NL11
- Mission NL12
- Mission MC1
- Mission MC10
- Mission MC2
- Mission MC3
- Mission MC4
- Mission MC5
- Mission MC6
- Mission MC7
- Mission MC8
- Mission MC9
- Mission RM7
- Mission RM9
- Mission RL7
- Mission RL9
- Mission SM7
- Mission SE1
- Mission SE10
- Mission SE11
- Mission SE12
- Mission SE2
- Mission SE3
- Mission SE4
- Mission SE5
- Mission SE6
- Mission SE7
- Mission SE8
- Mission SE9
- Mission VP1
- Mission VP10
- Mission VP2
- Mission VP3
- Mission VP4
- Mission VP5
- Mission VP6
- Mission VP7
- Mission VP8
- Mission VP9
- Mission WM1
- Mission WM2
- Mission WM3
- Mission WM4
- Mission WM5
- Mission WM6
- Mission WM7
- Mission WM8
- Mission WE1
- Mission WE10
- Mission WE2
- Mission WE3
- Mission WE4
- Mission WE5
- Mission WE6
- Mission WE7
- Mission WE8
- Mission WE9
- Vector Walk Interactive
- Name That Motion Interactive
- Kinematic Graphing 1 Concept Checker
- Kinematic Graphing 2 Concept Checker
- Graph That Motion Interactive
- Two Stage Rocket Interactive
- Rocket Sled Concept Checker
- Force Concept Checker
- Free-Body Diagrams Concept Checker
- Free-Body Diagrams The Sequel Concept Checker
- Skydiving Concept Checker
- Elevator Ride Concept Checker
- Vector Addition Concept Checker
- Vector Walk in Two Dimensions Interactive
- Name That Vector Interactive
- River Boat Simulator Concept Checker
- Projectile Simulator 2 Concept Checker
- Projectile Simulator 3 Concept Checker
- Hit the Target Interactive
- Turd the Target 1 Interactive
- Turd the Target 2 Interactive
- Balance It Interactive
- Go For The Gold Interactive
- Egg Drop Concept Checker
- Fish Catch Concept Checker
- Exploding Carts Concept Checker
- Collision Carts - Inelastic Collisions Concept Checker
- Its All Uphill Concept Checker
- Stopping Distance Concept Checker
- Chart That Motion Interactive
- Roller Coaster Model Concept Checker
- Uniform Circular Motion Concept Checker
- Horizontal Circle Simulation Concept Checker
- Vertical Circle Simulation Concept Checker
- Race Track Concept Checker
- Gravitational Fields Concept Checker
- Orbital Motion Concept Checker
- Angular Acceleration Concept Checker
- Balance Beam Concept Checker
- Torque Balancer Concept Checker
- Aluminum Can Polarization Concept Checker
- Charging Concept Checker
- Name That Charge Simulation
- Coulomb's Law Concept Checker
- Electric Field Lines Concept Checker
- Put the Charge in the Goal Concept Checker
- Circuit Builder Concept Checker (Series Circuits)
- Circuit Builder Concept Checker (Parallel Circuits)
- Circuit Builder Concept Checker (∆V-I-R)
- Circuit Builder Concept Checker (Voltage Drop)
- Equivalent Resistance Interactive
- Pendulum Motion Simulation Concept Checker
- Mass on a Spring Simulation Concept Checker
- Particle Wave Simulation Concept Checker
- Boundary Behavior Simulation Concept Checker
- Slinky Wave Simulator Concept Checker
- Simple Wave Simulator Concept Checker
- Wave Addition Simulation Concept Checker
- Standing Wave Maker Simulation Concept Checker
- Color Addition Concept Checker
- Painting With CMY Concept Checker
- Stage Lighting Concept Checker
- Filtering Away Concept Checker
- InterferencePatterns Concept Checker
- Young's Experiment Interactive
- Plane Mirror Images Interactive
- Who Can See Who Concept Checker
- Optics Bench (Mirrors) Concept Checker
- Name That Image (Mirrors) Interactive
- Refraction Concept Checker
- Total Internal Reflection Concept Checker
- Optics Bench (Lenses) Concept Checker
- Kinematics Preview
- Velocity Time Graphs Preview
- Moving Cart on an Inclined Plane Preview
- Stopping Distance Preview
- Cart, Bricks, and Bands Preview
- Fan Cart Study Preview
- Friction Preview
- Coffee Filter Lab Preview
- Friction, Speed, and Stopping Distance Preview
- Up and Down Preview
- Projectile Range Preview
- Ballistics Preview
- Juggling Preview
- Marshmallow Launcher Preview
- Air Bag Safety Preview
- Colliding Carts Preview
- Collisions Preview
- Engineering Safer Helmets Preview
- Push the Plow Preview
- Its All Uphill Preview
- Energy on an Incline Preview
- Modeling Roller Coasters Preview
- Hot Wheels Stopping Distance Preview
- Ball Bat Collision Preview
- Energy in Fields Preview
- Weightlessness Training Preview
- Roller Coaster Loops Preview
- Universal Gravitation Preview
- Keplers Laws Preview
- Kepler's Third Law Preview
- Charge Interactions Preview
- Sticky Tape Experiments Preview
- Wire Gauge Preview
- Voltage, Current, and Resistance Preview
- Light Bulb Resistance Preview
- Series and Parallel Circuits Preview
- Thermal Equilibrium Preview
- Linear Expansion Preview
- Heating Curves Preview
- Electricity and Magnetism - Part 1 Preview
- Electricity and Magnetism - Part 2 Preview
- Vibrating Mass on a Spring Preview
- Period of a Pendulum Preview
- Wave Speed Preview
- Slinky-Experiments Preview
- Standing Waves in a Rope Preview
- Sound as a Pressure Wave Preview
- DeciBel Scale Preview
- DeciBels, Phons, and Sones Preview
- Sound of Music Preview
- Shedding Light on Light Bulbs Preview
- Models of Light Preview
- Electromagnetic Radiation Preview
- Electromagnetic Spectrum Preview
- EM Wave Communication Preview
- Digitized Data Preview
- Light Intensity Preview
- Concave Mirrors Preview
- Object Image Relations Preview
- Snells Law Preview
- Reflection vs. Transmission Preview
- Magnification Lab Preview
- Reactivity Preview
- Ions and the Periodic Table Preview
- Periodic Trends Preview
- Intermolecular Forces Preview
- Melting Points and Boiling Points Preview
- Reaction Rates Preview
- Ammonia Factory Preview
- Stoichiometry Preview
- Gaining Teacher Access
- Tasks and Classes
- Tasks - Classic
- Subscription
- Subscription Locator
- 1-D Kinematics
- Newton's Laws
- Vectors - Motion and Forces in Two Dimensions
- Momentum and Its Conservation
- Work and Energy
- Circular Motion and Satellite Motion
- Thermal Physics
- Static Electricity
- Electric Circuits
- Vibrations and Waves
- Sound Waves and Music
- Light and Color
- Reflection and Mirrors
- About the Physics Interactives
- Task Tracker
- Usage Policy
- Newtons Laws
- Vectors and Projectiles
- Forces in 2D
- Momentum and Collisions
- Circular and Satellite Motion
- Balance and Rotation
- Electromagnetism
- Waves and Sound
- Forces in Two Dimensions
- Work, Energy, and Power
- Circular Motion and Gravitation
- Sound Waves
- 1-Dimensional Kinematics
- Circular, Satellite, and Rotational Motion
- Einstein's Theory of Special Relativity
- Waves, Sound and Light
- QuickTime Movies
- About the Concept Builders
- Pricing For Schools
- Directions for Version 2
- Measurement and Units
- Relationships and Graphs
- Rotation and Balance
- Vibrational Motion
- Reflection and Refraction
- Teacher Accounts
- Task Tracker Directions
- Kinematic Concepts
- Kinematic Graphing
- Wave Motion
- Sound and Music
- About CalcPad
- 1D Kinematics
- Vectors and Forces in 2D
- Simple Harmonic Motion
- Rotational Kinematics
- Rotation and Torque
- Rotational Dynamics
- Electric Fields, Potential, and Capacitance
- Transient RC Circuits
- Light Waves
- Units and Measurement
- Stoichiometry
- Molarity and Solutions
- Thermal Chemistry
- Acids and Bases
- Kinetics and Equilibrium
- Solution Equilibria
- Oxidation-Reduction
- Nuclear Chemistry
- NGSS Alignments
- 1D-Kinematics
- Projectiles
- Circular Motion
- Magnetism and Electromagnetism
- Graphing Practice
- About the ACT
- ACT Preparation
- For Teachers
- Other Resources
- Newton's Laws of Motion
- Work and Energy Packet
- Static Electricity Review
- Solutions Guide
- Solutions Guide Digital Download
- Motion in One Dimension
- Work, Energy and Power
- Frequently Asked Questions
- Purchasing the Download
- Purchasing the CD
- Purchasing the Digital Download
- About the NGSS Corner
- NGSS Search
- Force and Motion DCIs - High School
- Energy DCIs - High School
- Wave Applications DCIs - High School
- Force and Motion PEs - High School
- Energy PEs - High School
- Wave Applications PEs - High School
- Crosscutting Concepts
- The Practices
- Physics Topics
- NGSS Corner: Activity List
- NGSS Corner: Infographics
- About the Toolkits
- Position-Velocity-Acceleration
- Position-Time Graphs
- Velocity-Time Graphs
- Newton's First Law
- Newton's Second Law
- Newton's Third Law
- Terminal Velocity
- Projectile Motion
- Forces in 2 Dimensions
- Impulse and Momentum Change
- Momentum Conservation
- Work-Energy Fundamentals
- Work-Energy Relationship
- Roller Coaster Physics
- Satellite Motion
- Electric Fields
- Circuit Concepts
- Series Circuits
- Parallel Circuits
- Describing-Waves
- Wave Behavior Toolkit
- Standing Wave Patterns
- Resonating Air Columns
- Wave Model of Light
- Plane Mirrors
- Curved Mirrors
- Teacher Guide
- Using Lab Notebooks
- Current Electricity
- Light Waves and Color
- Reflection and Ray Model of Light
- Refraction and Ray Model of Light
- Classes (Legacy Version)
- Teacher Resources
- Subscriptions

- Newton's Laws
- Einstein's Theory of Special Relativity
- About Concept Checkers
- School Pricing
- Newton's Laws of Motion
- Newton's First Law
- Newton's Third Law
- Traveling Waves vs. Standing Waves
- Formation of Standing Waves
- Nodes and Anti-nodes
- Harmonics and Patterns
- Mathematics of Standing Waves
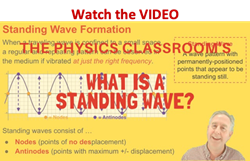
What is a Standing Wave Pattern?
It is however possible to have a wave confined to a given space in a medium and still produce a regular wave pattern that is readily discernible amidst the motion of the medium. For instance, if an elastic rope is held end-to-end and vibrated at just the right frequency , a wave pattern would be produced that assumes the shape of a sine wave and is seen to change over time. The wave pattern is only produced when one end of the rope is vibrated at just the right frequency. When the proper frequency is used, the interference of the incident wave and the reflected wave occur in such a manner that there are specific points along the medium that appear to be standing still. Because the observed wave pattern is characterized by points that appear to be standing still, the pattern is often called a standing wave pattern . There are other points along the medium whose displacement changes over time, but in a regular manner. These points vibrate back and forth from a positive displacement to a negative displacement; the vibrations occur at regular time intervals such that the motion of the medium is regular and repeating. A pattern is readily observable.
We Would Like to Suggest ...
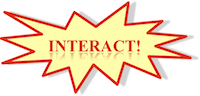
Travelling Wave
When something about the physical world changes, the information about that disturbance gradually moves outwards, away from the source, in every direction. As the information travels, it travels in the form of a wave. Sound to our ears, light to our eyes, and electromagnetic radiation to our mobile phones are all transported in the form of waves. A good visual example of the propagation of waves is the waves created on the surface of the water when a stone is dropped into a lake. In this article, we will be learning more about travelling waves.
Describing a Wave
A wave can be described as a disturbance in a medium that travels transferring momentum and energy without any net motion of the medium. A wave in which the positions of maximum and minimum amplitude travel through the medium is known as a travelling wave. To better understand a wave, let us think of the disturbance caused when we jump on a trampoline. When we jump on a trampoline, the downward push that we create at a point on the trampoline slightly moves the material next to it downward too.
When the created disturbance travels outward, the point at which our feet first hit the trampoline recovers moving outward because of the tension force in the trampoline and that moves the surrounding nearby materials outward too. This up and down motion gradually ripples out as it covers more area of the trampoline. And, this disturbance takes the shape of a wave.
Following are a few important points to remember about the wave:
- The high points in the wave are known as crests and the low points in the wave are known as troughs.
- The maximum distance of the disturbance of the wave from the mid-point to either the top of the crest or to the bottom of a trough is known as amplitude.
- The distance between two adjacent crests or two adjacent troughs is known as a wavelength and is denoted by 𝛌.
- The time interval of one complete vibration is known as a time period.
- The number of vibrations the wave undergoes in one second is known as a frequency.
- The relationship between the time period and frequency is given as follows:
- The speed of a wave is given by the equation
Different Types of Waves
Different types of waves exhibit distinct characteristics. These characteristics help us distinguish between wave types. The orientation of particle motion relative to the direction of wave propagation is one way the traveling waves are distinguished. Following are the different types of waves categorized based on the particle motion:
- Pulse Waves – A pulse wave is a wave comprising only one disturbance or only one crest that travels through the transmission medium.
- Continuous Waves – A continuous-wave is a waveform of constant amplitude and frequency.
- Transverse Waves – In a transverse wave, the motion of the particle is perpendicular to the direction of propagation of the wave.
- Longitudinal Waves – Longitudinal waves are the waves in which the motion of the particle is in the same direction as the propagation of the wave.
Although they are different, there is one property common between them and that is the transportation of energy. An object in simple harmonic motion has an energy of
Constructive and Destructive Interference
A phenomenon in which two waves superimpose to form a resultant wave of lower, greater, or the same amplitude is known as interference. Constructive and destructive interference occurs due to the interaction of waves that are correlated with each other either because of the same frequency or because they come from the same source. The interference effects can be observed in all types of waves such as gravity waves and light waves.
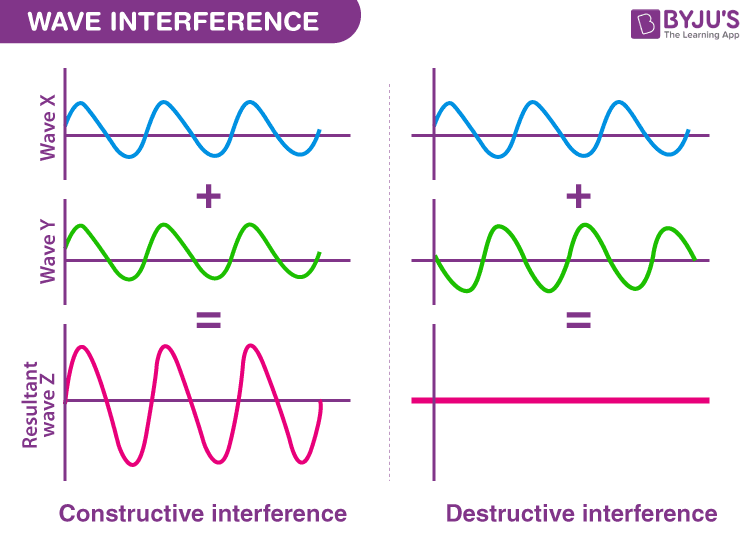
According to the principle of superposition of the waves , when two or more propagating waves of the same type are incidents on the same point, the resultant amplitude is equal to the vector sum of the amplitudes of the individual waves. When a crest of a wave meets a crest of another wave of the same frequency at the same point, then the resultant amplitude is the sum of the individual amplitudes. This type of interference is known as constructive interference. If a crest of a wave meets a trough of another wave, then the resulting amplitude is equal to the difference in the individual amplitudes and this is known as destructive interference.
Stay tuned to BYJU’S to learn more physics concepts with the help of interactive videos.
Watch the video and understand longitudinal and transverse waves in detail.
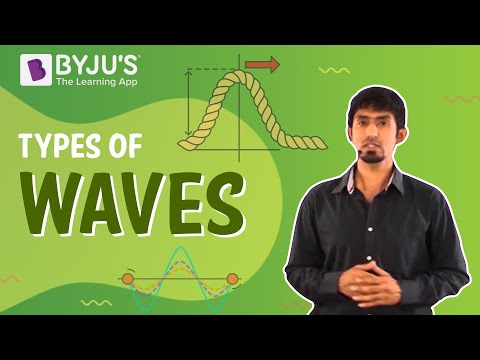
Frequently Asked Questions – FAQs
What is a pulse wave, what are longitudinal waves, what is superposition of waves, what is electromagnetic radiation, what is constructive interference.
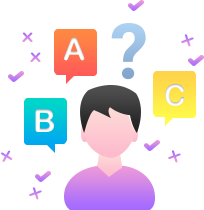
Put your understanding of this concept to test by answering a few MCQs. Click ‘Start Quiz’ to begin!
Select the correct answer and click on the “Finish” button Check your score and answers at the end of the quiz
Visit BYJU’S for all Physics related queries and study materials
Your result is as below
Request OTP on Voice Call
Leave a Comment Cancel reply
Your Mobile number and Email id will not be published. Required fields are marked *
Post My Comment

- Share Share
Register with BYJU'S & Download Free PDFs
Register with byju's & watch live videos.

If you're seeing this message, it means we're having trouble loading external resources on our website.
If you're behind a web filter, please make sure that the domains *.kastatic.org and *.kasandbox.org are unblocked.
To log in and use all the features of Khan Academy, please enable JavaScript in your browser.
Oscillations and waves (Essentials) - Class 11th
Course: oscillations and waves (essentials) - class 11th > unit 3.
- Introduction to waves
- Identifying transverse and longitudinal waves
Transverse and longitudinal waves review
How to identify types of waves, common mistakes and misconceptions, want to join the conversation.
- Upvote Button navigates to signup page
- Downvote Button navigates to signup page
- Flag Button navigates to signup page

13.1 Types of Waves
Section learning objectives.
By the end of this section, you will be able to do the following:
- Define mechanical waves and medium, and relate the two
- Distinguish a pulse wave from a periodic wave
- Distinguish a longitudinal wave from a transverse wave and give examples of such waves
Teacher Support
The learning objectives in this section will help your students master the following standards:
- (A) examine and describe oscillatory motion and wave propagation in various types of media.
Section Key Terms
Mechanical waves.
What do we mean when we say something is a wave? A wave is a disturbance that travels or propagates from the place where it was created. Waves transfer energy from one place to another, but they do not necessarily transfer any mass. Light, sound, and waves in the ocean are common examples of waves. Sound and water waves are mechanical waves ; meaning, they require a medium to travel through. The medium may be a solid, a liquid, or a gas, and the speed of the wave depends on the material properties of the medium through which it is traveling. However, light is not a mechanical wave; it can travel through a vacuum such as the empty parts of outer space.
A familiar wave that you can easily imagine is the water wave. For water waves, the disturbance is in the surface of the water, an example of which is the disturbance created by a rock thrown into a pond or by a swimmer splashing the water surface repeatedly. For sound waves, the disturbance is caused by a change in air pressure, an example of which is when the oscillating cone inside a speaker creates a disturbance. For earthquakes, there are several types of disturbances, which include the disturbance of Earth’s surface itself and the pressure disturbances under the surface. Even radio waves are most easily understood using an analogy with water waves. Because water waves are common and visible, visualizing water waves may help you in studying other types of waves, especially those that are not visible.
Water waves have characteristics common to all waves, such as amplitude , period , frequency , and energy , which we will discuss in the next section.
Misconception Alert
Many people think that water waves push water from one direction to another. In reality, however, the particles of water tend to stay in one location only, except for moving up and down due to the energy in the wave. The energy moves forward through the water, but the water particles stay in one place. If you feel yourself being pushed in an ocean, what you feel is the energy of the wave, not the rush of water. If you put a cork in water that has waves, you will see that the water mostly moves it up and down.
[BL] [OL] [AL] Ask students to give examples of mechanical and nonmechanical waves.
Pulse Waves and Periodic Waves
If you drop a pebble into the water, only a few waves may be generated before the disturbance dies down, whereas in a wave pool, the waves are continuous. A pulse wave is a sudden disturbance in which only one wave or a few waves are generated, such as in the example of the pebble. Thunder and explosions also create pulse waves. A periodic wave repeats the same oscillation for several cycles, such as in the case of the wave pool, and is associated with simple harmonic motion. Each particle in the medium experiences simple harmonic motion in periodic waves by moving back and forth periodically through the same positions.
[BL] Any kind of wave, whether mechanical or nonmechanical, or transverse or longitudinal, can be in the form of a pulse wave or a periodic wave.
Consider the simplified water wave in Figure 13.2 . This wave is an up-and-down disturbance of the water surface, characterized by a sine wave pattern. The uppermost position is called the crest and the lowest is the trough . It causes a seagull to move up and down in simple harmonic motion as the wave crests and troughs pass under the bird.
Longitudinal Waves and Transverse Waves
Mechanical waves are categorized by their type of motion and fall into any of two categories: transverse or longitudinal. Note that both transverse and longitudinal waves can be periodic. A transverse wave propagates so that the disturbance is perpendicular to the direction of propagation. An example of a transverse wave is shown in Figure 13.3 , where a woman moves a toy spring up and down, generating waves that propagate away from herself in the horizontal direction while disturbing the toy spring in the vertical direction.
In contrast, in a longitudinal wave , the disturbance is parallel to the direction of propagation. Figure 13.4 shows an example of a longitudinal wave, where the woman now creates a disturbance in the horizontal direction—which is the same direction as the wave propagation—by stretching and then compressing the toy spring.
Tips For Success
Longitudinal waves are sometimes called compression waves or compressional waves , and transverse waves are sometimes called shear waves .
Teacher Demonstration
Transverse and longitudinal waves may be demonstrated in the class using a spring or a toy spring, as shown in the figures.
Waves may be transverse, longitudinal, or a combination of the two . The waves on the strings of musical instruments are transverse (as shown in Figure 13.5 ), and so are electromagnetic waves, such as visible light. Sound waves in air and water are longitudinal. Their disturbances are periodic variations in pressure that are transmitted in fluids.
Sound in solids can be both longitudinal and transverse. Essentially, water waves are also a combination of transverse and longitudinal components, although the simplified water wave illustrated in Figure 13.2 does not show the longitudinal motion of the bird.
Earthquake waves under Earth’s surface have both longitudinal and transverse components as well. The longitudinal waves in an earthquake are called pressure or P-waves, and the transverse waves are called shear or S-waves. These components have important individual characteristics; for example, they propagate at different speeds. Earthquakes also have surface waves that are similar to surface waves on water.
Energy propagates differently in transverse and longitudinal waves. It is important to know the type of the wave in which energy is propagating to understand how it may affect the materials around it.
Watch Physics
Introduction to waves.
This video explains wave propagation in terms of momentum using an example of a wave moving along a rope. It also covers the differences between transverse and longitudinal waves, and between pulse and periodic waves.
- After a compression wave, some molecules move forward temporarily.
- After a compression wave, some molecules move backward temporarily.
- After a compression wave, some molecules move upward temporarily.
- After a compression wave, some molecules move downward temporarily.
Fun In Physics
The physics of surfing.
Many people enjoy surfing in the ocean. For some surfers, the bigger the wave, the better. In one area off the coast of central California, waves can reach heights of up to 50 feet in certain times of the year ( Figure 13.6 ).
How do waves reach such extreme heights? Other than unusual causes, such as when earthquakes produce tsunami waves, most huge waves are caused simply by interactions between the wind and the surface of the water. The wind pushes up against the surface of the water and transfers energy to the water in the process. The stronger the wind, the more energy transferred. As waves start to form, a larger surface area becomes in contact with the wind, and even more energy is transferred from the wind to the water, thus creating higher waves. Intense storms create the fastest winds, kicking up massive waves that travel out from the origin of the storm. Longer-lasting storms and those storms that affect a larger area of the ocean create the biggest waves since they transfer more energy. The cycle of the tides from the Moon’s gravitational pull also plays a small role in creating waves.
Actual ocean waves are more complicated than the idealized model of the simple transverse wave with a perfect sinusoidal shape. Ocean waves are examples of orbital progressive waves , where water particles at the surface follow a circular path from the crest to the trough of the passing wave, then cycle back again to their original position. This cycle repeats with each passing wave.
As waves reach shore, the water depth decreases and the energy of the wave is compressed into a smaller volume. This creates higher waves—an effect known as shoaling .
Since the water particles along the surface move from the crest to the trough, surfers hitch a ride on the cascading water, gliding along the surface. If ocean waves work exactly like the idealized transverse waves, surfing would be much less exciting as it would simply involve standing on a board that bobs up and down in place, just like the seagull in the previous figure.
Additional information and illustrations about the scientific principles behind surfing can be found in the “Using Science to Surf Better!” video.
- The surfer would move side-to-side/back-and-forth vertically with no horizontal motion.
- The surfer would forward and backward horizontally with no vertical motion.
Check Your Understanding
Use these questions to assess students’ achievement of the section’s Learning Objectives. If students are struggling with a specific objective, these questions will help identify such objective and direct them to the relevant content.
- A wave is a force that propagates from the place where it was created.
- A wave is a disturbance that propagates from the place where it was created.
- A wave is matter that provides volume to an object.
- A wave is matter that provides mass to an object.
- No, electromagnetic waves do not require any medium to propagate.
- No, mechanical waves do not require any medium to propagate.
- Yes, both mechanical and electromagnetic waves require a medium to propagate.
- Yes, all transverse waves require a medium to travel.
- A pulse wave is a sudden disturbance with only one wave generated.
- A pulse wave is a sudden disturbance with only one or a few waves generated.
- A pulse wave is a gradual disturbance with only one or a few waves generated.
- A pulse wave is a gradual disturbance with only one wave generated.
What are the categories of mechanical waves based on the type of motion?
- Both transverse and longitudinal waves
- Only longitudinal waves
- Only transverse waves
- Only surface waves
In which direction do the particles of the medium oscillate in a transverse wave?
- Perpendicular to the direction of propagation of the transverse wave
- Parallel to the direction of propagation of the transverse wave
As an Amazon Associate we earn from qualifying purchases.
This book may not be used in the training of large language models or otherwise be ingested into large language models or generative AI offerings without OpenStax's permission.
Want to cite, share, or modify this book? This book uses the Creative Commons Attribution License and you must attribute Texas Education Agency (TEA). The original material is available at: https://www.texasgateway.org/book/tea-physics . Changes were made to the original material, including updates to art, structure, and other content updates.
Access for free at https://openstax.org/books/physics/pages/1-introduction
- Authors: Paul Peter Urone, Roger Hinrichs
- Publisher/website: OpenStax
- Book title: Physics
- Publication date: Mar 26, 2020
- Location: Houston, Texas
- Book URL: https://openstax.org/books/physics/pages/1-introduction
- Section URL: https://openstax.org/books/physics/pages/13-1-types-of-waves
© Jan 19, 2024 Texas Education Agency (TEA). The OpenStax name, OpenStax logo, OpenStax book covers, OpenStax CNX name, and OpenStax CNX logo are not subject to the Creative Commons license and may not be reproduced without the prior and express written consent of Rice University.

An official website of the United States government
The .gov means it’s official. Federal government websites often end in .gov or .mil. Before sharing sensitive information, make sure you’re on a federal government site.
The site is secure. The https:// ensures that you are connecting to the official website and that any information you provide is encrypted and transmitted securely.
- Publications
- Account settings
Preview improvements coming to the PMC website in October 2024. Learn More or Try it out now .
- Advanced Search
- Journal List
- HHS Author Manuscripts

Cortical travelling waves: mechanisms and computational principles
Lyle muller.
1 Salk Institute for Biological Studies, La Jolla, CA, USA
Frédéric Chavane
2 Institut de Neurosciences de la Timone (INT), Centre National de la Recherche Scientifique (CNRS) and Aix-Marseille Université, Marseille, France
John Reynolds
Terrence j. sejnowski.
3 Division of Biological Sciences, University of California, La Jolla, CA, USA
Multichannel recording technologies have revealed travelling waves of neural activity in multiple sensory, motor and cognitive systems. These waves can be spontaneously generated by recurrent circuits or evoked by external stimuli. They travel along brain networks at multiple scales, transiently modulating spiking and excitability as they pass. Here, we review recent experimental findings that have found evidence for travelling waves at single-area (mesoscopic) and whole-brain (macroscopic) scales. We place these findings in the context of the current theoretical understanding of wave generation and propagation in recurrent networks. During the large low-frequency rhythms of sleep or the relatively desynchronized state of the awake cortex, travelling waves may serve a variety of functions, from long-term memory consolidation to processing of dynamic visual stimuli. We explore new avenues for experimental and computational understanding of the role of spatiotemporal activity patterns in the cortex.
Synchrony in the activity of neuronal populations has long been of interest in neuroscience 1 , 2 , but its function has remained elusive. Neural oscillations are rhythmic fluctuations of spiking activity within neuronal populations. It is proposed that synchrony of neural oscillations in different neural populations might be able to shape input gain and aid information transfer between these populations 3 , 4 because the synchronous occurrence of action potentials in many neurons is known to increase their ability to drive spikes in shared target neurons 5 , 6 . Indeed, experimental evidence suggests that oscillation synchrony modulates the effective connectivity of populations in the cortex 7 , 8 , shapes plasticity between neurons 9 and drives processing of stimulus features between visual cortex areas V1 and V4 ( REF. 10 ).
These proposed functions have traditionally been thought to involve precise zero-lag synchrony 11 , 12 , in which oscillations across single areas or distant regions in the cortex are aligned perfectly in time. However, with the advent of simultaneous multichannel recording technologies, we have begun to appreciate that the relative timing of neural population activity is constantly changing. Instead of precise zero-lag synchrony, a range of flexible phase offsets is possible. These flexible phase relationships can, in their simplest form, be travelling waves of various shapes (including plane, radial and spiral waves). The combination of multiple travelling waves can form complex spatiotemporal patterns.
Stimulus-driven and internally generated travelling waves occur across different brain states and sensory conditions. Brain state and synaptic activity strongly shape these waves so that they differ profoundly from sleep 13 to wake 14 . In turn, these waves transiently modulate neuronal excitability 15 – 19 and shape responses to external input 20 over a wide range of spatial and temporal scales. They travel over spatial scales that range from the mesoscopic (single cortical areas and millimetres of cortex) to the macroscopic (global patterns of activity over several centimetres) and extend over temporal scales from tens to hundreds of milliseconds. Although, in initial studies, mesoscopic travelling waves were pronounced only under conditions of low visual luminance contrast 21 , 22 and were mostly reported in anaesthetized states 23 – 28 , recent work has shown that stimulus-evoked mesoscopic travelling waves robustly appear in the awake visual cortex 14 , 29 and can influence intracortical dynamics during the viewing of natural stimuli 30 .
In vitro and in vivo cortical travelling waves have been reviewed previously 22 , 31 , 32 . In this article, we cover a series of recent studies that have led to important advances in our understanding of travelling waves and the generality of their role in cortical processing. We first summarize experimental observations of travelling waves in the cortex, focusing on advances in recording and analysis techniques that have enabled the detection of waves in single cortical regions. Next, we briefly review the broad computational and mathematical literature on travelling waves in neural networks, focusing on theoretical mechanisms for wave generation and possible experimental tests of these predictions. Finally, we discuss emerging computational roles for cortical travelling waves, from sensory processing to memory consolidation. As we describe, at the macroscopic scale, these waves allow the formation of the large-scale, distributed cell assemblies thought to underlie long-term memory 33 , 34 . At the mesoscopic scale, multiple lines of experimental evidence suggest that evoked waves are a way for neural circuits to flexibly store and use information from the recent past. In closing, we highlight open questions for new experiments and theoretical work to further elucidate the biological mechanisms underlying cortical travelling waves and their role in neural computation.
Travelling waves in neural systems
Travelling waves were first observed at the macroscopic, whole-brain scale. More recently, new high-resolution optical and electrophysiological recording technologies have led to the observation of travelling waves at the mesoscopic scale of single regions in the cortex. Distinct axonal fibre tracts carry waves at the two scales, and there are observable differences in the resulting dynamics — most notably, spatial extent and propagation speed — that make clear distinctions between the two possible. Macroscopic waves are generally recorded using electroencephalography (EEG) or electrocorticography (ECoG), in which arrays of electrodes either outside the skull (EEG) or directly on the cortical surface (ECoG) record potentials from neural populations with high temporal (millisecond) resolution but low spatial (centimetre) resolution. These waves generally have propagation speeds from 1 to 10 metres per second, falling within the range of the axonal conduction speeds of myelinated white matter fibres in the cortex 35 . Mesoscopic waves are generally observed in the local field potential (LFP) using multielectrode arrays (MEAs) or in optical imaging signals recorded with voltage-sensitive dyes (VSDs). These techniques record from single regions in the cortex with high temporal and spatial resolution 36 , 37 . Stimulus-evoked mesoscopic waves can appear in the sensory cortex during the fast onset transient that follows the presentation of a stimulus; these waves are thus time-locked to the stimulus. Alternatively, they may appear during sustained responses in a manner that is not necessarily locked to stimulus onset (often called induced waves). Mesoscopic waves generally have propagation speeds from 0.1 to 0.8 metres per second, consistent with the axonal conduction speed of the unmyelinated long-range horizontal fibres within the superficial layers of the cortex 38 .
Macroscopic travelling waves
Neuroscientists first observed oscillations in EEG through the work of clinicians in the late nineteenth and early twentieth centuries 39 . In 1929, Hans Berger reported the 10 Hz alpha oscillation in EEG recordings of human occipital lobes 40 . Shortly thereafter, there was much interest in understanding the spatial organization of alpha oscillations through multielectrode recordings, which often appeared to show a wave travelling from occipital to frontal regions 41 . Alpha-band travelling waves in EEG recordings under both spontaneous and stimulus-driven conditions continue to be a subject of active interest 42 , 43 . In subsequent studies, it also has been reported that the 0.1-1.0 Hz slow oscillation that occurs during sleep is an anterior-to-posterior travelling wave 44 ( FIG. 1a ) and that auditory-induced 30–80 Hz gamma oscillations make a coherent anterior-to-posterior sweep across the cortex (observed using magnetic field tomography) 45 . Signal distortion by cranial tissues 46 and volume conduction, however, pose substantial possible confounds for detection of travelling waves in EEG. Understanding the generation of electric and magnetic fields by neural activity remains an active pursuit for experimental and theoretical research 46 , 47 ; however, in the absence of a general physical theory 48 , 49 , the origin and interpretation of travelling waves observed solely in EEG is problematic (see REF. 50 for an initial comparison).

a | The slow oscillation of deep non-rapid-eye-movement (non-REM) sleep has been reported to be a travelling wave that moves globally from anterior to posterior regions 44 . The upper left panel represents the time course of one slow oscillation averaged across electroencephalogram (EEG) channels. The lower left panels illustrate the evolution of voltages across the scalp at different times during the slow wave. At the start of the slow oscillation, negative EEG potentials begin in anterior sections of the scalp, and as the oscillation progresses, these travel to posterior regions. The negative peak of the slow oscillation is delayed in posterior regions by hundreds of milliseconds, reflecting the propagation time of the travelling wave (right panel). b | Sleep ‘spindles’ are 11–15 Hz oscillations that occur during stage 2 non-REM sleep and have long been known to be important for learning and memory. These spindles systematically travel as global rotating waves from the temporal to the parietal to the frontal cortex (and are therefore called TPF waves) in intracranial electrocorticography (ECoG) recordings 13 . The panels illustrate the normalized spindle amplitude at each ECoG electrode (small circles) at successive points in the spindle cycle. From the start of an oscillation cycle (+0 ms), spindle amplitudes peak successively in the temporal (+0 ms), parietal (+20 ms) and frontal (+40 ms) lobes. The amplitudes recorded for each channel are normalized to their maximum amplitude. Part a is republished with permission of the Society of Neuroscience, from The sleep slow oscillation as a traveling wave. Massimini, M et al. J. Neurosci. 24 (31), 6872–6870 (2004); permission conveyed through Copyright Clearance Center, Inc. ( REF. 44 ). Part b is adapted with permission from REF 13 , eLife.
In recent years, intracranial ECoG recordings in clinical patients have made detailed analyses of human cortical dynamics possible. Following a technique developed by Penfield and Jasper for mapping the cortical sites involved in generating epileptic seizures 51 , surgeons typically implant two-dimensional ECoG electrode arrays on the surface of one cortical hemisphere, with coverage of temporal, parietal and frontal lobes supplemented by additional linear strips of electrodes on the same or the contralateral hemisphere. ECoG recordings have a high signal-to-noise ratio and spatial localization, reflecting neural activity spanning less than 1 centimetre of the cortex 52 , 53 . Furthermore, by capturing power in the 80-150 Hz high-gamma band, which serves as an index for neuronal firing near the electrode 54 , ECoG recordings can link oscillatory patterns with local population spiking activity.
Analysis of ECoG recordings has revealed travelling waves during both awake and sleep states. The spatial range of these oscillatory patterns can vary from relatively small patches of cortex to the global, whole-brain scale. In the awake state, travelling alpha waves in ECoG recordings have a dorsoventral direction of propagation that is markedly different from waves observed in EEG recordings 55 . Similarly, the sleep slow oscillation 56 and the related K-complex 57 have complicated and variable patterns of propagation in ECoG recordings, in contrast to the smooth anterior-to-posterior propagation observed in EEG. Thus, at the higher spatial resolution afforded by intracranial recordings, oscillatory activity generally has different and more complicated dynamical patterns than those observed with EEG. However, ECoG recordings have shown that sleep spindles in humans exhibit robust spatiotemporal travelling waves that either radiate out from a point source or rotate across the cortex in a preferred direction, travelling from the temporal cortex to the parietal cortex, on to the frontal lobe and back again to the temporal cortex 13 ( FIG. 1b ). These rotating waves represent a large proportion (50.8%) of all spindle cycles, with radial waves constituting a smaller fraction (15.6%) and the other cycles likely reflecting only local activity (33.6%) 58 , 59 . The observed wavelengths of these waves are similar to the size of the brain, blurring out any effects of the folds of cortical sulci and gyri; however, with higher-resolution imaging techniques, a more detailed impact of the cortical folds may become apparent. The speeds at which these waves travel are concentrated between 2 and 5 metres per second, consistent with the conduction speed of short-range 38 and long-range 35 , 60 association fibres in the cortical white matter. These association fibres thus provide a possible substrate for propagation of ECoG travelling waves, suggesting that the waves have a functional role in building distributed cell assemblies in the cortex 13 .
Mesoscopic travelling waves
Advances in multichannel recording technologies now allow single-trial recordings in individual cortical areas with very high spatial (up to 20 micrometres) and temporal (up to 1 millisecond) resolution. For the first time, the responses of distributed neuronal populations in the cortex to sensory stimuli can be monitored through both optical and electrophysiological approaches. These new recordings can give important clues about the neural code and the role of topographic maps in sensory systems. Furthermore, the analysis of single trials gives new insights into sources of variability in cortical responses to repeated presentations of the same sensory stimulus.
To understand results from these recordings in single regions, an important distinction must be made between two theoretical models of the cortical sensory response: stationary bumps of neural activity and cortical travelling waves 14 . A stationary bump of neural activity would remain centred on the point of strongest input to the cortex, perhaps increasing in amplitude and expanding in range over time ( FIG. 2 ). In V1, the point of strongest input is the retinotopic representation in the cortex of the stimulus in the visual field. Here, spikes carried by thalamocortical fibres enter layer 4, which then projects to layer 2/3 of V1. Thus, the spatiotemporal pattern of activation of a stationary bump is mainly driven by the input and shaped to a lesser degree by intracortical axons 61 – 63 . In a stimulus-evoked cortical travelling wave, on the other hand, the cells at the point of strongest input would emit a peak of activity that expands progressively across the retinotopic map ( FIG. 2 ). This pattern would indicate the presence of dominant recurrent interactions in the cortex, which are largely attributed to propagation along intracortical axons 64 , 65 . This distinction leads to quite different hypothesized downstream effects: in a cortical area receiving input from V1, cells would receive their strongest input at any point in time either from the central point (stationary bump) or from a progressively expanding ring of activation (cortical travelling wave) 14 . We expand on the computational consequences of each model for the sensory response below.

a | Small visual stimuli evoke spikes in a set of thalamocortical fibres targeting layer 4 of the primary visual cortex, which, in turn, synapses onto a small segment of layer 2/3 (the feedforward-driven zone). Recurrent horizontal fibres synapse widely across layer 2/3, spanning up to 8 millimetres of distance in the cortex (response zone) 107 . b | Within the response zone, the activity pattern is proposed to take two main forms: a stationary bump of activity, suggesting a relatively input-driven system, or a travelling wave, suggesting a circuit dominated by recurrent activity 14 . Schematic diagrams indicate these hypothesized activity patterns and are based on results reported in REF. 14 .
Whether the stimulus-evoked response in the awake sensory cortex is a stationary bump or a travelling wave is debated. Initial evidence that visual stimuli evoke travelling waves came from optical imaging during sustained responses in semi-isolated turtle cortex 66 . This was followed by intracellular recordings in V1 of anaesthetized cats, in which it was shown that the visually evoked response became progressively more delayed with increasing distance from the receptive field of the neuron 67 ( FIG. 3a ), with the relationship between the distance and delay being consistent with the axonal conduction speeds of the unmyelinated horizontal fibres in layer 2/3 (0.1–0.8 metres per second 38 ). By contrast, the initial VSD studies in V1 of awake behaving monkeys found a stationary bump of activation in the evoked response 68 ( FIG. 3b ). These results, however, required averaging over many trials to increase the signal-to-noise ratio, which may have masked travelling waves. The first VSD studies able to image cortical dynamics at the single-trial level were in V1 of anaesthetized rodents 69 , 70 . These studies found an active travelling wave propagating out from the stimulus location in the cortex. A later study used a spike- triggered averaging (STA) approach in multielectrode array recordings to reveal spontaneous and stimulus-evoked waves in V1 of anaesthetized cats and macaques 21 . The validity of these findings, however, was later called into question because STA is open to artefacts caused by low-pass filtering 71 (see REF 72 for a rebuttal) or by not fully accounting for the point process statistics of spike trains 73 . Furthermore, in another VSD study performed in V1 of awake monkeys, again at the level of trial- averaged data, no delay across cortical space (2.5 millimetres) in the initial rise of the neural response was found, suggesting that the delays of the response peak are explained by intracortical gain- control mechanisms rather than by horizontal-fibre travelling waves 74 .

a | These intracellular recordings of a complex cell in the cat visual cortex area V1 during anaesthesia show responses to an optimally oriented bar stimulus 67 . The middle row is the response to stimulation in the centre of the receptive field of the cell. Response deflections become weaker and more delayed as the stimulus is presented farther away in visual space (top and bottom traces). This delay suggests that individual small visual stimuli evoke waves travelling across the retinotopic map of visual space. b | The first voltage-sensitive dye (VSD) studies in area V1 of an awake monkey exhibited only stationary bump responses to small visual stimuli, questioning the existence of travelling waves in V1 during waking states 68 . These plots show time from stimulus onset (above) and the VSD response in V1 and V2 (coloured in intensity from yellow to red to white). c | Single-trial analysis of VSD data in V1 of an awake monkey demonstrated that the stimulus onset transient (which occurs 40-140 ms after the start of stimulus presentation) is indeed a travelling wave, which is masked in trial-averaged data. Plotted are the average amplitudes of the VSD response in regions of interest located at increasing distances from the point of afferent input (indicated by colours from blue to red, as shown in inset box). The dark blue region contains the afferent input zone. Evoked amplitudes show increasing temporal offset in both the initial (40-60 ms) and peak (80-100 ms) response. Grey dots represent interpolated maxima illustrating the temporal offset in the peak response. Part a is adapted with permission from REF. 67 , AAAS. Part b is adapted with permission from Slovin, H. et al. Long-term voltage-sensitive dye imaging reveals cortical dynamics in behaving monkeys. J. Neurophysiol. 88, 3421–3438 (2002) ( REF 68 ). Part c is adapted from REF 14 , Macmillan Publishers Limited.
New methods for the analysis of single-trial recordings have clarified this issue. A general phase-based algorithm was introduced that can detect arbitrarily shaped waves in high-noise multichannel data 14 . Applied to single- trial VSD recordings in awake behaving monkeys, this approach revealed that a small, high-contrast visual stimulus consistently evoked a single-cycle wave travelling across several millimetres of V1 ( FIG. 3c ), which was previously obscured by trial averaging. The isolated waves occurred during the fast stimulus onset response and exhibited consistent delays in initial rise times across the cortex ( FIG. 3c ), providing evidence against the gain-control mechanism previously suggested 74 . Furthermore, the waves were shown to possess an intrinsic reliability: although wave onset was variable across trials, their cross-trial variability did not increase as they travelled across the cortex, indicating a propagation substrate with a highly consistent speed. These shifts in wave onset time relative to stimulus onset strongly contribute to attenuation of the waves in trial-averaged data. Together with the measured propagation speeds and shift in initial rises, this result implicates the horizontal fibres in layer 2/3 as the physical substrate for a wave of neural activity evoked by sensory input.
Importantly, these evoked waves in awake monkeys appear not to cross anatomical boundaries in the cortex (that is, between V1 and V2) 14 , unlike those observed in anaesthetized rodents 69 . In V1 and V2, the waves evoked in rapid succession by feedforward input are aligned so that they travel simultaneously across their respective retinotopic maps. This alignment is important because it could allow integration of retinotopic information in parallel across multiple regions of the visual system. Whether this stimulus-evoked alignment of travelling waves indeed occurs in multiple visual areas, in addition to whether spontaneous waves exhibit such alignment, remains an open question. However, consistent phase relationships have been observed both within individual visual areas (for example, in LFP recorded from V4 ( REF 75 ) and across different visual areas (in magnetoencephalography 43 ), indicating that such spatiotemporal patterning may be apparent in visual cortical dynamics during the awake state 76 .
Previous work noted that the phase of gamma oscillations changed across retinotopic domains in V1 of awake monkeys 77 and rabbits 78 as well as across orientation domains in V1 of awake monkeys 79 . This suggests that these multicycle high-frequency oscillations ride on top of a broader stimulus-evoked travelling wave and form sustained complex patterns that reflect features of the input. Furthermore, using a measure of directed information transfer across recording sites, a recent study found that shifts of the phase of gamma oscillations between pairs of electrodes in V1 of anaesthetized primates during stimulation with Hollywood movies are consistent with the presence of complex travelling waves propagating in the direction of maximal information transfer 30 . Importantly, these interactions were consistent with propagation along the horizontal-fibre network and not with input changes, illustrating that the effect of the horizontal-fibre networks in the visual cortex (and their intrinsic time delays) does not disappear in more naturalistic high-input situations. Taken together, these results indicate that stimulus-evoked travelling waves are a general and important feature of dynamics in the visual system that can affect neuronal processing. The functional importance of these waves, however, remains unclear.
The importance of finding travelling waves evoked by high-contrast visual stimuli lies in what it implies about cortical dynamics during normal sensory processing. It has previously been noted that stimulus-evoked travelling waves, which modulate the excitability of neurons as they travel across a topographic sensory map, appear inconsistent with the precise retinotopy and orientation topography described by Hubel and Wiesel at the columnar level 22 , 80 , 81 . If the visual system works to localize activity through retinotopic projections at each stage of processing, then why would it distribute activity across the map through travelling waves? Although initial results suggested that stimulus-evoked travelling waves were pronounced only at low luminance contrast 21 , thus minimizing consideration of their impact during normal visual processing 22 , the results reviewed above demonstrate that they are indeed an important feature of cortical dynamics that must be taken into account in describing the stimulus-evoked visual response at normal levels of contrast. These results indicate that activity evoked at one point in the visual cortex can, through the generation of a travelling wave, impact neurons quite far across the retinotopic map, and this affects how subsequent inputs are processed across visual space 82 . Rather than being a detriment to precise neuronal selectivity, however, these dynamic activity patterns may in fact be mediating previously unappreciated computations at the single-trial level. Theoreticians have postulated that a travelling wave with total phase offset between 0 and 2π could mark locations in the cortex with a unique phase that is useful for visual coding 83 ; however, it remains unclear how this can be used for neural computations with relevant ensembles of visual stimuli. We consider emerging ideas for computation with travelling waves below. Before this, however, we briefly consider the network-level mechanisms responsible for generating travelling waves at the mesoscopic and macroscopic scales.
Network mechanisms for travelling waves
As a paradigmatic example of a neural dynamic requiring the participation of millions of neurons and the recurrent connectivity of the cortex, the question of how travelling waves are generated and propagate has long attracted the attention of mathematicians and physicists. Computational and theoretical neuroscientists study cortical dynamics with a range of techniques, spanning from the mathematical continuum approximation of Wilson and Cowan 84 to the simulation of millions of neurons and billions of synapses on multicore and parallel computers 85 . With recent advances in neural recording and optogenetic stimulation technologies, experimental tests of predictions generated by these approaches are now being realized. The results of mathematical and computational studies on travelling waves in neural networks have been reviewed previously 32 , 83 , 86 , 87 ; here, we focus briefly on results that concern the waves and regime most pertinent to the studies reviewed above — that is, the sparse, weakly modulating waves that occur during the asynchronous and irregular state of neocortex in awake, behaving animals. We do not address the strongly modulating travelling waves that occur during pathological states, such as seizures and spreading depression; furthermore, we note that sparse travelling waves in the awake adult entail fundamentally different mechanisms than the slowly propagating waves observed during development 88 , 89 .
Macroscopic network mechanisms
At the whole-brain scale, neural recordings capture the effects of interaction among many billions of neurons across the cortex. Here, phase relationships among oscillations in individual cortical regions constitute the travelling wave, and interactions among regions can be effectively modelled by large-scale population descriptions, such as the Wilson-Cowan equations or coupled oscillator networks. Connections are mediated by cortical white matter fibres, which can traverse just below the grey matter when connecting two nearby regions or can run in the deep, long-range white matter tracts 60 , 90 . These connections have axonal conduction delays up to tens of milliseconds 35 , 60 and, as with mesoscopic waves, these distance-dependent delays can strongly influence the emergence of spatiotemporal patterns at the macroscopic level. It has been shown that distance- dependent delays lead to a rich repertoire of spatiotemporal dynamics, including radial and spiral waves, in networks of coupled oscillators 91 . Furthermore, in simplified networks, mathematical analysis has established that highly structured, geometric connection patterns for networks arranged on a sphere can lead to rotating waves 92 . Further work is required, however, to understand how alpha band and spindle macroscale travelling waves emerge through the dynamics and time delays of the corticothalamic system.
Mesoscopic network mechanisms
In the cortex, neurons are embedded in a sea of so-called spontaneous activity. Pyramidal cells in V1 have dense recurrent synaptic connectivity, each receiving from 6,000 to 13,000 inputs per cell 93 . Roughly 80% of these connections come from within the same cortical area, 95% of which arise from neurons within 2 millimetres of the receiving cell 94 . Long-range horizontal fibres can span several milli metres across the supragranular layers of the cortex 95 . These axons thus connect neurons spanning multiple functional hypercolumns within a cortical map. During the awake state, pyramidal cells in V1 of monkeys exhibit a median spontaneous firing rate of 7 Hz ( REF. 96 ). This indicates that neurons in V1 of primates receive tens of thousands of synaptic inputs per second, with most arriving from the mesoscopic, intracortical circuit. This synaptic noise has well-known effects on the integration properties of cortical neurons 97 , allowing them to respond quickly to transient input fluctuations 98 , and forms the basis for the difference in network state between anaesthetized to awake 99 .
Travelling waves transiently modulate this ongoing synaptic noise as they pass. For example, there is evidence that, depending on the specific cell-type recruitment, oscillations in the hippocampus may either excite or inhibit neurons in the local circuit at various points during the cycle 100 . Similarly, during normal cortical function in awake animals, travelling waves weakly modulate neural spiking activity in the motor cortex 19 . This is consistent with observations at single points in the visual cortex, where alpha and gamma oscillations weakly entrain spiking activity 101 – 103 , increasing and decreasing spike probability over the oscillation cycle. Here, we refer to this type of wave (in which the probability of any individual neuron participating in the wave is low) as a sparse wave ( FIG. 4 ). This stands in contrast to the travelling waves observed in in vitro models of epilepsy 104 , 105 , where dense waves dominate the spiking activity of the local circuit as they pass. Under these in vitro conditions, networks lack a large fraction of the spontaneous synaptic input that is present in vivo and furthermore are often studied in disinhibited conditions

Two schematic models for wave generation in topographic networks of neurons with local, random connections and linearly increasing axonal conduction delays are illustrated. Spheres represent neurons whose membrane potential is indicated by colour. a | In a cortical network with no background activity (as observed in deep anaesthesia or often in cortical slices in vitro), we hypothesize that local stimulation will elicit strong travelling waves, which recruit nearly all cells as they pass. Arrows indicate directions of wave propagation. b | In a cortical network with strong background activity (as observed in normal waking states), we hypothesize that local stimulation can elicit waves that weakly entrain neuronal spiking as they travel across the network.
When a burst of spikes arrives at a local patch of the cortex, it evokes a brief increase in spiking that can propagate out across the network 24 , 67 , 69 . As outlined above, while most studies have focused on waves during the stimulus onset response, travelling waves may be induced during sustained stimulation responses and during the brief increase of spiking activity at stimulus offset in the sensory cortex of awake, behaving animals, and these waves may not be time-locked to the stimulus. Bursts during the fast onset transient arise through feedforward input; however, during spontaneous and induced waves, these bursts can result from recurrent fluctuations or feedback connections 26 , 106 . Nevertheless, initial evidence indicates that induced waves travel over similar fibre networks as waves evoked during stimulus onset 66 , 77 .
The key factor governing the generation and propagation of stimulus-evoked, induced and spontaneous travelling waves at the mesoscale is the axonal conduction delay of the horizontal fibres of superficial layers in the cortex 14 , 23 , 67 . These fibres project over long distances across the cortical sheet 95 , 107 , providing the substrate for travelling waves and governing their amplitude decay. Computational and mathematical studies have demonstrated the role of distance-dependent axonal conduction delays in generating stimulus-evoked waves in recurrent networks 87 , 108 , 109 . Specifically, it has been shown that distance- dependent delays can destabilize synchrony in coupled oscillator networks, leading to travelling waves 110 . However, it remains unknown whether and how spontaneous and stimulus-evoked waves can be generated in large-scale spiking networks with biologically realistic synaptic noise, in which model neurons exhibit the irregular asynchronous (IA) state that is the hallmark of cortical dynamics 111 . Stochastic neural field theory has studied the effect of noise on propagation of travelling waves in neural systems 112 ; however, because the noise generated by networks in the IA state has a specific and nonstationary signature 113 , the dynamics of large-scale spiking networks may differ substantially from those of stochastic neural fields, and few detailed comparisons have been done.
In computational models of spiking networks, the degree to which neurons are entrained by travelling waves is a key factor in determining the dynamics and interactions of waves. In small models, in which synaptic weights are strong and correlations are increased 114 (a rough analogue of biological networks in vitro), travelling waves are dense and strongly entrain neurons in the local circuit as they pass ( FIG. 4a ). Waves in such networks generally exhibit repelling interactions 115 , 116 , in which two colliding waves will either disappear or deflect each other onto new paths. In larger networks, however, with network states matching those in the cortex and sparse (weakly entraining) waves ( FIG. 4b ), these interactions could be much different, with waves weakly interacting while passing through each other as they travel. These differences in wave interaction suggest vastly different general computational principles, to which we turn in the next section.
Emerging computational principles
As introduced above and noted previously 22 , spontaneous and stimulus-evoked travelling waves add a spatiotemporal context to the orderly response properties of the visual cortex first described by Hubel and Wiesel 80 , 81 . In the canonical model of visual processing (and, indeed, of sensory processing in general), cortical neurons are independent feature detectors that build progressively more complex representations through successive elaboration of receptive field selectivity in each cortical region 117 . Stimulus-evoked travelling waves in the visual cortex introduce strong contextual influences into this model of cortical dynamics: for example, if two nearby sensory stimuli are presented in succession, the first may generate a travelling wave that has an effect on the response to the second, transiently modulating the receptive field properties of neurons whose receptive fields contain the second stimulus. Such contextual modulations have been discussed previously in connection with the notion of ‘non-classical’ receptive fields 118 – 121 , which can implement spatial interactions that are well captured by the theory of association fields ( REFS 122 – 124 ). Evidence that travelling waves actively influence visual processing, for example, by producing cortical correlates of low-level visual percepts such as the line-motion illusion 24 , 125 or the perceived speed of apparent motion stimuli 126 , 127 , has been reported by linking cortical dynamics during anaesthetized states to human psychophysical data. An overview of observed cortical travelling waves and their potential computational roles is given in TABLE 1 . A comprehensive conceptual framework for travelling waves and dominant recurrent interactions during normal awake sensory processing is, however, lacking.
LFP, local field potential; M1, primary motor cortex; NA, not applicable; PMd, dorsal premotor cortex; V1, primary visual cortex; V2, secondary visual cortex; V4, visual area V4.
Converging evidence shows that the canonical model of visual processing is excellent for coding stationary images but has difficulty explaining processing of dynamic, nonstationary naturalistic stimuli 128 . For example, standard models of cortical neurons based on this framework capture only a fraction of the response variance observed under natural sensory conditions 129 . Below, we first review specific examples of functional roles for waves recently found in sensorimotor conditions and the hippocampus and then, inspired by a physical process for information storage by dynamic wave fields, introduce a general conceptual framework for cortical travelling waves in the processing of visual scenes.
Waves and sensorimotor coordination
Beta-frequency (15–30 Hz) oscillations have a strong connection to movement preparation and motor coordination 130 , and their spatiotemporal properties have been a subject of much interest in recent years. In the motor cortex of monkeys, beta oscillations before movement initiation during an instructed-delay reach task are waves travelling in a consistent anatomical direction 131 . Similar dynamics were found in the motor cortex of humans 132 . It was later confirmed that these LFP travelling waves modulate spiking activity at the single-unit level 19 . In the context of the instructed-delay reach task, the travelling waves in the motor cortex have a plausible proposed functional role: they have been suggested to facilitate sequences of activation in proximal-to-distal muscle representations in preparation for reaching behaviour 133 , 134 . While detailed future studies could illustrate the effect of recorded cortical activity on specific resulting patterns of muscle activation 19 , these observations provided a first link between cortical travelling waves and physiologically relevant neural outputs, suggesting that they play distinct functional roles.
In similar sensorimotor conditions in the visual system, saccades have been found to induce beta frequency travelling LFP waves in visual area V4 ( REF. 29 ). These waves travel from foveal to peripheral regions of the V4 retinotopic map, creating a spatiotemporal pattern of post-saccadic excitability. Various functional roles are possible for this pattern, including saccadic suppression (useful in eliminating blurred visual scenes during eye movements) 135 and motor guidance in planning the following saccade. While their exact functional role remains unclear, these saccade-induced travelling waves in the visual system represent a second sensorimotor condition that may be probed by future work for a clear link between cortical travelling waves and physiological function.
Travelling waves in the hippocampus
Hippocampal population rhythms are some of the most well-studied oscillations in the brain and are of particular interest because of their important connections to learning and long-term memory 136 . The 6–12 Hz theta oscillation modulates hippocampal LFP and pyramidal cell spiking during active exploration and rapid-eye-movement sleep 15 , 137 , organizing the firing of hippocampal place cells in a crucial manner 138 . Long thought to be approximately synchronous throughout the hippo campal formation 139 , theta oscillations have recently been shown to be waves travelling along the dorsoventral axis of CA1 ( REFS 17 , 18 , 140 ) ( FIG. 5a ). Because hippocampal place fields become progressively larger along this axis 141 , 142 ( FIG. 5b ), these travelling theta waves organize CA1 place cell spikes into a sweep from the finest to the coarsest scales of spatial selectivity 17 , 18 ( FIG. 5c ), possibly linking high-precision spatial information with broader contextual content. In addtion, the specific spatiotemporal structure of the LFP during these travelling theta waves has been shown to contain precise information about an animal's location 143

a | The 6–12 Hz hippocampal theta oscillation, which appears during active behavioural exploration and rapid-eye-movement sleep, is a wave travelling from dorsal to ventral hippocampal CA1 (REFS 17,18). This flattened map view of the rodent hippocampus shows the positions of the dentate gyrus (DG), CA3, CA1, subiculum (S) and entorhinal cortex (ENT). The phase offset of the theta oscillation is plotted in colour along the dorsoventral axis of CA1 (colour axis, bottom left). b | As the rodent runs on a linear track, pyramidal neurons in CA1 fire. These neurons exhibit place fields that become progressively larger as their location in CA1 changes from dorsal to ventral regions 141 , 142 . The schematic illustrates a typical example of the place fields of neurons that fire when the rodent is at a particular spatial location during the run and is based on data in REF 17 . The place fields are arranged according to their location in CA1 and with their colour illustrating the phase offset of the theta oscillation at that location on the dorsoventral axis. c | Because of the phase offset of the theta local field potential (LFP; as recorded in stratum pyramidale18; shown as light transparent lines) owing to the travelling wave (represented by the distance between the dotted lines) and the modulation of neuronal firing by theta (solid lines), neurons with CA1 place fields centred at the rodent's position fire in a temporal sweep from those with the smallest scales of spatial selectivity to those with the largest scales of spatial selectivity. Part a is reproduced from REF. 17 , Macmillan Publishers Limited. Part c is adapted with permission from REF. 18 , Elsevier.
High-frequency 140-200 Hz ripple oscillations, which appear during awake resting and slow-wave sleep 144 and are involved in replay of waking hippocampal spike sequences during sleep 145 , have also been shown to be travelling waves, starting at discrete points along the dorsoventral axis and propagating in either the dorsal or ventral direction 146 . The computational consequences of this exquisite mesoscopic organization of firing patterns by theta and ripple travelling waves in the hippocampus have not yet been investigated in detail; however, they have implications for theories involving synaptic plasticity as a mechanism for linking neurons across the hippocampus 147 , 148 .
Potential computational roles for stimulus-evoked travelling waves
In the specific examples presented above, the existence of travelling waves is not in conflict with what is known about the underlying physiology: in both cases, travelling waves may organize population spiking along some behaviourally relevant axis (in a sweep from proximal to distal muscle representations, foveal to peripheral visual fields or small to large place fields). As outlined at the beginning of this section, however, a coherent account of computational roles for stimulus- evoked waves in the sensory cortex is lacking. At present, we cannot exclude the possibility that no specific computational role exists for spontaneous and stimulus-evoked waves and that they may be an epiphenomenon of the massive recurrent connectivity in the cortex. A direct demonstration of the function of these waves in sensory processing is lacking, most importantly, in the awake animal. However, it is clear that the information carried by these waves could play important computational roles. Stimulus-evoked travelling waves in V1 extend well beyond the locus of the initial stimulus- evoked response, broadly influencing neurons over several millimetres of the retinotopic map 14 , 24 , 25 , 67 . Furthermore, it is important to consider that these waves travel over other functional maps such as ocular dominance and orientation maps in V1 and have been shown to distribute orientation specific information across the cortex (though this specificity decreases over a moderately short distance in the cortex) 25 , 149 , 150 . This suggests that waves will be useful in visual processing on scales beyond the imprint of feedforward activation, opening up interesting new computational capacities. To explore this further, we now consider general models for computation with evoked waves travelling over sensory maps in the cortex.
Computation with waves has been a subject of continued interest in reaction-diffusion systems 151 , with some applications to spiking neural networks 116 . In these models, a localized wave state (generally a small travelling excitation) is considered a unit (or packet) of information, with computations implemented by interactions between localized wave states. For example, the merging of two waves might serve to compute an AND logic operation, while the annihilation of two waves may represent a dynamic XOR gate. These collision-based computations 152 , however, require dense wave interactions of the kind observed in small spiking networks with low numbers of synaptic connections per cell (see above). In the weakly entrained regime consistent with the low-correlated firing in physiologically normal cortex 153 , 154 , sparse waves may interact much less strongly as they travel than dense waves. In this situation, multiple evoked waves could lead to a global spatiotemporal pattern of activation whose usefulness in computation must be considered within a different context.
What computations can be done with evoked, weakly interacting travelling waves in single cortical regions? For inspiration, we can look to a recently described physical process as an analogue. A recent study examined the dynamics of a silicone droplet bouncing on a vibrating oil bath 155 . The vibrations of this medium propel the droplet upward and cause it to bounce continually, creating a pattern of successive impacts across the surface ( FIG. 6a ). With each bounce, the droplet creates a set of waves in the oil bath. In our analogy, these droplet waves may be similar to those created in the sensory cortex by the impact of afferent, stimulus-driven action potentials. The waves evoked by each impact of the droplet then create a pattern across the oil bath that leaves a trace of the path of the droplet. With sufficient knowledge of the dynamic properties of the medium, one could decode the history of the path of the droplet simply from the observed spatial pattern at each point in time. Furthermore, temporal reversibility is preserved for waves even in chaotic systems; this property, along with a clever manipulation of the experimental apparatus, can be used to cause the droplet to exactly reverse its trajectory ( FIG. 6a ). It is argued that this last manipulation shows that the global wave field stores information about the trajectory of the droplet with sufficient precision that it can be read back out. While the authors did not have neuroscience in mind and, indeed, note that they have not yet considered practical implementations, they posit that this system implements the basic elements for general computations that could find application in future work.

a | A droplet emits travelling radial waves as it bounces and creates a trajectory (white arrow) across the surface of a silicone oil bath (left panel) 155 . As it bounces, the droplet creates a forward trajectory (blue line, right panel). The x axis, y axis and z axis represent the position of the droplet in space. With a simple manipulation, or introduced shift (a higher bounce, black line), the droplet can reverse its trajectory (red line), illustrating that the evoked wave field stores information about the recent past. b | Schematic models of standing bumps and travelling waves evoked by an external stimulus (red dot) are shown. As time increases (from 1 to 4 time units (t)), the standing bump reaches a steady pattern, whereas the travelling wave continues to expand across the network. It is therefore hypothesized that time from stimulus onset can be decoded in the case of travelling waves but not standing bumps. c | It is hypothesized that two sparse waves interacting in the cortex will weakly interact, passing through each other (unlike dense waves) and creating a global wave field with excitations that are symmetric about each stimulated point. Part a is adapted with permission from REF. 155 . Copyrighted by the American Physical Society.
This physical system, though in many ways an imperfect analogy to the sensory cortex, provides a suggestive example for possible computations that could be performed by waves radiating from the retinotopic loci of stimulus-evoked input. The key difference between stimulus-driven stationary bumps of activity and active travelling waves is that the latter contain information about time from stimulus onset ( FIG. 6b ). While stationary bumps contain information about stimulus position in a retinotopic map, in a network with active stimulus-evoked travelling waves, one could decode both stimulus position and onset time. During the lifetime of waves travelling over a retinotopic map, they could therefore encode both stimulus position and the recent past in a distributed spatiotemporal pattern of activation ( FIG. 6c ). The availability of information about the recent past may be useful in processing dynamic, non-stationary sensory scenes, which present difficulties for the static feedforward framework 128 , 129 . It is important to note that, though other activity patterns (such as expanding bumps) could encode both stimulus position and onset time, such activity patterns will be less robust than travelling waves arising from multiple points of stimulation. Similar ideas for computation have been discussed previously 156 but never before in the context of sensory systems and cortical topographic maps. Finally, because of their timing relative to sensory stimuli, induced waves during sustained responses may exhibit a much richer interaction between feedforward and feedback pathways in the cortex; thus, they may serve different computational roles than waves evoked by the initial feedforward input. However, because induced waves will propagate similarly across topographic maps in the sensory cortex, the framework discussed here can serve as a guiding example for further investigations.
This framework could potentially provide a general outline for computation with travelling waves in the sensory cortex, helping to resolve the difficulties posed by stimulus-evoked travelling waves to the canonical feedforward model of visual processing. Further experimental and theoretical work will be needed to further refine our understanding of travelling waves in these regions. Here, however, we have highlighted the importance of multi-wave interactions and suggest that they provide fertile ground for future experimental and theoretical work. Analysis of multichannel recordings will make it possible to test whether stimulus position and timing can be reliably decoded from responses at the single-trial level. While it has been noted that cortical travelling waves may structure visual responses to dynamic natural scenes 30 , it remains unclear how the spatiotemporal interactions revealed following simple sensory stimuli 14 generalize to multi-stimulus paradigms and natural scenes. Nevertheless, the effect of the abundant horizontal connections highlighted in this work (and their intrinsic axonal time delays) is not expected to disappear. Furthermore, it remains unknown how spontaneous and stimulus-evoked mesoscopic waves may influence computations in downstream cortical areas. Improved imaging techniques 52 , 157 and new optogenetic approaches in awake, behaving primates 158 , 159 may allow future studies to directly test the functional roles for cortical travelling waves discussed here. Central to this framework is the recognition that the fine-scale dynamics of neural populations can carry important additional information over sensory topographic maps. Even more complex interactions in higher-order association regions and in prefrontal cortex could be involved in cognitive functions such as planning and decision making.
Conclusions
In this Review, we have covered recent advances in travelling waves in neural systems. While initial evidence indicated that travelling waves were pronounced only in weak input regimes 21 , 22 , recent studies have shown that travelling waves are evoked following small, high-contrast visual stimuli 14 , and they appear to play a role in cortical dynamics driven by dynamic sensory input and sensorimotor planning 30 . It has previously been noted that the existence of stimulus-evoked travelling waves in the sensory cortex presents a challenge to the orderly topographic arrangement of selectivity first described by Hubel and Wiesel at the trial-averaged level 22 . Here, we have outlined an initial conceptual framework for computations with travelling waves in the sensory cortex. This regime, in which activity patterns generated by intracortical circuits actively shape responses to external stimuli, is well matched to the basic anatomy of the cortex, where neurons receive a large fraction of their input from a local patch of cells in the same region 94 . Much theoretical and experimental work remains to test the feasibility of computations with travelling waves in the cortex; however, the development of large-scale, high-precision recording technologies is a priority in neuroscience, which will drive advances in these fields.
Acknowledgments
The authors thank Z. Davis, T. Bartol, G. Pao, A. Destexhe, Y Frégnac and C. F. Stevens for helpful discussions and J. Ogawa for helpful discussions and help with illustrations. L.M. acknowledges support from the US National Institute of Mental Health (5T32MH020002-17). F.C. acknowledges support from Agence Nationale de la Recherche (ANR) projects BalaV1 (ANR-13-BSV4-0014-02) and Trajectory (ANR- 15-CE37-0011-01). J.R. acknowledges support from the Fiona and Sanjay Jha Chair in Neuroscience at the Salk Institute. T.J.S. acknowledges support from Howard Hughes Medical Institute, Swartz Foundation and the Office of Naval Research (N000141210299).
Author contributions: L.M., F.C, J.R. and T.J.S. researched data for the article, made substantial contributions to discussions of the content, wrote the article and reviewed and/or edited the manuscript before submission.
Competing interests: The authors declare no competing interests
Publisher's note: Springer Nature remains neutral with regard to jurisdictional claims in published maps and institutional affiliations.
Reviewer information: Nature Reviews Neuroscience thanks Gustavo Deco, Pascal Fries and the other anonymous reviewer(s) for their contribution to the peer review of this work.
Talk to our experts
1800-120-456-456
- Travelling Wave
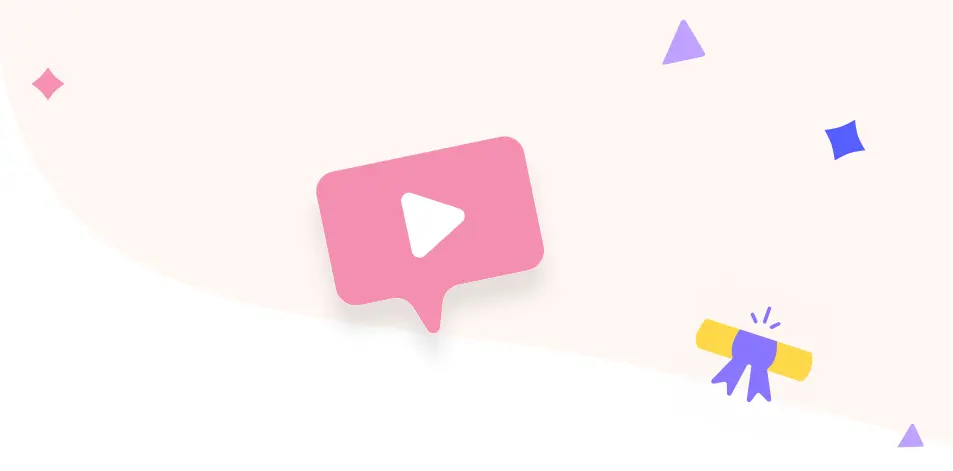
Have you ever sat by a lake and observed the waves created on the surface of the water when you throw a stone into it? This is a good visual example of the propagation of waves and makes it simpler for you to understand travelling of waves and all other concepts related to it. Our universe has an amazing way of informing us about any changes in the physical world. When there are changes the information about that disturbance moves gradually outwards. It moves far from the source of disturbance in all the directions. When the said information travels, it travels in the form of a wave, just like the way waves are created when you throw a stone in the still water. This is known as the travelling wave.
Define Travelling Wave
(image will be uploaded soon)
Before understanding what is travelling wave, let’s understand waves. Wave can be defined as a disturbance in a medium that travels transferring momentum and energy without any actual movement of the medium. However, the medium must have elastic properties. In our everyday life, there are many examples of waves, for example, ocean waves, strings of musical instruments, etc. On the other hand, a travelling wave is a wave in which the positions of minimum and maximum amplitude travel through the medium.
Points To Remember
Here are some of the points that are necessary to keep in mind about the wave:
Every wave has a high point and a low point. The high points are known by the name of crests. On the other hand, the low points are named by troughs.
Amplitude is the maximum distance of the disturbance from the midpoint of the wave to either the top of the crest or the bottom of a trough.
The maximum distance between the two adjacent troughs or the two adjacent crests is known as a wavelength.
Now, the time period is actually the time taken to complete one vibration.
Frequency is the number of vibrations the wave undergoes in one second.
You can witness an inverse relationship between both frequency and time period. The relationship is given below,
The speed of a wave is given by the travelling wave equation,
Where 𝛌 is the wavelength.
What are the Various Types of Travelling Waves?
Each type of wave contains different characteristics. And with these characteristics, we can easily distinguish between them. Here is a list of different types of waves that have been categorized based upon their particle motion.
Pulse Waves - the sudden disturbance that travels through a medium is known as a pulse wave. The disturbance can be caused by a chain reaction or sudden compression of air caused by an explosion. One example of a pulse wave is thunder. It comprises only one crest that travels through the transmission medium.
Continuous Waves - it is an electromagnetic wave that has constant amplitude and frequency. It is a typical sine wave and is considered to be of infinite duration. It was used in the earlier days of radio transmission.
Transverse Waves - in the transverse wave, the movement of the particles is at right angles to the motion of the energy. It is generated through a solid object like a stretched rope. Trampoline is the best example to understand this wave.
Longitudinal Waves - in this type of travelling wave the motion of the wave-particle is in the same direction as the propagation of the wave. In simple words, the movement of the particles is parallel to the motion of the energy. The best example for longitudinal waves is sound waves moving through the air when you hear a loudspeaker playing in the distance.
There is a second way to characterize the waves by types of matter they are able to move or travel through.
Electromagnetic Waves - this type of wave can travel easily through a vacuum. It does not need any medium, soft or hard to travel. An example of an electromagnetic wave is mobile phone waves or sound waves. They don't need any vacuum to travel.
Physical waves - Unlike electromagnetic waves, they require a medium to travel. They are further distinguished on the basis of phases of matter through which they can move.
Longitudinal Waves - these waves can easily pass through liquids and games.
Transverse Waves - they require a solid material or medium to propagate.
Problems based on travelling wave equation
Solved Examples
1: A wave on a rope is shown on the right at some time t. What is the wavelength of this wave? If the said frequency is about 4 Hz, what will be the wave speed?
Now, for all the periodic waves, you will find v = λ/T = λf.
Details of the calculation:
The wavelength λ is 3 m. The speed is v = λf = (3 m)(4/s) = 12 m/s.
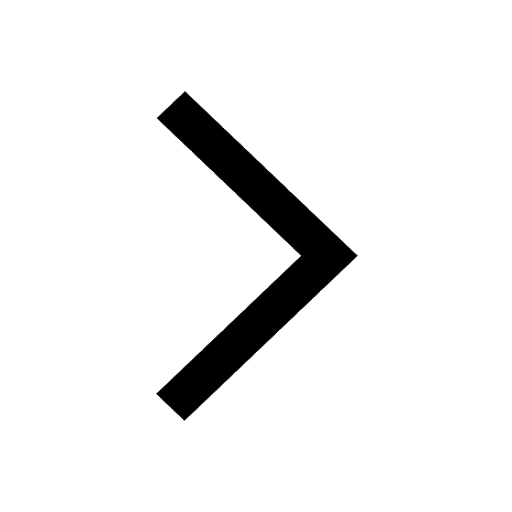
FAQs on Travelling Wave
1. Do all the Waves have a Defined Wavelength?
Yes, every wave has a specific wavelength, which is defined as the length from one wave crest to the next.
Different kinds of waves have different wavelengths. In water, the surf waves produced by a surfer, have wavelengths of 30–50 m, and the large tsunamis have much longer wavelengths (about 100km). The Sound waves vary in wavelength according to the pitch of it.
2. At What Wavelengths can a Human Hear a Sound?
As per experts and facts, the humans can very well hear sounds possessing wavelengths between 70 mm and 70 m. Any sound above or below this level can not be heard by a human. Example- our universe is filled with cosmic noises. The wavelength of the noise is so high that we cannot hear it on earth.
How to Detect and Analyze Traveling Waves in Human Intracranial EEG Oscillations?
- First Online: 02 August 2023
Cite this chapter
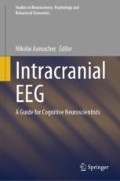
- Anup Das 4 ,
- Erfan Zabeh 4 , 5 &
- Joshua Jacobs 4 , 6
Part of the book series: Studies in Neuroscience, Psychology and Behavioral Economics ((SNPBE))
1799 Accesses
1 Altmetric
The brain is a complex, interconnected network and the large-scale spatiotemporal coordination of neuronal activity is vital for cognition and behavior. Prior studies have proposed that traveling waves of brain oscillations are one mechanism that helps coordinate complex neuronal processes and are crucial for cognition. Traveling waves consist of oscillations that propagate progressively across the cortex and previous studies have shown that these waves play a foundational role for learning, memory processing, and memory consolidation and a range of other behaviors across multiple species. The prevalence of traveling waves in cognition thus indicates that spatiotemporal patterns of neuronal oscillations may coordinate multiple neuronal brain networks and impact behavior. Even though there are several different approaches for analyzing traveling waves using electrophysiological recordings, computational tools targeting the analysis and visualization and understanding of traveling waves are still rare. We briefly review the literature on human intracranial electroencephalography (iEEG), which has shown that traveling waves play an important role in cognition. We then describe a statistical methodology based on circular–linear regression for the detection and analysis of traveling waves from human electrophysiological oscillations. We hope that this approach will provide a more mechanistic understanding of the coordination of neurons across space and time.
This is a preview of subscription content, log in via an institution to check access.
Access this chapter
- Available as PDF
- Read on any device
- Instant download
- Own it forever
- Available as EPUB and PDF
- Durable hardcover edition
- Dispatched in 3 to 5 business days
- Free shipping worldwide - see info
Tax calculation will be finalised at checkout
Purchases are for personal use only
Institutional subscriptions
Buzsáki G (2000) Rhythms of the brain. Oxford University Press, New York
MATH Google Scholar
Buzsaki G, Draguhn A (2004) Neuronal oscillations in cortical networks. Science 304(5679):1926–1929
Article Google Scholar
Berger H (1929) Über das Elektrenkephalogramm des Menschen. Archiv f Psychiatrie 87:527–570
Jasper H, Penfield W (1949) Electrocorticograms in man: effect of voluntary movement upon the electrical activity of the precentral gyrus. Arch Psychiatr Nervenkr 183:163–174
Muller L, Chavane F, Reynolds J, Sejnowski TJ (2018) Cortical travelling waves: mechanisms and computational principles. Nat Rev Neurosci 19(5):255–268
Uhlhaas PJ, Pipa G, Lima B, Melloni L, Neuenschwander S, Nikolić D et al (2009) Neural synchrony in cortical networks: history, concept and current status. Front Integr Neurosci 3:17
Bahramisharif A, van Gerven MA, Aarnoutse EJ, Mercier MR, Schwartz TH, Foxe JJ et al (2013) Propagating neocortical gamma bursts are coordinated by traveling alpha waves. J Neurosci 33(48):18849–18854
Dickey CW, Sargsyan A, Madsen JR, Eskandar EN, Cash SS, Halgren E (2021) Travelling spindles create necessary conditions for spike-timing-dependent plasticity in humans. Nat Commun 12(1):1027
Halgren M, Ulbert I, Bastuji H, Fabó D, Erőss L, Rey M et al (2019) The generation and propagation of the human alpha rhythm. Proc Natl Acad Sci U S A 116(47):23772–23782
Hangya B, Tihanyi BT, Entz L, Fabó D, Erőss L, Wittner L et al (2011) Complex propagation patterns characterize human cortical activity during slow-wave sleep. J Neurosci 31(24):8770–8779
Kleen JK, Chung JE, Sellers KK, Zhou J, Triplett M, Lee K et al (2021) Bidirectional propagation of low frequency oscillations over the human hippocampal surface. Nat Commun 12(1):2764
Muller L, Piantoni G, Koller D, Cash SS, Halgren E, Sejnowski TJ (2016) Rotating waves during human sleep spindles organize global patterns of activity that repeat precisely through the night. Elife 5
Google Scholar
Stolk A, Brinkman L, Vansteensel MJ, Aarnoutse E, Leijten FS, Dijkerman CH et al (2019) Electrocorticographic dissociation of alpha and beta rhythmic activity in the human sensorimotor system. Elife 8
Zhang H, Jacobs J (2015) Traveling theta waves in the human hippocampus. J Neurosci 35(36):12477–12487
Zhang H, Watrous AJ, Patel A, Jacobs J (2018) Theta and alpha oscillations are traveling waves in the human neocortex. Neuron 98(6):1269–1281.e4
Besserve M, Lowe SC, Logothetis NK, Schölkopf B, Panzeri S (2015) Shifts of gamma phase across primary visual cortical sites reflect dynamic stimulus-modulated information transfer. PLoS Biol 13(9):e1002257
Davis ZW, Muller L, Martinez-Trujillo J, Sejnowski T, Reynolds JH (2020) Spontaneous travelling cortical waves gate perception in behaving primates. Nature 587(7834):432–436
Gabriel A, Eckhorn R (2003) A multi-channel correlation method detects traveling gamma-waves in monkey visual cortex. J Neurosci Methods 131(1–2):171–184
Muller L, Reynaud A, Chavane F, Destexhe A (2014) The stimulus-evoked population response in visual cortex of awake monkey is a propagating wave. Nat Commun 5:3675
Nauhaus I, Busse L, Carandini M, Ringach DL (2009) Stimulus contrast modulates functional connectivity in visual cortex. Nat Neurosci 12(1):70–76
Townsend RG, Solomon SS, Chen SC, Pietersen AN, Martin PR, Solomon SG et al (2015) Emergence of complex wave patterns in primate cerebral cortex. J Neurosci 35(11):4657–4662
Vinck M, Lima B, Womelsdorf T, Oostenveld R, Singer W, Neuenschwander S et al (2010) Gamma-phase shifting in awake monkey visual cortex. J Neurosci 30(4):1250–1257
Zanos TP, Mineault PJ, Nasiotis KT, Guitton D, Pack CC (2015) A sensorimotor role for traveling waves in primate visual cortex. Neuron 85(3):615–627
Balasubramanian K, Papadourakis V, Liang W, Takahashi K, Best MD, Suminski AJ et al (2020) Propagating motor cortical dynamics facilitate movement initiation. Neuron 106(3):526–536.e4
Denker M, Zehl L, Kilavik BE, Diesmann M, Brochier T, Riehle A et al (2018) LFP beta amplitude is linked to mesoscopic spatio-temporal phase patterns. Sci Rep 8(1):5200
Rubino D, Robbins KA, Hatsopoulos NG (2006) Propagating waves mediate information transfer in the motor cortex. Nat Neurosci 9(12):1549–1557
Rule ME, Vargas-Irwin C, Donoghue JP, Truccolo W (2018) Phase reorganization leads to transient β-LFP spatial wave patterns in motor cortex during steady-state movement preparation. J Neurophysiol 119(6):2212–2228
Takahashi K, Kim S, Coleman TP, Brown KA, Suminski AJ, Best MD et al (2015) Large-scale spatiotemporal spike patterning consistent with wave propagation in motor cortex. Nat Commun 6:7169
Bhattacharya S, Brincat SL, Lundqvist M, Miller EK (2022) Traveling waves in the prefrontal cortex during working memory. PLoS Comput Biol 18(1):e1009827
Zabeh E, Foley NC, Jacobs J, Gottlieb JP (2022) Traveling waves in the monkey frontoparietal network predict recent reward memory. bioRxiv
Liang Y, Song C, Liu M, Gong P, Zhou C, Knöpfel T (2021) Cortex-wide dynamics of intrinsic electrical activities: propagating waves and their interactions. J Neurosci 41(16):3665–3678
Stroh A, Adelsberger H, Groh A, Rühlmann C, Fischer S, Schierloh A et al (2013) Making waves: initiation and propagation of corticothalamic Ca2+ waves in vivo. Neuron 77(6):1136–1150
Xu W, Huang X, Takagaki K, Wu JY (2007) Compression and reflection of visually evoked cortical waves. Neuron 55(1):119–129
Agarwal G, Stevenson IH, Berényi A, Mizuseki K, Buzsáki G, Sommer FT (2014) Spatially distributed local fields in the hippocampus encode rat position. Science 344(6184):626–630
Patel J, Fujisawa S, Berényi A, Royer S, Buzsáki G (2012) Traveling theta waves along the entire septotemporal axis of the hippocampus. Neuron 75(3):410–417
Patel J, Schomburg EW, Berényi A, Fujisawa S, Buzsáki G (2013) Local generation and propagation of ripples along the septotemporal axis of the hippocampus. J Neurosci 33(43):17029–17041
Alamia A, VanRullen R (2019) Alpha oscillations and traveling waves: signatures of predictive coding? PLoS Biol 17(10):e3000487
Alexander DM, Jurica P, Trengove C, Nikolaev AR, Gepshtein S, Zvyagintsev M et al (2013) Traveling waves and trial averaging: the nature of single-trial and averaged brain responses in large-scale cortical signals. Neuroimage 73:95–112
Lozano-Soldevilla D, VanRullen R (2019) The Hidden spatial dimension of alpha: 10-Hz perceptual echoes propagate as periodic traveling waves in the human brain. Cell Rep 26(2):374–380.e4
Mahjoory K, Schoffelen JM, Keitel A, Gross J (2020) The frequency gradient of human resting-state brain oscillations follows cortical hierarchies. Elife 9
Massimini M, Huber R, Ferrarelli F, Hill S, Tononi G (2004) The sleep slow oscillation as a traveling wave. J Neurosci 24(31):6862–6870
Zich C, Quinn AJ, Bonaiuto JJ, O’Neill G, Mardell LC, Ward NS et al (2022) Spatiotemporal organization of human sensorimotor beta burst activity. bioRxiv
Das A, Myers J, Mathura R, Shofty B, Metzger BA, Bijanki K et al (2022) Spontaneous neuronal oscillations in the human insula are hierarchically organized traveling waves. Elife 11
Mercier MR, Dubarry AS, Tadel F, Avanzini P, Axmacher N, Cellier D et al (2022) Advances in human intracranial electroencephalography research, guidelines and good practices. Neuroimage 119438
Parvizi J, Kastner S (2018) Promises and limitations of human intracranial electroencephalography. Nat Neurosci 21(4):474
Donoghue T, Haller M, Peterson EJ, Varma P, Sebastian P, Gao R et al (2020) Parameterizing neural power spectra into periodic and aperiodic components. Nat Neurosci 23(12):1655–1665
Watrous AJ, Miller J, Qasim SE, Fried I, Jacobs J (2018) Phase-tuned neuronal firing encodes human contextual representations for navigational goals. Elife 7
Solomon EA, Stein JM, Das S, Gorniak R, Sperling MR, Worrell G et al (2019) Dynamic theta networks in the human medial temporal lobe support episodic memory. Curr Biol 29(7):1100–1111.e4
Tarjan R (1972) Depth-first search and linear graph algorithms. Siam J Comput 1(2):146–160
Article MathSciNet MATH Google Scholar
Fisher NI (1993) Statistical analysis of circular data. Cambridge University Press
Mohan UR, Zhang H, Jacobs J (2022) The direction and timing of theta and alpha traveling waves modulate human memory processing. bioRxiv
Ermentrout GB, Kopell N (1984) Frequency plateaus in a chain of weakly coupled oscillators I. SIAM J Math Anal 15(2):215–237
Alexander DM, Nikolaev AR, Jurica P, Zvyagintsev M, Mathiak K, van Leeuwen C (2016) Global neuromagnetic cortical fields have non-zero velocity. PLoS ONE 11(3):e0148413
Hindriks R, van Putten M, Deco G (2014) Intra-cortical propagation of EEG alpha oscillations. Neuroimage 103:444–453
Lubenov EV, Siapas AG (2009) Hippocampal theta oscillations are travelling waves. Nature 459(7246):534–539
Canolty RT, Edwards E, Dalal SS, Soltani M, Nagarajan SS, Kirsch HE et al (2006) High gamma power is phase-locked to theta oscillations in human neocortex. Science 313(5793):1626–1628
Friston KJ (2019) Waves of prediction. PLoS Biol 17(10):e3000426
Badre D, D’Esposito M (2009) Is the rostro-caudal axis of the frontal lobe hierarchical? Nat Rev Neurosci 10(9):659–669
Strange BA, Witter MP, Lein ES, Moser EI (2014) Functional organization of the hippocampal longitudinal axis. Nat Rev Neurosci 15(10):655–669
Smith EH, Liou JY, Merricks EM, Davis T, Thomson K, Greger B et al (2022) Human interictal epileptiform discharges are bidirectional traveling waves echoing ictal discharges. Elife 11
Smith EH, Liou JY, Davis TS, Merricks EM, Kellis SS, Weiss SA et al (2016) The ictal wavefront is the spatiotemporal source of discharges during spontaneous human seizures. Nat Commun 7:11098
Frauscher B, von Ellenrieder N, Zelmann R, Doležalová I, Minotti L, Olivier A et al (2018) Atlas of the normal intracranial electroencephalogram: neurophysiological awake activity in different cortical areas. Brain 141(4):1130–1144
Bhattacharya S, Donoghue JA, Mahnke M, Brincat SL, Brown EN, Miller EK (2022) Propofol anesthesia alters cortical traveling waves. J Cogn Neurosci 1–13
Hamid AA, Frank MJ, Moore CI (2021) Wave-like dopamine dynamics as a mechanism for spatiotemporal credit assignment. Cell 184(10):2733–2749.e16
Matityahu L, Gilin N, Atmna Y, Tiroshi L, Wickens JR, Goldberg JA (2022) Mechanism of dopamine traveling waves in the striatum: theory and experiment. bioRxiv
Ermentrout GB, Kleinfeld D (2001) Traveling electrical waves in cortex: insights from phase dynamics and speculation on a computational role. Neuron 29(1):33–44
Townsend RG, Gong P (2018) Detection and analysis of spatiotemporal patterns in brain activity. PLoS Comput Biol 14(12):e1006643
Download references
Acknowledgements
We thank Drs. Uma Mohan and Honghui Zhang for technical assistance. This research was supported by a RISE award and an NSF CAREER award to J.J.
Author information
Authors and affiliations.
Department of Biomedical Engineering, Columbia University, New York, NY, 10027, USA
Anup Das, Erfan Zabeh & Joshua Jacobs
Mortimer B. Zuckerman Mind Brain Behavior Institute, Columbia University, New York, NY, 10027, USA
Erfan Zabeh
Department of Neurological Surgery, Columbia University, New York, NY, 10027, USA
Joshua Jacobs
You can also search for this author in PubMed Google Scholar
Corresponding author
Correspondence to Anup Das .
Editor information
Editors and affiliations.
Ruhr University Bochum, Department of Neuropsychology, Institute of Cognitive Neuroscience, Faculty of Psychology, Bochum, Germany
Nikolai Axmacher
Ethics declarations
The authors declare no competing financial interests.
Rights and permissions
Reprints and permissions
Copyright information
© 2023 The Author(s), under exclusive license to Springer Nature Switzerland AG
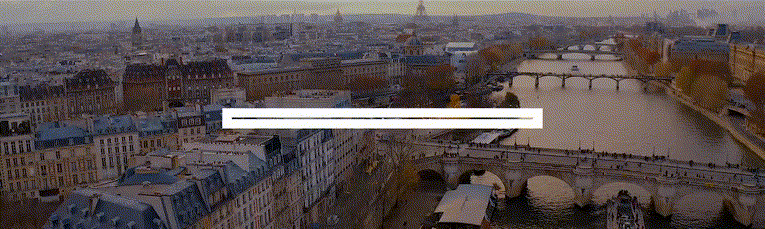
About this chapter
Das, A., Zabeh, E., Jacobs, J. (2023). How to Detect and Analyze Traveling Waves in Human Intracranial EEG Oscillations?. In: Axmacher, N. (eds) Intracranial EEG. Studies in Neuroscience, Psychology and Behavioral Economics. Springer, Cham. https://doi.org/10.1007/978-3-031-20910-9_30
Download citation
DOI : https://doi.org/10.1007/978-3-031-20910-9_30
Published : 02 August 2023
Publisher Name : Springer, Cham
Print ISBN : 978-3-031-20909-3
Online ISBN : 978-3-031-20910-9
eBook Packages : Behavioral Science and Psychology Behavioral Science and Psychology (R0)
Share this chapter
Anyone you share the following link with will be able to read this content:
Sorry, a shareable link is not currently available for this article.
Provided by the Springer Nature SharedIt content-sharing initiative
- Publish with us
Policies and ethics
- Find a journal
- Track your research
EU plans post-Brexit free movement deal for young Britons
T he European Commission has proposed restoring much of the pre- Brexit freedom that allows British travellers aged 18 to 30 the opportunity to travel, work or study for up to four years in the EU.
One of the most-mourned European freedoms that the UK chose to remove with Brexit was the opportunity for young Brits to travel, study and work in any EU country without restriction .
Boris Johnson’s government successfully negotiated for UK citizens to become “third-country nationals”, on a par with Venezuelans. As a result of the Brexit deal, British passport holders can stay a maximum of 90 days in the European Union and wider Schengen area – and must then leave for at least a further 90 days.
Post-Brexit work or study in continental Europe is possible only after fulfilling complex visa requirements.
“Beneficiaries should be able to undertake different activities during that period, such as studying, training, working or travelling,” says the commission in a briefing document .
The European Commission adds: “The UK has shown interest in the issue by reaching out to a number of member states on youth mobility.”
Under the proposals, young EU citizens would have a reciprocal opportunity to spend time in the UK .
Maros Sefcovic, executive vice-president responsible for inter-institutional relations, said: “The United Kingdom’s withdrawal from the European Union has hit young people in the EU and the UK who would like to study, work and live abroad particularly hard.
“Today, we take the first step towards an ambitious but realistic agreement between the EU and the UK that would fix this issue. Our aim is to rebuild human bridges between young Europeans on both sides of the Channel.”
The proposed requirements look simple, comprising “a valid travel document, valid comprehensive sickness insurance, proof of sufficient means of subsistence”. But applications could be rejected if the individual is believed to pose a threat to “public policy, public security or public health”.
Young people would pay for a visa or residence permit; the European Commission says this “should not be disproportionate or excessive”.
Technically, the commission is merely recommending the move to the European Council, comprising the leaders of EU member states. But it is likely to be rubber-stamped.
The question is: how will Rishi Sunak’s government respond to the offer? Ministers have already backtracked on some of the hardline rules introduced with Brexit , such as a complete ban on EU children on school trips using identity cards.
Any such deal would be welcomed by the UK hospitality industry, which has struggled with recruitment since Brexit.
But with immigration high on the Conservatives’ election agenda, the government may not wish to confer freedom on young Europeans.
The Independent has asked the Home Office for a response.
Abta, the travel association, welcomed the move from Brussels. Luke Petherbridge, director of public affairs for the association, said: “We strongly welcome today’s announcement and urge the UK government to be ready to begin negotiations.
“Post-Brexit restrictions on UK-EU labour mobility have undoubtedly been detrimental to the UK travel and tourism industry, whether outbound, inbound or domestic.
“The impacts are being most keenly felt by young people, who are now less able to access the roles that have previously proven a springboard for successful careers, as well as providing enriching and rewarding experiences.
“Abta has long argued that youth mobility should be a priority for both sides as we seek to rebuild relations post-Brexit, and we hope that negotiations can now begin quickly.”
The Independent is the world’s most free-thinking news brand, providing global news, commentary and analysis for the independently-minded. We have grown a huge, global readership of independently minded individuals, who value our trusted voice and commitment to positive change. Our mission, making change happen, has never been as important as it is today.
Thank you for visiting nature.com. You are using a browser version with limited support for CSS. To obtain the best experience, we recommend you use a more up to date browser (or turn off compatibility mode in Internet Explorer). In the meantime, to ensure continued support, we are displaying the site without styles and JavaScript.
- View all journals
- My Account Login
- Explore content
- About the journal
- Publish with us
- Sign up for alerts
- Open access
- Published: 18 October 2021
Spontaneous traveling waves naturally emerge from horizontal fiber time delays and travel through locally asynchronous-irregular states
- Zachary W. Davis ORCID: orcid.org/0000-0003-4440-9011 1 na1 ,
- Gabriel B. Benigno 2 , 3 na1 ,
- Charlee Fletterman 1 na1 ,
- Theo Desbordes 1 ,
- Christopher Steward 3 ,
- Terrence J. Sejnowski ORCID: orcid.org/0000-0002-0622-7391 1 na2 ,
- John H. Reynolds ORCID: orcid.org/0000-0001-6988-4607 1 na2 &
- Lyle Muller ORCID: orcid.org/0000-0001-5165-9890 2 , 3 na2
Nature Communications volume 12 , Article number: 6057 ( 2021 ) Cite this article
7572 Accesses
17 Citations
27 Altmetric
Metrics details
- Extrastriate cortex
- Network models
- Sensory processing
Studies of sensory-evoked neuronal responses often focus on mean spike rates, with fluctuations treated as internally-generated noise. However, fluctuations of spontaneous activity, often organized as traveling waves, shape stimulus-evoked responses and perceptual sensitivity. The mechanisms underlying these waves are unknown. Further, it is unclear whether waves are consistent with the low rate and weakly correlated “asynchronous-irregular” dynamics observed in cortical recordings. Here, we describe a large-scale computational model with topographically-organized connectivity and conduction delays relevant to biological scales. We find that spontaneous traveling waves are a general property of these networks. The traveling waves that occur in the model are sparse, with only a small fraction of neurons participating in any individual wave. Consequently, they do not induce measurable spike correlations and remain consistent with locally asynchronous irregular states. Further, by modulating local network state, they can shape responses to incoming inputs as observed in vivo.
Similar content being viewed by others
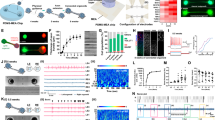
Complex activity and short-term plasticity of human cerebral organoids reciprocally connected with axons
Tatsuya Osaki, Tomoya Duenki, … Yoshiho Ikeuchi
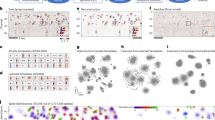
Spike sorting with Kilosort4
Marius Pachitariu, Shashwat Sridhar, … Carsen Stringer
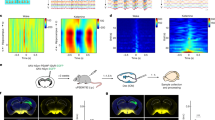
Neuronal dynamics direct cerebrospinal fluid perfusion and brain clearance
Li-Feng Jiang-Xie, Antoine Drieu, … Jonathan Kipnis
Introduction
Visual cortical neurons exhibit variable fluctuations in their spontaneous activity and stimulus-evoked responses. Rather than being due to noise intrinsic to the neural spiking mechanism 1 , which is highly reliable 2 , variability is thought to emerge from ongoing synaptic activity in the dense recurrent connectivity of cortical networks 3 , 4 . When observed from a single point in the cortex, spontaneous fluctuations resemble a broadband temporal noise process 4 , 5 . Multisite recordings have revealed that these temporal fluctuations can be part of waves traveling across a cortical area 6 , 7 , 8 , 9 , 10 . Spontaneous traveling waves had largely been observed in slow-wave fluctuations associated with anesthesia, sleep, or low arousal 11 , 12 , 13 . While traveling waves had been theorized to have an impact on cortical computation, it was difficult to identify their role since active cortical states exhibit fluctuations that are more complex, dominated by higher-frequency and lower-amplitude activity 14 , making these waves harder to detect. Further, driving input is believed to quench variability in ongoing dynamics 15 , calling into question the potential impact of traveling waves on evoked activity 16 .
Recent work has shown that spontaneous traveling waves are present in the awake state, that they influence the magnitude of sensory-evoked activity, and that—depending on their retinotopic alignment with sensory input—they can improve perceptual sensitivity 17 . However, the mechanisms that generate them, and whether they are consistent with the asynchronous-irregular spiking dynamics characteristic of awake cortex 18 , are unknown. Based on their speed of propagation, we hypothesize that these waves result from action potentials propagating along unmyelinated horizontal fibers. To test this hypothesis, we studied a spiking network model across a range of biologically realistic neuronal densities, distance-dependent connection probabilities, excitatory/inhibitory balances, and synaptic conductance states. Importantly, this model incorporated axonal time delays from conduction along unmyelinated horizontal fibers, which shaped ongoing activity patterns into traveling waves consistent with those observed in vivo. Spontaneous traveling waves were apparent in this network model and occurred consistently across a wide parameter range that produced asynchronous-irregular dynamics.
One might wonder whether the occurrence of these traveling waves induces correlated variability, which has been found to impair perception 19 . Results from the spiking network model show this need not be the case. In both the computational model and multielectrode recordings in the marmoset visual system, we found the change in spiking probability due to the wave was low, only sparsely modulating spiking activity. We thus refer to the model as the sparse-wave model and this regime as the sparse-wave regime. This is in contrast to smaller-scale network models where spikes are strongly coupled to the state of traveling waves, producing strong correlations in spiking activity. Rather, at the scale of entire cortical areas, spontaneous waves can emerge in spatially structured shifts in spiking probability and propagate through sparse spiking activity along horizontal fibers, without inducing changes in pairwise correlations in the activity of individual neurons. Traveling waves can thus coexist with a locally asynchronous-irregular state, conferring their benefits while maintaining the computational advantages of this dynamical regime 20 , 21 .
Spontaneous synaptic fluctuations are comparable to those during stimulus-evoked responses
Previous work has shown that moment-by-moment fluctuations in synaptic input in the cortex can be on the same order of magnitude as during the sustained period of stimulus-evoked responses 6 , 22 , 23 , 24 . Fluctuating synaptic inputs can have a significant impact on neural excitability 25 , gain modulation 26 , and readout of sensory information 7 . To understand the impact of the spontaneous network state on evoked responses in the awake visual cortex, we recorded spontaneous and stimulus-evoked activity from chronically implanted multielectrode Utah arrays (Blackrock Microsystems) in area MT of two common marmosets ( Callithrix jacchus ; data previously reported by Davis et al. 17 ). Spontaneous multiunit activity recorded from a single electrode while a marmoset fixated a fixation point was characterized by a low, irregular firing rate. The appearance of a highly salient stimulus (10% Michelson contrast drifting Gabor) within the multiunit receptive field evoked a robust response (Fig. 1a ). When measured over many repeated presentations of the stimulus, the mean multiunit firing rate rose from 13 ± 1.6 sp/s during fixation, to 97 ± 5.7 sp/s in response to the stimulus ( N = 40 trials over three recording sessions). These evoked spiking responses were variable from trial to trial (mean fano factor = 1.01 ± 0.01 SEM, 40–240 ms after stimulus onset), consistent with previous observations 15 , 27 , 28 .
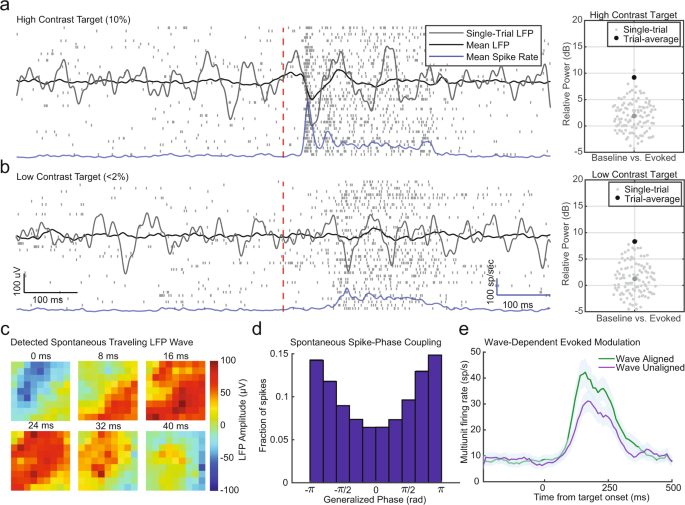
a Spike raster for repeated presentations ( N = 40) of a high-contrast (10% Michelson contrast) drifting Gabor recorded from area MT of a fixating marmoset (stimulus-onset, red line; mean response, blue line). A single-trial LFP trace is plotted in gray, and the average LFP response is plotted in black. The relative power between baseline (−200 ms to stimulus-onset) and evoked fluctuations (stimulus-onset + 50–250 ms) significantly favored the evoked response (right panel; N = 110 trials; median = 1.89 dB, p = 0.000019, two-tailed Wilcoxon’s ranked-sum test). b Same as in ( a ), but for a low contrast stimulus (<2% Michelson contrast). The relative power between baseline and evoked LFP fluctuations was not statistically different from parity (median = 1.23 dB, p = 0.087 two-tailed Wilcoxon’s ranked-sum test). c An example of spontaneous LFP fluctuations structured as a traveling wave recorded from a spatially distributed multielectrode array in marmoset area MT. d Histogram of spontaneous spike probability as a function of the generalized phase of the LFP during fixation. e The average evoked response to low contrast stimuli was stronger when a more excitable phase (± π rad) of a spontaneous traveling wave aligned with the retinotopic location of the target (aligned, green line) as compared to when a less excitable phase (0 rad) was aligned (unaligned, purple line; N = 43 wave and non-wave trials; shaded region SEM; p = 0.0000015 two-tailed Wilcoxon’s rank-sum test). Data for panels c – e modified from Davis et al. 17 with permission.
This variability is partly the result of ongoing spontaneous fluctuations in synaptic activity in the local population at the time of the evoked spiking response 6 , 7 . These fluctuating synaptic inputs, in turn, contribute to the local field potential (LFP) 29 , 30 . When averaged across high-contrast trials, the LFP had a robust negative deflection aligned to the stimulus-evoked spiking response, while the pre-stimulus period was flat (black line, Fig. 1a ). However, at the single-trial level, the stimulus-evoked LFP response was similar in magnitude to the spontaneous fluctuations occurring during fixation (right panel, Fig. 1a ). The relative power between the LFP just prior to the stimulus (−200 to 0 ms) and following stimulus onset (+50 to +250 ms) across single trials had a small but significant difference from 0 dB, which represents parity between spontaneous and stimulus-evoked fluctuations (median 1.89 dB, p = 0.00005 two-tailed Wilcoxon’s rank-sum test).
While strong, high-contrast visual stimulation evoked slightly stronger LFP fluctuations than intrinsic network fluctuations, the distinction disappears in the context of weak visual inputs (Fig. 1b ). When the marmoset was presented a faint stimulus that was detected ~50% of the time (<2% Michelson contrast), the evoked spiking response was significantly weaker and more variable (mean = 68 ± 4.4 sp/s, p = 0.0009; fano factor = 1.54 ± 0.14, p = 0.002, two-tailed Wilcoxon’s rank-sum test). This corresponded with a weaker average LFP response, and the trial-by-trial relative power between spontaneous and evoked fluctuations was not significantly different from 0 dB (median = 1.23 dB, p = 0.07 two-tailed Wilcoxon’s rank-sum test).
Given the comparable magnitude of spontaneous LFP fluctuations to responses evoked by weak sensory inputs, we hypothesized that much of the variability in neuronal spiking could be explained by the state of the local network since the synaptic drive (manifested in the LFP) during spontaneous and evoked activity is roughly equal 22 , 23 . We recently reported that spontaneous LFP fluctuations in the awake cortex are organized into waves that travel across an entire cortical area (Fig. 1c and Supplemental Movie S1 ). They modulate spontaneous spiking probability (Fig. 1d ), and they directly impact the magnitude of stimulus-evoked responses depending on their alignment with neuronal receptive fields (Fig. 1e ). We found that, rather than acting as a source of noise that impairs perception, spontaneous waves can—depending on their spatiotemporal alignment with a visual stimulus—improve the monkeys’ ability to detect the stimulus. We thus sought to understand what mechanisms might generate traveling waves in the cortex and test whether they represent an operating regime either consistent with or distinct from the irregular, asynchronous activity patterns classically observed in silico 31 , 32 and in vivo 21 , 33 .
Spontaneous traveling waves can emerge in network models without altering individual neuron spiking statistics
To address this question, we studied large-scale spiking network models composed of leaky integrate-and-fire (LIF) neurons with balanced excitation and inhibition and conductance-based synapses. When neuronal interactions are modeled as conductances, taking into account the time-dependent driving forces and channel activations at the synapse, spiking network models can enter into states of self-sustained activity 34 , 35 . Asynchronous-irregular activity 32 in these self-sustained states, generated without external drive, results naturally from the recurrently generated fluctuations intrinsic to the dynamics of the system 34 , 35 . These dynamics are characterized by low, variable firing rates, weak pairwise correlations, and coefficient of variation (CV) near unity. These self-sustained states provide an opportunity to study spiking network dynamics that are structured by the recurrent activity of the network itself, rather than dominated by random external Poisson synaptic input 20 , and are well suited to model the spontaneous background activity observed in the cortex during active perception.
We first studied a two-dimensional (2D) conductance-based spiking network model with over 1,000,000 neurons distributed over a 6 × 6 mm 2 area consisting of 80% excitatory and 20% inhibitory neurons, randomly connected with 3000 synapses per cell, yielding a sparsely connected network (Fig. 2a ). We eliminated the outer millimeter from analysis, yielding a 4 × 4 mm 2 area with 450,000 neurons. These values were selected to approximate the density and connectivity of neurons in cortical layer 2/3 of area MT in the common marmoset 36 , 37 . This randomly connected network generated self-sustained activity with spontaneous spiking fluctuations consistent with the asynchronous-irregular regime 32 , 38 and lacked any spatiotemporal structure (Fig. 2b, c ). A simulated LFP was calculated from summed excitatory and inhibitory synaptic activity over adjacent, nonoverlapping pools of 10 × 10 neurons (corresponding to 67.8 × 67.8 μm 2 ) 39 and was used to estimate the local excitability state at each point in the network for comparison to the electrophysiological recordings. The LFP was homogeneous across the network, as would be expected from pools of neurons receiving synaptic input from random positions in the network (Fig. 2c and Supplemental Movie S2 ).
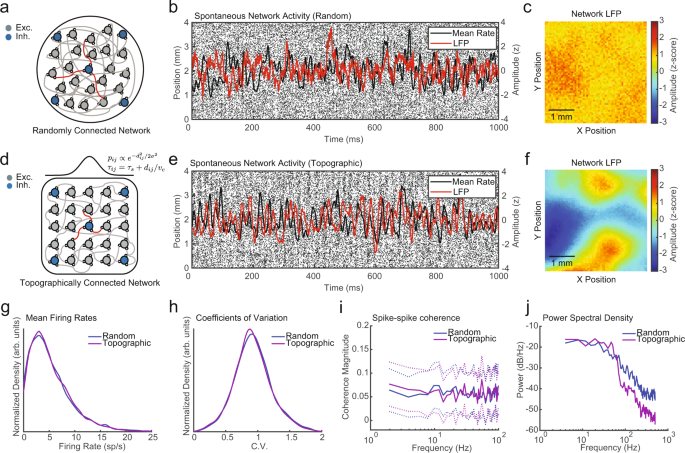
a Schematic diagram of a 2D spiking network model with 80% excitatory (gray) and 20% inhibitory (blue) neurons wired with a uniformly random connection probability. b Spike rasters from 10,000 excitatory neurons along a 1D slice arranged by linear distance in the network. LFP fluctuations calculated from summed synaptic currents for a single 10 × 10 neuron pool is plotted in red. The mean spike rate within one neuron pool is shown in black. c Spatial organization of LFP amplitude for each neuron pool in the network plotted at one time point. d Network schematic as in ( a ), but the network was topographically connected with probabilities drawn from a Gaussian ( σ = 400 µm), and activity had a distance-dependent transmission delay (0.2 m/s). e Spike rasters as in ( b ), but sparse structured fluctuations were apparent across the network. f Spatial LFP amplitude as in ( c ), but the LFP was heterogeneous across the network with topographic structure. g The distribution of single-unit mean firing rates did not differ between the random (blue line) and topographic networks (purple line; N = 5000 neurons; p = 0.28, two-tailed Wilcoxon’s rank-sum test). h The distribution of single-unit CV did not differ between the random and topographic networks ( p = 0.11). i Pairwise spike coherence did not differ between the random (blue line) and topographic networks (purple line; N = 10 paired adjacent neuron pools; CI test, α = 0.05; dotted lines 95% CI). j Power spectral density for LFP from the random and topographically connected networks.
To test whether topographic connections with transmission delays were sufficient to generate spontaneous traveling waves in the network, as a refinement to the model described in Fig. 2a , two key elements were introduced: (1) connection probability decayed as a Gaussian with the distance between neurons ( σ = 400 µm) 40 , 41 to mimic the topographic connectivity in cortex and (2) action potentials activated synaptic currents after a time delay determined by the distance between neurons to simulate the conduction velocity of horizontal fibers in the cortex ( v c = 0.2 m/s; 42 Fig. 2d ). This network also produced self-sustained, spontaneous fluctuations, but spiking activity was weakly organized into bands that moved across the network as traveling waves (rasters, Fig. 2e ). LFP fluctuations were heterogeneous across the network and exhibited organized spatial structure with localized regions coordinated in amplitude (Fig. 2f and Supplemental Movie S3 ).
To test whether the presence of these organized topographic fluctuations altered the asynchronous-irregular dynamics of individual neurons in the network, we compared the firing rates and CV across a randomly selected population of excitatory neurons ( N = 5000). There was no difference in the distribution of firing rates across the networks (mean rate = 5.23 vs. 5.27 sp/s; p = 0.28, two-tailed Wilcoxon’s rank-sum test; Fig. 2g ) or in the distribution of CV (mean CV = 0.93 vs. 0.92; p = 0.11, two-tailed Wilcoxon rank-sum test; Fig. 2h ). Therefore, individual neurons maintained their asynchronous and irregular firing states while the topographically connected network produced spontaneous traveling waves.
While one might expect the organized bands of spiking activity would result in increased correlations across neurons, we found no evidence that this was the case. The introduction of topographic connections did not affect pairwise correlations, as the degree of spike–spike coherence between the randomly and topographically connected networks was indistinguishable (Fig. 2i ). No change in coherence occurred despite the topographically connected network producing increased power in lower frequencies (30–50 Hz) and reduced power in higher frequencies (>60 Hz) relative to the randomly connected network (Fig. 2j ). The spatiotemporal structure could, therefore, exist in these networks without disrupting CV or pairwise coherence because the spiking probability was only weakly modulated by the presence of traveling waves. The probability of a neuron firing a spike at any given millisecond was low, and the peak of a traveling wave only marginally increased spiking probability (2.33% increase), resulting in only a small fraction of neurons spiking during the peak of any given wave. We, therefore, refer to this as the “sparse-wave” network regime. If it were the case that neurons strongly participated in these fluctuations, then they would show a degree of coherence in the range of frequencies dominated by those fluctuations. To demonstrate this, we simulated a smaller network with fewer neurons and denser connections (model parameters Table S1 ), which generated spontaneous fluctuations that strongly regulated spiking activity. This “dense-wave” network did strongly modulate spiking activity during traveling waves (26.48% increase in spiking probability), which produced strong spike–spike coherence in the frequency band dominated by fluctuations in the LFP (Fig. S1 ). This increase in correlation was greatest for nearby locations in the network and was negatively correlated with distance (Pearson’s r = −0.72; Fig. S2 ). Thus, unlike in the dense-wave network, traveling waves in the sparse-wave regime do not necessarily induce pairwise correlation across the network.
Topographic connectivity and distance-dependent delays are necessary to generate spontaneous waves
As hypothesized, the addition of topographic connections and conduction delays was sufficient to produce clear spatiotemporal organization in the network activity (Fig. 3a ). In order to detect traveling waves, we utilized the property that activity patterns propagating at a fixed speed in the network will produce a band at a constant slope in the 2D space–time fast Fourier transform (FFT) 43 . Importantly, although the power spectral density at each point in the network had broad-spectral power (Fig. 2j ), the 2D space–time FFT revealed a clear spectral peak (Fig. 3b ), whose slope in relation to the temporal and spatial frequencies was dependent on the axonal conduction speed. To classify these activity patterns as traveling waves and quantify their properties relative to cortical recordings from the marmoset cortex, we applied the same analysis technique developed for the experimental recordings (generalized phase, GP 17 ) to the simulated LFP in each 10 × 10 neuronal pool. We then adapted a technique previously developed for detecting traveling waves in noisy multielectrode recordings 44 , 45 . We estimated the gradient of the phase at each moment in time and calculated putative wavelengths. We then identified places and times in the network where there was significant spatial organization. Significance was determined by comparing the observed wavelengths to the wavelength distribution after a spatial shuffle of electrode positions, with the 99th percentile of the shuffle distribution taken as the threshold criterion (Fig. 3c, d ). This approach provides a sensitive and robust means to detect traveling waves from moment to moment 44 , 45 . We found significant wave activity in the topographically connected network ~50% of the time, whereas the presence of significantly structured wave organization was absent from networks with random connections and no delays (Fig. 3e ) as the distribution of putative wavelengths was similar to the shuffled distribution (Fig. 3f ).
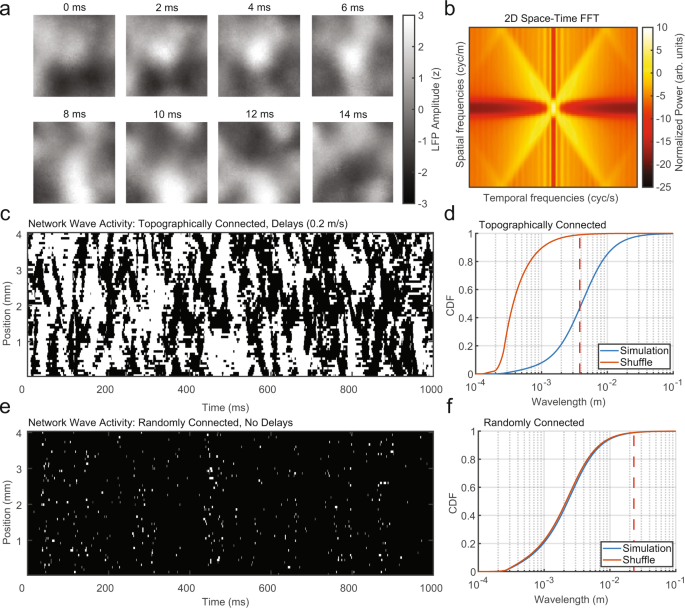
a Time series of simulated LFP activity from the topographically connected network in Fig. 2 . Regional peaks and troughs moved coherently across the network as traveling waves. b 2D (space–time) FFT reveals a concentration of spatiotemporal energy along temporal ( x -axis) and spatial ( y -axis) frequencies reflecting the flow of activity across the network. c The presence of significant wave activity for a linear slice through the large-scale 2D network model. Significant (white) wave values were defined as estimated wavelengths that exceeded the 99th percentile of the spatially shuffled wavelength distribution (48.53% of network activity). d Cumulative distribution functions (CDFs) of the observed wavelengths (blue) and wavelengths after spatially shuffling the LFP pool locations (red, 99th percentile, red dashed line). e The randomly connected network had few points that were classified as traveling waves (1.12% of network activity). f Wavelength CDFs as in ( d ) for the randomly connected network and its shuffle.
We also explored the sufficiency of topographic connections and conduction delays in generating waves separately (Fig. S3 ). A topographic network lacking transmission delays produced spatially organized activity, but there was no spectral line in the 2D FFT consistent with traveling waves (Fig. S3b ). Conversely, delays in an otherwise randomly connected network did not generate large-scale spatially organized activity, but did have a clear spectral line consistent with propagating activity (Fig. S3c ). From this, we conclude that, in our framework, topographic connectivity is necessary for the emergence of large-scale spatially organized activity, and transmission delays are necessary for the regular flow of activity over space and time. Both topography and delays together are necessary in our network framework to produce spatiotemporal dynamics that travel over the network consistent with the traveling waves we observed in our cortical recordings. These results were consistent in a simpler one-dimensional (1D) network model where the emergence of traveling waves required both topographic connections and transmission delays (Fig. S4 ).
Spontaneous waves occur throughout the asynchronous-irregular regime
In the example network, topographic connections with axonal conduction delays were sufficient to induce large-scale waves of activity without disrupting the fine-scale asynchronous-irregular dynamics of individual neurons. Does the presence of traveling waves generalize across all networks with asynchronous-irregular activity, topographic connections, and axonal conduction delays 35 , 38 ? We scanned across 2500 combinations of different excitatory and inhibitory (E/I) conductances in the topographically connected model and found 601 combinations that produced self-sustained spiking activity. We then identified networks with asynchronous-irregular spiking dynamics, defined as networks with mean excitatory firing rates between 1 and 25 sp/s and mean CV between 0.7 and 1.4 38 . Approximately 99% (599 out of 601) of the networks that generated self-sustained activity were classified as asynchronous irregular. We then measured the percentage of time each network’s activity was significantly organized into traveling waves. Waves were present across the entire range of asynchronous-irregular networks (Fig. 4a ). The strength of wave activity was negatively correlated with the magnitude of E/I conductance (Pearson’s r = −0.55 ± 0.002, 95% confidence interval (CI)) indicating weaker synapses led to stronger wave activity. The average wavelength was positively correlated with synaptic strength (Pearson’s r = 0.72 ± 0.001; Fig. 4b ), indicating stronger synaptic weights lead to more synchronous network dynamics. These results demonstrate that spontaneous traveling waves are a general property of topographic connectivity and are entirely consistent with locally asynchronous-irregular states.
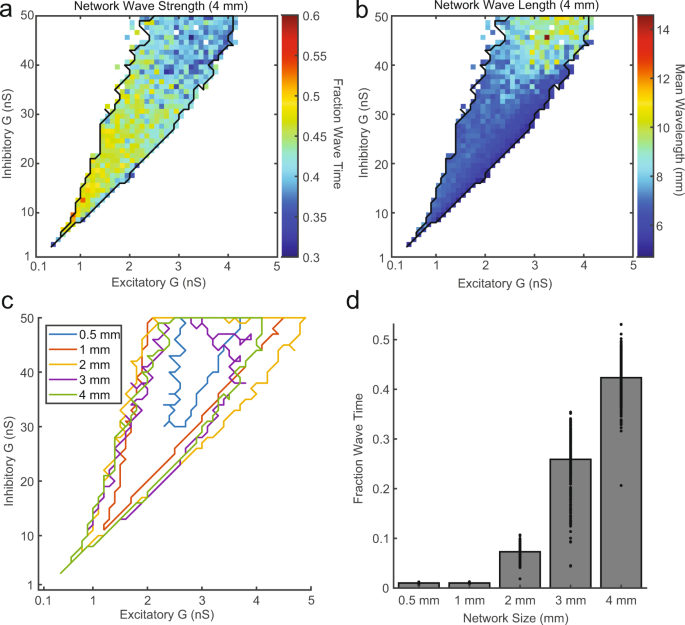
a The percentage of wave-like activity across ranges of excitatory and inhibitory conductances for 4 mm width topographic network simulations. Each pixel is one simulation of a network as described in Fig. 2d , but with the excitatory and inhibitory conductances corresponding to its x and y coordinate, respectively. Networks consistent with asynchronous-irregular spiking dynamics fall within the black border. White pixels are networks that did not self-sustain or had extremely low/high mean firing rates (FR < 1 or FR > 25 sp/s). b Same as ( a ), but the mean of the wavelength distribution is plotted. c The region of the parameter space that exhibited asynchronous-irregular activity grew to include smaller synaptic conductances with the size of the network (width 0.5 mm to 4 mm). d The fraction of wave activity present across all self-sustained and asynchronous-irregular networks grew with the size of the simulated network ( N = 163, 532, 752, 540, and 599 stable A–I simulations for sizes 0.5, 1, 2, 3, and 4 mm respectively).
How important is network scale in generating traveling waves? To answer this question, we simulated networks ranging from 0.5 to 4 mm in width, holding neuronal and connection density constant. For small networks (~0.5 mm), a very limited range of the E/I space produced self-sustained and asynchronous network dynamics. As network size grew, the asynchronous-irregular parameter space grew as well, extending to include smaller and smaller combinations of E/I synaptic strength 18 (Fig. 4c ). It was thus necessary to simulate spiking network models at sufficient spatial scales (>1 mm) to generate asynchronous-irregular activity in networks with conductances resembling those estimated in vivo 18 . At small network scales, wavelength distributions during asynchronous-irregular dynamics were not distinct from the spatial shuffle, and the parameters that favored longer wavelengths did not produce asynchronous-irregular activity. Only at larger network scales did wave activity become strongly apparent (Fig. 4d ).
Network connectivity determines wave properties
What effect did our chosen parameters for connection distance and conduction velocity have on wave properties? We hypothesized the spatial extent of connections and the conduction speed of spikes directly control the wavelength and propagation speed, respectively, of traveling waves in the model. To test these predictions, we simulated networks with various values of standard deviation ( σ s ) for the Gaussian connection probability distribution. Consistent with our hypotheses, the distribution of significant wavelengths increased with larger connection distances (Fig. 5a and Supplemental Movies S4 and S5 ), and increasing the conduction velocity created a corresponding increase in the propagation speed reflected in the slope of the spectral line in the space–time FFT (Fig. 5b ). Thus, the macroscopic features of spontaneous traveling waves were directly related to specific network structures in the model.
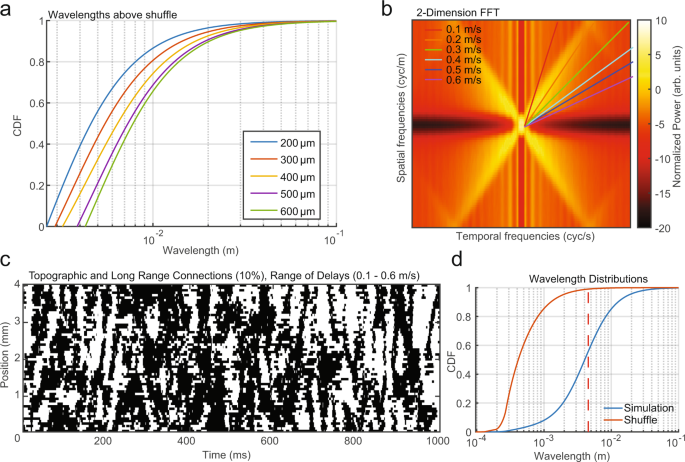
a Distribution of wavelengths exceeding the 99th percentile of a spatial shuffle for networks simulated across a range of Gaussian sigma values for the topographic connection probability. b 2D FFT for the topographic network with delays produced by 0.2 m/s conduction velocities. The colored lines match the slopes of the concentration of spatiotemporal energy across different simulations with conduction velocities ranging from 0.1 to 0.6 m/s. c Significant wavelengths (white pixels) were strongly present in a network with a mix of topographically (90%) and randomly (10%) connected projections and a range of conduction velocities (0.1–0.6 m/s). d CDFs for the simulation in ( c ) and its shuffle (blue and red, respectively).
Are these waves only possible with perfectly Gaussian connection profiles and uniform conduction velocity, or can they tolerate a broad range of values similar to those observed in vivo? To test this, we simulated the example model in Fig. 2 , with 10% of the connections randomly rewired with uniform probability across the network, generating long-range connections 46 , 47 . The conduction velocity along each connection was drawn from the range of conduction speeds observed for unmyelinated horizontal fibers in the cortex (0.1–0.6 m/s 42 , 48 , 49 ). Spontaneous traveling waves persisted under these network conditions (Fig. 5c, d ), indicating that the presence of waves was not limited to a fixed or limited set of homogeneous network properties, but instead also occurred in networks with large heterogeneity, as in the cortex.
Network simulations are consistent with traveling waves in vivo
How well do the dynamics observed in our simulations match the dynamics observed in electrophysiological recordings of the cortex? To test this, we compared the model results to the data recorded from marmoset MT, while monkeys fixated a spot at the center of an otherwise gray computer screen. We measured the mean firing rates (Fig. 6a ) and CV (Fig. 6b ) across the population of single- and multiunit activity over multiple recording sessions. The distributions of firing rates and CV were qualitatively similar between the recorded data and the sparse-wave model, suggesting that the spontaneous dynamics in the cortical recordings are also consistent with the asynchronous-irregular regime.
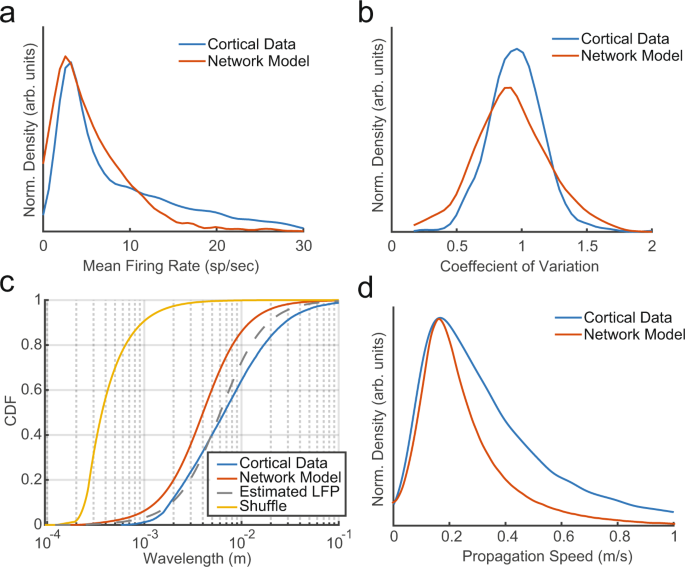
a Distribution of unit mean firing rates across the topographic network simulation in Fig. 2d (red) and the distribution observed across single- and multiunits recorded from area MT of awake, behaving marmosets (blue). b The distributions of CV for simulated (red) and recorded cortical data (blue). c CDFs of wavelengths for the simulation in Fig. 5c (red), its shuffle (yellow), and the observed distribution recorded in the cortex (blue). Simulated wavelengths were more similar to observed wavelengths in vivo after applying a smoothing kernel across space in the simulated network to approximate the estimated spatial integration of the LFP in electrophysiological recordings (250 µm, gray dashed line). d Wave speed distributions for the simulation in Fig. 5c (red), and recorded cortical data (blue) covered a range consistent with axonal conduction velocities (0.1–0.6 m/s).
We next measured the distribution of estimated wavelengths in the data and compared this to the wavelength distribution in the model. LFP data in the cortex are not independent across electrodes (as it is in our simulation), but rather pools signals from a cortical volume of ~250 μm in a radius around the electrode tip 29 , 30 and has correlations that fall off with distance 14 . To emulate these properties of cortical LFP recordings, we applied a smoothing kernel that expanded the area of integration from each simulated LFP point and reduced the independence of the signal. After smoothing our simulated LFP and quantifying wave properties, the distribution of simulated wavelengths closely approximated the distribution observed in the cortex (dotted gray and blue lines, Fig. 6c ). Similarly, the distribution of observed speeds in both the network simulation and the data covered the range of conduction velocities in the horizontal fibers, peaking at ~0.2 m/s (Fig. 6d ). Thus, across four different measures (spike rate, spike variability, wave size, wave velocity), the distributions characterizing activity in the network model were in close alignment with experimental recordings.
Neurons sparsely participate in waves due to weak coupling to synaptic fluctuations
How does activity in the sparse- and dense-wave networks affect the membrane potentials at the level of individual neurons? To answer this question, we studied the membrane potential distributions of individual neurons in each network. In the sparse-wave model, membrane potential fluctuations were Gaussian and close to the spiking threshold, consistent with the fluctuation-driven regime 50 (black line, Fig. 7a ). This was in contrast to the skewed distribution of membrane potentials in the dense-wave network, which was consistent with a synaptic drive to neurons that is clustered and strongly correlated 51 (purple line, Fig. 7a ).
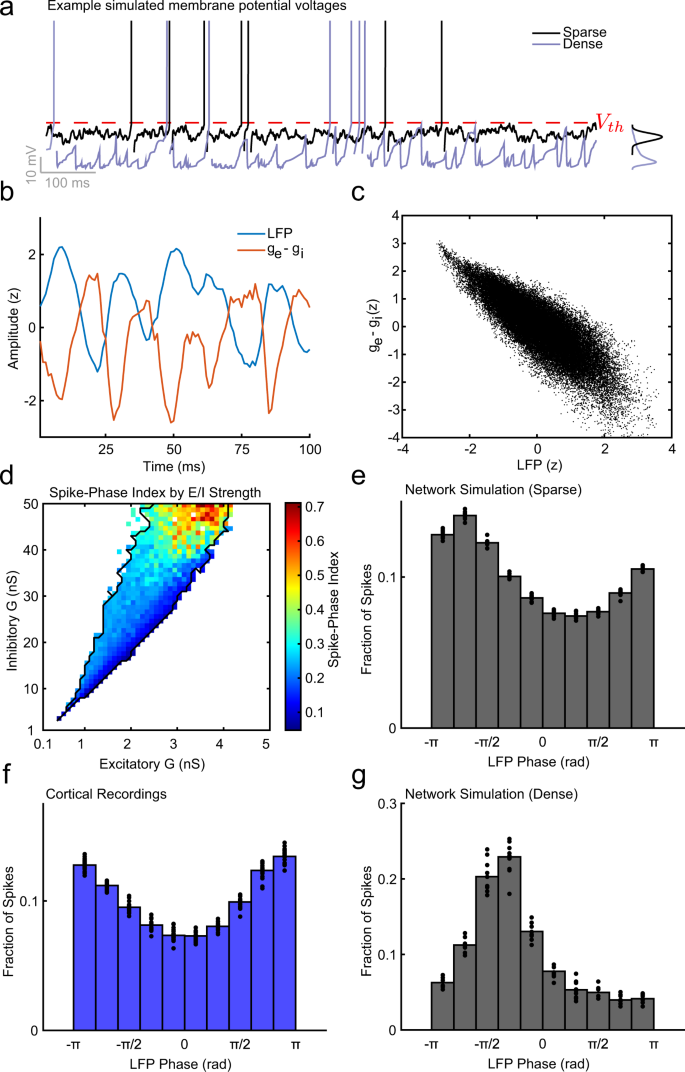
a Membrane voltage for a simulated neuron in either the sparse-wave network (black line) or dense-wave network (purple line) calculated from the summed excitatory and inhibitory synaptic currents received by that neuron. Spiking activity occurred when the voltage crosses the threshold ( V th red line). The distribution of membrane potentials over the interval for the sparse and dense networks is plotted on the right. b The amplitude of the simulated LFP (blue line) and the relative level of excitatory and inhibitory conductance (red line) over a 10 × 10 neuron pool were counter phase. c Scatter plot of LFP and g e − g i difference revealed a significant negative correlation ( N = 50,000 time points; Pearson’s r = −0.83; CI test, α = 0.01). d Spike-phase coupling was significant across networks in the asynchronous-irregular regime, and the degree of coupling was correlated with the magnitude of synaptic conductance ( N = 599 simulations; Pearson’s r = 0.78 ± 0.001, 95% CI). e Histogram showing the fraction of spikes that occurred during each phase of the simulated LFP in the topographically connected network. Spike probability was modulated by the LFP phase ( N = 22 resamples vs. shuffle; spike-phase index = 0.15). f Same as in ( e ), but for recorded cortical data. Spike probability was similarly modulated (spike-phase index = 0.16; N = 22 recording sessions vs. shuffle). g The dense-wave network simulation had a significantly stronger spike-phase relationship ( N = 10 resamples; spike-phase index = 0.44, p = 0.0000085, two-tailed Wilcoxon’s rank-sum test).
In the sparse-wave network model, stochastic fluctuations in the membrane potential produced sparse and irregular spiking activity. These fluctuations were driven by shifts in excitatory-inhibitory balance across the local population, which, due to the topographic network connections, were shared by adjacent populations and carried by spikes across horizontal connections. These summed currents in our estimate of the LFP reflect the total synaptic input in the local population, which exhibited a counter phase relationship with the relative E/I balance: the inhibition-dominated E/I regime produced positive LFP potentials, and the excitation-dominated E/I regime produced negative LFP potentials (Fig. 7b, c ). This leads to the mechanistic observation that when the conductances are high, the z -scored LFP is positive and the balance is dominated by the shunting effects of inhibition. When the conductances are low, the z -scored LFP is negative and the balance is shifted to excitation, producing more spiking activity. This relationship mechanistically accounts for the phase-dependent relationship of spiking to the LFP in our cortical recordings.
To demonstrate that simulated neurons are sparsely modulated by traveling waves, we measured the LFP phase at which each spike occurred (10 bins from − π to π ; Fig. 7d ) across network simulations with varying E/I synaptic conductances. The degree of spike-phase modulation was significant across the entire parameter space, with spikes more likely during phases closer to ± π . The magnitude of this modulation was correlated with the magnitude of E/I conductances (Pearson’s r = 0.78 ± 0.001, 95% CI; N = 599 simulations), with stronger synaptic weights driving stronger coupling of spiking activity to LFP fluctuations indicating denser and more synchronous spiking waves. This result highlights the importance of large-scale network simulations that can produce stable A–I spiking dynamics with weaker synaptic weights to see sparse modulations of spiking probability by traveling waves. We chose a point among these small conductance values (1 nS G e , 10 nS G i ; same values for the topographic network in Fig. 2 ), to compare the degree of coupling between the model and the cortical recordings. There was no difference between the magnitude of spike-phase modulation observed in the sparse-wave network model and the recorded data ( N = 22 matched resamplings; model spike-phase index = 0.15 ± 0.001 SEM; cortex spike-phase index = 0.16 ± 0.005; p = 0.18, two-tailed Wilcoxon’s rank-sum test), although the preferred phase-angle differed slightly between the data and model (data-preferred phase = 3.05 rad, model-preferred phase = −2.27 rad).
While there was a similar degree of spike-phase modulation between the cortical data and the sparse-wave model (Fig. 7e, f) , the modulation was significantly stronger in the dense-wave model ( N = 10 resamples; spike-phase index = 0.44 ± 0.01, p = 0.000085, two-tailed Wilcoxon’s rank-sum test; Fig. 7g ). The phase distribution also differed strongly in peak phase angle (dense-preferred phase = −1.11 rad). In addition, the randomly connected network showed no spike-phase relationship ( N = 22 resamples; spike-phase index = 0.03 ± 0.001 SEM, Fig. S5 ), as expected from a network where the neurons in the LFP pool draw from inputs distributed throughout the entire network. These results demonstrate that—in the simulated large-scale spiking networks—spatiotemporal organization emerges from weak modulations of spiking probability that produces sparse, phase-modulated spiking activity traveling along topographically distributed horizontal fibers. The presence of a similar spike-phase relationship in vivo, particularly for model conductance states that corresponded to experimental estimates of neuronal conductance states 18 , demonstrates that the sparse-wave regime in the model is consistent with the properties of waves observed in the experimental recordings.
Spontaneous traveling waves modulate responses to inputs
Finally, we hypothesized the state of network fluctuations in the sparse-wave network model would modulate the magnitude of responses evoked by feed-forward inputs, as previously studied for synaptic noise 4 , 26 and contextual gain control by visual stimuli 52 , 53 , 54 , 55 . To test this, we stimulated one 10 × 10 neuron pool in the sparse-wave network with a 20 Hz Poisson spike train on 100 afferent synapses to each neuron to mimic feed-forward stimulus input to the network. We stimulated for 10 ms, aligned either to the depolarized or hyperpolarized state of network fluctuations defined, respectively, by the most and least probable phases for spikes to occur according to the network’s spontaneous spike-phase distribution. When spiking inputs were aligned to the depolarized state, the evoked spiking responses were boosted (blue lines, Fig. 8a ) relative to weaker responses when inputs were aligned to the hyperpolarized state (red lines, Fig. 8a ). These effects were consistent with wave-modulated visual responses to motion stimuli observed in area MT in vivo (cf. Fig. 1e ). In contrast, equal stimulation in either state of the dense-wave network produced little effect (red and blue lines, Fig. 8b ).
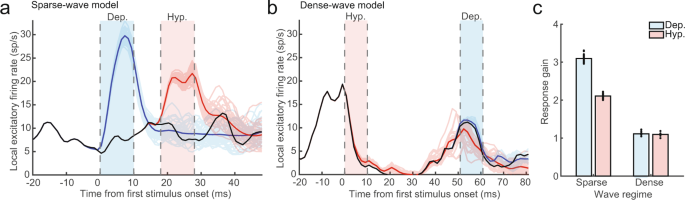
a A 0.2 × 0.2 mm 2 pool in the sparse-wave network model received a 20 Hz Poisson spike train input for 10 ms aligned either to a period of depolarization (blue shaded region) or hyperpolarization (red shaded region) as defined by the spike-LFP phase relationship. The dark blue and red lines are the mean evoked firing rate after receiving the spiking input in either the depolarized or hyperpolarized phase respectively (light blue and red lines represent N = 40 individual trials). The black line is the firing rate of the neuron pool when no input was given. b Same as ( a ), but the inputs were delivered to the dense-wave network. The evoked responses were much weaker as the network shunted the currents evoked by the incoming spikes. c The response gain between the distributions of spontaneous and evoked activity across N = 40 presentations of spiking input. In the sparse-wave network (left bars), inputs during the depolarized state had larger relative responses as compared to inputs during the hyperpolarized state (3.09 ± 0.09 compared to 2.11 ± 0.05 mean ± standard deviation; p = 3.57 × 10 −8 , two-tailed Wilcoxon’s signed-rank test). In contrast, the dense-wave network (right bars) responses did not differ in their response gain during the hyperpolarized and depolarized states (1.10 ± 0.04 and 1.11 ± 0.05, respectively; p = 0.20).
To quantify the effect of the traveling waves on evoked responses in each network, we then calculated the gain modulation, which is the ratio of firing rates during the stimulus-evoked response divided by that of the no-stimulus case (Fig. 8c ). Across repeated input stimulations, input gain was significantly stronger for the depolarized state relative to the hyperpolarized state in the sparse-wave network ( N = 40 stimulations; depolarized state = 3.09 ± 0.09; hyperpolarized state = 2.11 ± 0.05, mean ± standard deviation; p = 3.57 × 10 −8 , two-tailed Wilcoxon’s signed-rank test). In contrast, in the dense-wave network, the strong spontaneous fluctuations shunted the incoming spikes, resulting in very weak evoked responses that did not significantly differ depending on network state (depolarized state = 1.11 ± 0.05; hyperpolarized state = 1.10 ± 0.04; p = 0.20; two-tailed Wilcoxon’s signed-rank test). The increase in gain that occurs in the sparse-wave network mirrors our observations of wave-dependent sensitivity in awake monkeys performing a threshold detection task 17 . Thus, the sparse-wave model offers a mechanistic account for observed phase-dependent modulations of weak sensory responses by traveling waves measured in vivo that a network characterized by dense-wave dynamics fails to replicate.
The present work builds on and seeks to explain our recent finding that spontaneous fluctuations in cortical activity modulate the moment-to-moment processing of sensory information in a manner that affects perceptual sensitivity. These fluctuations are neither synchronous across the cortical surface nor independent noise processes. Rather, they are often structured as traveling waves. The model presented here shows that distance-dependent conduction delays in topographic, conductance-based spiking network models are sufficient to account for our results in vivo. Waves occur spontaneously, without requiring a driving input, and they occur robustly, in the sense that they are generated across a wide parameter space and in the sense that they occur across the entire space of E/I conductances that gives rise to asynchronous-irregular activity dynamics. The properties of these waves depend systematically on the scales of distance-dependent connections and the speeds of action-potential propagation. The waves were well-matched to those observed in cortical recordings from behaving marmosets 17 for speeds consistent with the conduction velocity of unmyelinated horizontal fibers. Neurons sparsely participated in these waves at the scales of neuronal density and connectivity found in the cortex. The spiking sparseness of the waves allowed them to occur without disturbing the asynchronous-irregular dynamics that are observed in cortical activity and have advantages for neural computation 3 , 20 , 21 , 56 , 57 . These sparse-wave networks remain sensitive to spiking inputs, producing evoked responses modulated in a phase-dependent manner, as observed in vivo. This is in contrast to smaller-scale networks that exhibit dense waves that drive correlated fluctuations across the population and render the network insensitive to spiking inputs.
These results demonstrate the importance of considering distance-dependent time delays in neural systems. When considered at the scales of entire cortical areas, individual horizontal fibers can span distances ranging from hundreds of microns to several millimeters 46 , 47 , with axonal conduction delays on the order of tens of milliseconds 42 , 48 . While previous spiking network models that considered relatively smaller spatial scales (from 100 µm to 1 mm of cortex) held that the contribution of axonal delays was negligible in their effects on the temporal properties of spiking networks 58 , other studies have found fixed delays can have profound effects 59 , 60 , 61 , 62 , 63 , 64 , 65 , 66 , 67 . The effects of fixed and distance-dependent delays have been extensively studied in neural field equations 68 , 69 , 70 , 71 , 72 , 73 , 74 , 75 , 76 , 77 ; however, in these averaged population models the connection between single-unit and population activity is difficult to study because single-unit information is lost. Finally, traveling waves have been described in smaller-scale topographic spiking network models that lack distance-dependent delays, but these networks only produced dense waves of strongly correlated spiking activity 78 , 79 , 80 . In this work, our large-scale spiking model provides the insight that distance-dependent delays on scales relevant to a large extent of a visual region in the cortex provide a fundamental mechanism to shape spontaneous activity into waves throughout the state of balanced excitation and inhibition. Further, instead of being inconsistent with asynchronous-irregular states, as with previous models of traveling waves in spiking networks, spontaneous waves can travel across these large-scale spiking networks while local networks remain locally asynchronous. Thus, not only are spontaneous traveling waves consistent with the asynchronous-irregular regime, they are a necessary consequence of topographic connectivity and distance-dependent delays in cortex across conditions that yield asynchronous-irregular spiking.
Critically, the waves we observe sparsely modulate the background spiking probability of neurons in the network, allowing them to maintain locally asynchronous-irregular dynamics. These sparse-wave dynamics only become apparent when networks are modeled at sufficient scales, in particular hundreds of thousands of neurons over an area a few millimeters across. Our custom software implementation allowed for the simulation of networks with ~100,000 to 1,000,000 neurons, each with 3000 outgoing synapses per cell, addressing in the largest networks over 60 GB of RAM. Importantly, while the cells in our network models have a large number of synapses per cell, the number of possible connection partners is high such that the network connectivity remains sparse. In these networks, the large number of synapses per cell allows the network to achieve self-sustained asynchronous-irregular activity when synaptic conductances are on the order observed in cortex 35 , 81 . Importantly, the sparse waves we observe here may be related to the concept of sparse synchrony 82 , 83 , 84 , which has been shown to facilitate information transfer across areas during narrowband oscillations. Sparse waves may reflect this principle unfolding over both space and time, while also being consistent with the more generally occurring broad-spectral fluctuations during spontaneous awake activity in vivo.
Our findings that traveling waves need not induce pairwise correlations may at first appear to differ from recent work by Huang et al. (2019), in which traveling waves emerged from different spatial and temporal scales of excitation and inhibition and drove shared variability in ongoing dynamics. We do not view these findings as mutually exclusive. The work of Huang et al. offers a mechanistic explanation for a source of shared variability that occurs particularly in low-frequency fluctuations in the sensory cortex 85 . This shared variability has been shown in theoretical 86 , 87 , 88 and experimental studies 19 to have deleterious effects on sensory processing and has been observed experimentally to be reduced by attention 85 , 89 . In contrast, our model does not generate strong low-frequency dynamics, but instead seeks to describe traveling waves that occupy higher-frequency ranges (above 10 Hz) that our model suggests propagate through horizontal connectivity. Separate mechanisms could underlie the generation of low-frequency correlated variability and higher-frequency traveling waves, the latter of which have recently been shown to have phase-dependent benefits for visual detection 17 . One critical difference between the two models, however, is their relative scale, leaving open the question as to whether the differences in spiking correlation are due to fundamental mechanisms or network size. Additional research is necessary to understand how such mechanisms may interact in large-scale network models to better recapitulate the broader space of cortical dynamics observed in vivo.
Unlike the outsized conductances typically used in smaller network simulations, the large-scale networks simulated here enabled us to incorporate E/I synaptic strengths that were similar to those observed experimentally, leading to total E/I conductances on the order of the leak conductance 18 , 90 . This is advantageous because the larger conductances needed in smaller networks yield unrealistic coupling of spiking activity to synaptic fluctuations and shunt driving inputs 91 , as illustrated in the dense-wave model. By scaling our model to realistic neuronal densities on a spatial scale over several millimeters of the cortex (450,000 neurons over 16 mm 2 in the case studied here), the sparse-wave model sustains irregular activity, with strengths of individual synaptic inputs down to 0.5 and 4 nS for excitatory connections and inhibitory connections respectively. At these spatial scales and synaptic conductances, waves are present about 50% of the time, similar to what we find in the neocortex, and the wavelengths closely approximate those we find in vivo.
It is important to recognize that, while our network model of a cortical sheet generates self-sustained activity intrinsically, cortical circuits in vivo are driven by inputs from other cortical areas and subcortical structures, particularly the thalamus. Thalamic inactivation has been shown to severely attenuate the spontaneous firing rates of cortical neurons 92 , 93 , raising the question as to whether spontaneous traveling waves in vivo also involve interactions between cortical and subcortical areas. In this work, our objective was to study whether or not topographic connectivity with conduction delays was sufficient to generate spontaneous waves, as well as to ask whether waves were compatible with asynchronous-irregular dynamics. We, therefore, chose to use the simplest model we could to test what parameters might recapitulate the properties of waves we had observed in the cortex. Undoubtedly, the massive connectivity across cortical areas and subcortical structures impact the features of spontaneous activity in the cortex, and understanding their contribution to the properties of intrinsic traveling waves will be an important avenue of future study.
Traveling waves of neural activity in the awake cortex have been observed under stimulus-evoked 44 , 94 , 95 , behavior-evoked 96 , 97 , 98 , and spontaneous conditions 17 . The fundamental neural circuit mechanism for these waves, however, had remained unclear. Our modeling results suggest that the spontaneous LFP fluctuations we observe traveling as waves in the cortex during active vision result from sparse waves of spiking activity traveling unmyelinated horizontal fibers. The sparse-wave model, which produces activity patterns consistent with the spiking activity observed in vivo, posits that these waves arise from the time delays inherent in communicating spikes across topographic connections within a cortical area. Further, observations from the model suggest that as these sparse waves traverse the massive recurrent connectivity within cortical areas 3 , 36 , 99 , 100 , they produce subthreshold shifts in the local E-I balance that, collectively, modulate cortical excitability. Thus, the model offers an explanation for our empirical finding that perceptual sensitivity varies over space and time depending on the alignment of wave phase 17 . Importantly, these traveling waves need not introduce correlated variability believed to harm perceptual sensitivity; instead, the sparse-wave state weakly modulates the background spiking probability in locally asynchronous-irregular neuron pools. Rather than a source of noise, as would be predicted if waves modulated activity akin to the dense-wave regime, the presence of these sparse waves can boost weak inputs that would otherwise have been imperceptible. These results indicate these traveling waves may be a network mechanism that can improve perceptual processing when aligned to the source of feed-forward signals, without disrupting the computational benefits of the irregular spiking dynamics of individual neurons.
In vivo cortical recordings
The methods for the recordings and behavioral task used in this work was identical to work previously published 17 , which provided the physiology and behavioral data used in this work. Two marmoset monkeys ( C. jacchus ), one male (monkey W) and one female (monkey T), were surgically implanted with a headpost for head stabilization during eye-tracking. The headpost housed an Omnetics connector for a 64-channel multielectrode recording array (Utah array, Blackrock Microsystems), which was implanted in a 7 × 10 mm 2 craniotomy over area MT (stereotaxic coordinates 2 mm anterior, 12 mm dorsal). Monkey W was implanted with an 8 × 8 recording array with channel spacing of 400 µm and monkey T was implanted with a 9 × 9 array with alternating channels removed yielding a channel spacing of 800 µm. Both arrays had a pitch depth of 1.5 mm. The arrays were chronically implanted over area MT using a pneumatic inserter wand. The craniotomy was closed with Duraseal (Integra Life Sciences, monkey W) or Duragen (Integra Life Sciences, monkey T), and covered with a titanium mesh embedded in dental acrylic. All surgical procedures were performed with the monkeys under general anesthesia in an aseptic environment in compliance with NIH guidelines. All experimental methods were approved by the Institutional Animal Care and Use Committee (IACUC) of the Salk Institute for Biological Studies and conformed to NIH guidelines.
Marmosets entered a custom-built marmoset chair that was placed inside a Faraday box with a liquid crystal display (LCD) monitor (ASUS VG248QE) at a distance of 40 cm. The monitor refresh rate was 100 Hz and gamma corrected with a mean gray luminance of 75 cd/m 2 . Electrode voltages were recorded at 30 kHz from the Utah arrays using two Intan RHD2132 amplifiers connected to an Intan RHD2000 USB interface board. The marmosets were headfixed by a headpost for all recordings. Eye position was measured with an IScan CCD infrared camera sampling eye position at 500 Hz. Stimulus presentation and behavioral control were managed through MonkeyLogic (revision date: 4-05-2014, build 1.0.26) in MATLAB (version R2016b). Digital and analog signals were coordinated through National Instrument DAQ cards (NI PCI6621) and BNC breakout boxes (NI BNC2090A). Neural data were broken into two streams for offline processing of spikes (single- and multiunit activity) and LFPs. Spike data were high-pass filtered at 500 Hz and candidate spike waveforms were defined as exceeding 4 SDs of a sliding 1 s window of ongoing voltage fluctuations. Artifacts were rejected if appearing synchronously (within 0.5 ms) on over a quarter of all recorded channels. Segments of data (1.5 ms) around the time of candidate spikes were selected for spike sorting using principal component analysis through the open-source spike sorting software MClust (ver. 4.3.02; A. David Redish, University of Minnesota) in MATLAB. Sorted units were classified as single- or multiunit and single units were validated by the presence of a clear refractory period in the autocorrelogram. LFP data were low-pass filtered at 300 Hz and down-sampled to 1000 Hz for further analysis.
Receptive field mapping
Receptive fields were mapped using a reverse correlation technique. The marmoset was trained to hold fixation on an image (marmoset face, 1 degree of visual angle (DVA) square) presented at the center of the LCD monitor. A drifting Gabor (2° diameter, spatial frequency: 0.5 cycles/degree, temporal frequency 10 cycles/s) appeared at a random position on the monitor between 0° and 18° in azimuth and −15° to 15° in elevation, drifting in one of eight possible directions for 200 ms, after which it disappeared. A new probe then appeared after a random delay drawn from an exponential distribution (mean delay = 40 ms). The sequence repeated until the marmoset broke fixation (defined as an excursion of 1.5° from fixation) or viewed 16 probes. The marmoset was given a juice reward proportional to the number of probes presented. The receptive field for each unit recorded on the array was estimated by calculating the spike-triggered average (STA) stimulus that evoked the maximal response:
The STA is the sum of probe location x i weighted by the spike count y i within the time bin 40–200 ms after probe onset, normalized by the number of all recorded spikes N . We estimated the relative position of each recording array in cortex from the location of estimated receptive fields on each spiking channel, and the known topography of area MT in the marmoset 101 (Fig. 1a ). We excluded from the analysis the upper half of monkey W’s array as the recordings did not appear to be in area MT.
Behavioral task
The marmosets were trained to saccade to a marmoset face to initiate a trial of a visual detection task. Upon their gaze landing on the face, the face turned into a fixation point (0.15 DVA). The marmosets held fixation on the fixation point (1.5° tolerance) for a minimum duration (400 ms monkey W, 300 ms monkey T) awaiting the appearance of a drifting Gabor. The Gabor target was 4 DVA in diameter, which reliably produced evoked responses in the multiunit spiking activity on 1–2 adjacent electrodes. The Gabor had a spatial frequency of 0.5 cycles/degree, a temporal frequency of 10 cycles/s, and could drift in one of up to 8 possible directions. Spontaneous data were analyzed for the period of fixation preceding the appearance of a target and excluded a period of at least 100 ms following the initial saccade to initiate the trial. Early fixation breaks (defined by the excursion of the eye position from the fixation window) were excluded from the analysis. The target only appeared if fixation was held for an additional random duration beyond the minimum duration. The random duration was drawn from an exponential distribution (mean duration = 200 ms) to generate a flat hazard function.
Relative power between spontaneous and evoked LFP
We calculated the relative power between spontaneous and evoked LFP (forward-reverse filtered with a fourth-order Butterworth at 5–50 Hz) by computing the sum-squared LFP magnitude in a window just after stimulus onset (0–200 ms) divided by sum-squared LFP magnitude just before stimulus onset (−200 to 0 ms) on the electrode retinotopically aligned to the stimulus location in cortex. For LFP values λ t at this electrode, where t ∈ {Δ t , 2Δ t , …, n Δ t }, the relative power P is then
where t 1 = −200 ms, t 2 = 0, and t 3 = 200 ms.
Computational simulations
The model consists of N LIF neurons, with N e = 0.8 N excitatory units and N i = 0.2 N inhibitory. The membrane potential V ( i ) of the i th neuron evolves according to the equations
where C m is the membrane capacitance, G L is the leak conductance, E L is the resting membrane potential, τ { e , i } are the excitatory and inhibitory synaptic time constants, g { e , i } (( i )) are the time-dependent synaptic conductances of the i th neuron, and E { e , i } are the reversal potentials for excitatory and inhibitory synaptic transmission, respectively.
When V ( i ) exceeds threshold V T at time t s , the following spike and reset conditions occur:
where V r is the reset potential, t n is the time at which the postsynaptic neuron receives its input following axonal and synaptic delays, G { e , i } are the excitatory and inhibitory synaptic weights, g { e , i } (( j )) are the excitatory and inhibitory conductances of postsynaptic neuron j ≠ i , respectively, and K is the number of postsynaptic targets of neuron i . Immediately after neuron i spikes, it undergoes a refractory period of τ r where the membrane potential is not updated.
Network connectivity and axonal conduction delays
We studied spiking network models with unstructured, random connectivity (random networks, Fig. 2a ) or topographic, locally random connectivity (topographic networks, Fig. 2d ) or a dense version of the topographic network (dense network, Figs. 7 and 8 ). The N e = 0.8 N excitatory neurons, of indices 1, 2, …, N e , where N e is a square number, are arranged uniformly on a 2D grid. Similarly, the N i = 0.2 N inhibitory neurons, of indices N e + 1 , N e + 2 , …, N , where N i is also a square number, are arranged uniformly on a 2D grid. Both grids have side length L and they are concentric, together forming a 2D sheet of the N neurons.
In the random network, connections were randomly and uniformly drawn, and the only delay modeled was that relating to synaptic vesicle release, τ s , which was short and homogeneous across the network. In the topographic and dense networks, connections were randomly drawn from an isotropic 2D Gaussian probability distribution of zero mean and SD σ in either dimension. σ is 400 µm except in Fig. 6a , where the effect of this parameter was studied systematically. In all networks, there were no self- or double-connections. Axonal conduction delays increased linearly with distance between pre- and postsynaptic cells:
where τ ( i , j ) is the delay from neuron i to neuron j , τ s is the same delay representing synaptic vesicle release as in the random network, d ( i , j ) is the Euclidean distance between neurons i and j , and v c (( i , j )) is the axonal conduction speed for the connection from neuron i to neuron j . All distances were calculated taking 2D periodic boundary conditions into account, effectively wrapping the network onto a toroidal topology 58 , 81 . 1D versions of the random and topographic networks were also simulated. The models were the same as in the 2D cases, except the neurons were positioned on a ring of length L with periodic boundary conditions.
Self-sustained activity
Instead of initializing self-sustained activity through a “kick” of external Poisson input spikes 34 , 35 , 38 , which may induce trace activity correlations, we recorded the state variables of a self-sustained network, including membrane potential ( V ( i ) ) and conductance ( g { e , i } ((i)) ), after a long period (10 s) of simulated self-sustained activity. Taking these distributions as a steady state, we then used the Gaussian approximation (mean and variance) to initialize the membrane potentials and conductances with randomly drawn values in the simulations thereafter. After starting the simulation with these initial conditions, networks with approximately balanced excitation and inhibition exhibit self-sustained, irregular spiking activity. Each simulation ran for 1.2 s, from which we eliminated the first 200 ms from our analysis in case of residual initialization artifacts.
Spike train statistics and the asynchronous-irregular regime
To characterize basic spike train statistics, we randomly selected 5000 neurons in the simulation and measured the mean firing rate, CV (defined as the ratio of the standard variation of the interspike interval to the mean for each neuron that has a minimum of three spikes over the simulation window), and the average pairwise correlation (average Pearson’s correlation between spike trains smoothed with a 100 ms window for 1000 randomly selected pairs). To prevent longer simulations with high firing rates during our parallel runs, networks that produced mean firing rates over 25 sp/s had an early exit condition. Simulations were classified as asynchronous irregular if the mean firing rate across all simulated units was >1 and <25 sp/s; the mean CV across all units was >0.7 and <1.4 38 , 102 .
Pairwise spike coherence
Pairwise spike coherence was calculated using multitaper methods 85 . We took the spike trains from the 10 × 10 excitatory neurons comprising the pool for estimating the LFP and an adjacent LFP pool. The 1000 ms of simulation time was broken into 500 ms epochs, stepping 125 ms to cover the full period. The DC component of each unit’s spike train was removed, and tapered with a single Slepian taper, giving an effective smoothing of 2.5 Hz for the 500 ms data windows.
To estimate the coherence between two spike trains x = [ x 1 x 2 … x i … x n ] and y = [ y 1 y 2 … y i … y n ], we first calculated their FFT spectra X = [ X 1 X 2 … X j … X m ] and Y = [ Y 1 Y 2 … Y j … Y m ], respectively, where j denotes the index of spectral frequency. The auto- and cross-spectral densities are calculated as
respectively, where Δ t is the sampling interval, T is the spike train duration, and superscript * denotes complex conjugation. In practice, x and y each represent pools of 100 concurrent spike trains across space. The coherence at a given spectral frequency is calculated as
This coherence calculation is averaged across ten trials to generate an estimate of the average coherence at each frequency as well as an estimate of the variance. For estimating differences in pairwise coherence across networks, we take the frequency with the maximum coherence in the two networks.
NETSIM software
Simulations were generated using a specialized program called NETSIM (v0.1), which is ~1500 lines of C code (available at http://mullerlab.github.io ). Equations in the model were integrated using the forward Euler method with a time step of 0.1 ms. Simulation results were additionally point-checked with shorter timesteps throughout. Random numbers were generated using a C implementation of the ISAAC algorithm 103 (Tom Bartol, personal communication, 2016). To verify the numerical integration in this program, we confirmed the network displayed the correct firing rate for unconnected LIF neurons with varying DC-current injections. We also verified simulations under a simple feed-forward network topology to confirm the accuracy of the simulations. In addition, to ensure reproducibility of our computational simulations 104 , we compared results from NETSIM and Brian2 at specific points in the ( G e , G i ) parameter space for the balanced random network model, verifying that the mean firing rate, CV, and cross-correlation were in agreement between the two simulators.
Network parameter scans
In order to identify the excitatory and inhibitory synaptic conductance weights that produced self-sustained and asynchronous-irregular activity, we simulated networks with 50 values of G e ranging from 0.1 to 5 nS and G i ranging from 1 to 50 nS for a total of 2500 simulations (Table S2 ). In order to determine the effect of network scale on the range of these 2500 simulations that produced self-sustained and asynchronous-irregular activity, we repeated these simulations five times with varying parameters of network size, neuron number, and connections per neuron (Table S2 ). The number of neurons per network size was chosen to maintain a density of 28,125 neurons/mm 2 . The number of connections was chosen to maintain the density of connections within the Gaussian used to assign connections across network sizes. For larger networks (> 2 mm), the connection number did not grow with the size of the network, as 99% of the connections occur within 3 SDs of the Gaussian ( σ = 400 μm). In order to run these simulations across all combinations of network size and conductance parameters, we utilized the Extreme Science Engineering Discovery Environment Comet cluster at the San Diego SuperComputer center at UC San Diego. Data analysis for these simulations was also performed on the Comet cluster running MATLAB. Circular variables were analyzed using the Circular Statistics Toolbox.
Calculation of LFP estimate
We utilized a previously developed proxy for the LFP generated by a network of point LIF neurons, which was systematically developed from a spatially extended model 39 . The LFP estimate λ ( t ) is computed as a weighted sum of the excitatory and inhibitory synaptic currents I e and I i across m excitatory cells in each 10 × 10 neuron pool:
where τ = 6 ms, α = 1.65, and m = 100 excitatory cells. These values of τ and α were found by the authors to have an optimal agreement with the LFP generated from a three-dimensional model of spatially extended multi-compartment model neurons 39 and are the values used here. Here, we computed the LFP using the pooled excitatory and inhibitory synaptic conductances and the driving force between the mean pooled membrane potential and the synaptic reversal potential to calculate the average current in the pool. We verified that this approach was nearly precisely equivalent to the proxy calculated using synaptic inputs to each individual neuron in the pool. This LFP proxy is then computed for each 10 × 10 neuron pool across the 2D network. The LFP proxy was thus independent across each spatial pool, unlike cortical recordings where LFP signals show varying frequency-dependent scales of spatial integration 105 . We note that excluding these effects is a conservative step, as the addition of spatial integration would only increase traveling waves in the LFP. Further, we note that our results do not depend critically on the choice of LFP proxy and our conclusions are unchanged when analyzing the mean membrane potentials or excitatory synaptic conductances.
In order to compare the properties of waves in our model, where LFP signals are independent across space, with waves recorded from the cortex, where electrodes pool signals across a volume ~250 μm in radius 29 , 106 , we convolved the LFP with a 2D Gaussian kernel (with a spatial standard deviation of four LFP bins, corresponding to a radius of 272 μm) before further analysis.
Analysis of spatiotemporal dynamics
To analyze spatiotemporal dynamics in the population activity produced by the spiking network model, we used a technique we recently developed for the wideband analysis of nonstationary data. Briefly, for each real-valued time series λ ( x , y ) ( t ) ∀ x ∈ [1, N c ], y ∈ [1, N r ], where N c and N r are the numbers of columns and rows, respectively, we compute the GP ϕ ( x , y ) of the wideband filtered LFP (fourth-order Butterworth from 5 to 100 Hz) at each point using the corrected analytic signal representation introduced in recent work 17 . We next calculated the gradient of GP \({{{\rm{g}}}}_{(x,y)}(t)\) at each moment in time:
For the spatial gradient, derivatives are taken across the two dimensions of space and are approximated by the appropriate forward and centered finite differences (formulas and code available in the wave MATLAB toolbox: https://github.com/mullerlab/wave-MATLAB/blob/master/analysis/phase_gradient_complex_multiplication.m ). As in previous work, phase differences were implemented as multiplications in the complex plane 44 , 107 ,
so that the unwrapping phase across the two dimensions of the network was not necessary. Here, Λ is the analytic signal representation of λ ( t ). Wavelength is the reciprocal of the phase gradient magnitude at each point in space and time:
As specified in the main text, significance was determined at each point in space and time by comparing observed wavelengths to a spatial shuffle of electrode positions, with the 99th percentile of the shuffle distribution serving as a threshold. The fraction of wave state (Fig. 4a ) is the ratio between points with detected waves over all points α w / α , where α w is the number of points with detected waves and α is the total number of points tested.
Wave speed s ( t ) was computed as the ratio of instantaneous frequency to phase gradient magnitude 96 ,
We further analyzed the spatiotemporal activity patterns using a 2D spectral decomposition in space and time (Figs. 3b and 5b ). To do this, we calculated the 2D FFT of λ ( x , y ) ( t ) for each 1D slice through the network by transforming first in space, and then in time. To account for the spatial and temporal autocorrelation in the data, each slice’s spectrum was normalized by dividing the spectrum produced from a spatial and temporal shuffle respectively. This normalization allows visualization of the spectral line representing traveling waves in the network LFP; it is important to note, however, that the spectral peak representing traveling waves is nevertheless clear in the raw spectrum. The normalized spectrum for each slice through the network was then averaged together.
Calculation of response gain
To quantify the sensitivity of the sparse- and dense-wave network regimes to incoming stimulation, we first identified depolarized and hyperpolarized states from the LFP of a 0.2 × 0.2 mm 2 neuron pool defined by the spike-phase bins that generated the maximum or minimum spiking probability, respectively, for each network regime. We then applied feed-forward stimulation of 20-Hz Poisson spiking inputs to 100 synapses for each neuron within the pool for 10 ms, aligned to the depolarized or hyperpolarized phase in the network. This process was repeated across 40 trials, yielding a distribution of evoked responses. The same random seed was used to construct the networks across each trial, so that the simulations were identical up to the point of stimulation. We calculated the sum of firing rate during stimulus for the evoked response divided by that of the no-stimulus case.
Calculation of the spike-phase index
The degree of spike-phase coupling was measured as the mean resultant vector length for the LFP (filtered with a forward-reverse fourth-order Butterworth filter from 5 to 100 Hz) phase distribution from observed spike times. This measure was calculated using the circ_r function in the Circular Statistics Toolbox for MATLAB (P. Berens, CircStat: A MATLAB Toolbox for Circular Statistics, Journal of Statistical Software, Volume 31, Issue 10, 2009). The mean resultant r of the spike-phase distribution is the normalized sum over complex exponentials of the phase angles ϕ j ,
where M is the number of spikes, the modulus of r (| r | ∈ [0,1]) represents the degree of spike-phase modulation, and i 2 = −1. The closer r is to 0, the more uniform the phase distribution. The closer it is to 1, the more concentrated the phases.
Statistics and reproducibility
Experimental results from in vivo electrophysiology were generated in an initial monkey and replicated in a second monkey with a similar result. All analyses that stemmed from previous experimental work were reproduced from newly written analysis code. Network simulations and subsequent data analysis including statistical tests were initially generated and then repeated on separate machines across different institutes to ensure the reproducibility of the results.
Reporting summary
Further information on research design is available in the Nature Research Reporting Summary linked to this article.
Data availability
Access to the raw simulation data and the processed electrophysiology data used in this study are available at https://github.com/mullerlab/davis2021ncomms . Source data are provided with this paper.
Code availability
An open-source code repository for all analysis methods is available on https://github.com/mullerlab/davis2021ncomms . The open-source code for the custom simulation framework NETSIM is available at https://github.com/mullerlab/NETSIM .
Croner, L. J., Purpura, K. & Kaplan, E. Response variability in retinal ganglion cells of primates. Proc. Natl. Acad. Sci. USA 90 , 8128–8130 (1993).
Article ADS CAS PubMed PubMed Central Google Scholar
Mainen, Z. F. & Sejnowski, T. J. Reliability of spike timing in neocortical neurons. Science 268 , 1503–1506 (1995).
Article ADS CAS PubMed Google Scholar
Destexhe, A., Rudolph, M. & Paré, D. The high-conductance state of neocortical neurons in vivo. Nat. Rev. Neurosci. 4 , 739–751 (2003).
Article CAS PubMed Google Scholar
Destexhe, A. & Rudolph-Lilith, M. Neuronal Noise (Springer US, 2012).
Shadlen, M. N. & Newsome, W. T. Noise, neural codes and cortical organization. Curr. Opin. Neurobiol. 4 , 569–579 (1994).
Arieli, A., Sterkin, A., Grinvald, A. & Aertsen, A. Dynamics of ongoing activity: explanation of the large variability in evoked cortical responses. Science 273 , 1868–1871 (1996).
Tsodyks, M., Kenet, T., Grinvald, A. & Arieli, A. Linking spontaneous activity of single cortical neurons and the underlying functional architecture. Science 286 , 1943–1946 (1999).
Kenet, T., Bibitchkov, D., Tsodyks, M., Grinvald, A. & Arieli, A. Spontaneously emerging cortical representations of visual attributes. Nature 425 , 954–956 (2003).
Han, F., Caporale, N. & Dan, Y. Reverberation of recent visual experience in spontaneous cortical waves. Neuron 60 , 321–327 (2008).
Article CAS PubMed PubMed Central Google Scholar
Ermentrout, G. B. & Kleinfeld, D. Traveling Electrical Waves in Cortex. Neuron 29 , 33–44 (2001).
Santos, E. et al. Radial, spiral and reverberating waves of spreading depolarization occur in the gyrencephalic brain. Neuroimage 99 , 244–255 (2014).
Article PubMed Google Scholar
Benucci, A., Frazor, R. A. & Carandini, M. Standing waves and traveling waves distinguish two circuits in visual cortex. Neuron 55 , 103–117 (2007).
Petersen, C. C. H., Hahn, T. T. G., Mehta, M., Grinvald, A. & Sakmann, B. Interaction of sensory responses with spontaneous depolarization in layer 2/3 barrel cortex. Proc. Natl Acad. Sci. USA 100 , 13638–13643 (2003).
Destexhe, A., Contreras, D. & Steriade, M. Spatiotemporal analysis of local field potentials and unit discharges in cat cerebral cortex during natural wake and sleep states. J. Neurosci. 19 , 4595–4608 (1999).
Churchland, M. M. et al. Stimulus onset quenches neural variability: a widespread cortical phenomenon. Nat. Neurosci. 13 , 369–378 (2010).
Sato, T. K., Nauhaus, I. & Carandini, M. Traveling waves in visual cortex. Neuron 75 , 218–229 (2012).
Davis, Z. W., Muller, L., Martinez-Trujillo, J., Sejnowski, T. & Reynolds, J. H. Spontaneous travelling cortical waves gate perception in behaving primates. Nature https://doi.org/10.1038/s41586-020-2802-y (2020).
Article PubMed PubMed Central Google Scholar
El Boustani, S., Pospischil, M., Rudolph-Lilith, M. & Destexhe, A. Activated cortical states: experiments, analyses and models. J. Physiol. Paris 101 , 99–109 (2007).
Nandy, A., Nassi, J. J., Jadi, M. P. & Reynolds, J. Optogenetically induced low-frequency correlations impair perception. Elife 8 , e35123 (2019).
Destexhe, A. & Contreras, D. Neuronal computations with stochastic network states. Science 314 , 85–90 (2006).
Article ADS MathSciNet CAS PubMed MATH Google Scholar
Renart, A. et al. The asynchronous state in cortical circuits. Science 327 , 587–590 (2010).
Fiser, J., Chiu, C. & Weliky, M. Small modulation of ongoing cortical dynamics by sensory input during natural vision. Nature 431 , 573–578 (2004).
Luczak, A., Bartho, P. & Harris, K. D. Gating of sensory input by spontaneous cortical activity. J. Neurosci. 33 , 1684–1695 (2013).
Gutnisky, D. A., Beaman, C. B., Lew, S. E. & Dragoi, V. Spontaneous Fluctuations in Visual Cortical Responses Influence Population Coding Accuracy. Cereb. Cortex https://doi.org/10.1093/cercor/bhv312 (2016).
Article PubMed Central Google Scholar
Destexhe, A., Rudolph, M., Fellous, J. M. & Sejnowski, T. J. Fluctuating synaptic conductances recreate in vivo-like activity in neocortical neurons. Neuroscience 107 , 13–24 (2001).
Chance, F. S., Abbott, L. F. & Reyes, A. D. Gain modulation from background synaptic input. Neuron 35 , 773–782 (2002).
Tomko, G. J. & Crapper, D. R. Neuronal variability: non-stationary responses to identical visual stimuli. Brain Res. 79 , 405–418 (1974).
Shadlen, M. N. & Newsome, W. T. The variable discharge of cortical neurons: implications for connectivity, computation, and information coding. J. Neurosci. 18 , 3870–3896 (1998).
Katzner, S. et al. Local origin of field potentials in visual cortex. Neuron 61 , 35–41 (2009).
Lindén, H. et al. Modeling the spatial reach of the LFP. Neuron 72 , 859–872 (2011).
Article PubMed CAS Google Scholar
Abbott, L. F. & van Vreeswijk, C. Asynchronous states in networks of pulse-coupled oscillators. Phys. Rev. E Stat. Phys. Plasmas Fluids Relat. Interdiscip. Topics 48 , 1483–1490 (1993).
CAS PubMed Google Scholar
Brunel, N. Dynamics of sparsely connected networks of excitatory and inhibitory spiking neurons. J. Comput. Neurosci. 8 , 183–208 (2000).
Article CAS PubMed MATH Google Scholar
Softky, W. R. & Koch, C. The highly irregular firing of cortical cells is inconsistent with temporal integration of random EPSPs. J. Neurosci. 13 , 334–350 (1993).
Vogels, T. P. & Abbott, L. F. Signal propagation and logic gating in networks of integrate-and-fire neurons. J. Neurosci. 25 , 10786–10795 (2005).
Kumar, A., Schrader, S., Aertsen, A. & Rotter, S. The high-conductance state of cortical networks. Neural Comput. 20 , 1–43 (2008).
Article MathSciNet PubMed MATH Google Scholar
Binzegger, T., Douglas, R. J. & Martin, K. A. C. A quantitative map of the circuit of cat primary visual cortex. J. Neurosci. 24 , 8441–8453 (2004).
Atapour, N. et al. Neuronal Distribution Across the Cerebral Cortex of the Marmoset Monkey (Callithrix jacchus). Cereb. Cortex 29 , 3836–3863 (2019).
Destexhe, A. Self-sustained asynchronous irregular states and Up-Down states in thalamic, cortical and thalamocortical networks of nonlinear integrate-and-fire neurons. J. Comput. Neurosci. 27 , 493–506 (2009).
Article MathSciNet PubMed Google Scholar
Mazzoni, A. et al. Computing the Local Field Potential (LFP) from Integrate-and-Fire Network Models. PLoS Comput. Biol. 11 , e1004584 (2015).
Article PubMed PubMed Central CAS Google Scholar
Hellwig, B. A quantitative analysis of the local connectivity between pyramidal neurons in layers 2/3 of the rat visual cortex. Biol. Cybern. 82 , 111–121 (2000).
Levy, R. B. & Reyes, A. D. Spatial profile of excitatory and inhibitory synaptic connectivity in mouse primary auditory cortex. J. Neurosci. 32 , 5609–5619 (2012).
Girard, P., Hupé, J. M. & Bullier, J. Feedforward and feedback connections between areas V1 and V2 of the monkey have similar rapid conduction velocities. J. Neurophysiol. 85 , 1328–1331 (2001).
Cagigal, M. P., Vega, L. & Prieto, P. Movement characterization with the spatiotemporal Fourier transform of low-light-level images. Appl. Opt. 34 , 1769–1774 (1995).
Muller, L., Reynaud, A., Chavane, F. & Destexhe, A. The stimulus-evoked population response in visual cortex of awake monkey is a propagating wave. Nat. Commun. 5 , 3675 (2014).
Article ADS PubMed Google Scholar
Muller, L. et al. Rotating waves during human sleep spindles organize global patterns of activity that repeat precisely through the night. Elife 5 , e17267 (2016).
Stettler, D. D., Das, A., Bennett, J. & Gilbert, C. D. Lateral connectivity and contextual interactions in macaque primary visual cortex. Neuron 36 , 739–750 (2002).
Angelucci, A. et al. Circuits for local and global signal integration in primary visual cortex. J. Neurosci. 22 , 8633–8646 (2002).
Bringuier, V., Chavane, F., Glaeser, L. & Frégnac, Y. Horizontal propagation of visual activity in the synaptic integration field of area 17 neurons. Science 283 , 695–699 (1999).
Gonzalez-Burgos, G., Barrionuevo, G. & Lewis, D. A. Horizontal Synaptic Connections in Monkey Prefrontal Cortex. Cerebral Cortex 10 , 82–92 (2000).
Kuhn, A., Aertsen, A. & Rotter, S. Neuronal integration of synaptic input in the fluctuation-driven regime. J. Neurosci. 24 , 2345–2356 (2004).
DeWeese, M. R. & Zador, A. M. Non-Gaussian membrane potential dynamics imply sparse, synchronous activity in auditory cortex. J. Neurosci. 26 , 12206–12218 (2006).
Hirsch, J. A. & Gilbert, C. D. Synaptic physiology of horizontal connections in the cat’s visual cortex. J. Neurosci. 11 , 1800–1809 (1991).
Frégnac, Y., Bringuier, V. & Chavane, F. Synaptic integration fields and associative plasticity of visual cortical cells in vivo. J. Physiol. Paris 90 , 367–372 (1996).
Frégnac, Y. Reading Out the Synaptic Echoes of Low-Level Perception inV1. in Computer Vision – ECCV2012 . Workshops and Demonstrations 486–495 (Springer Berlin Heidelberg, 2012).
Gerard-Mercier, F., Carelli, P. V., Pananceau, M., Troncoso, X. G. & Frégnac, Y. Synaptic Correlates of Low-Level Perception in V1. J. Neurosci. 36 , 3925–3942 (2016).
Destexhe, A. Oscillations, complex spatiotemporal behavior, and information transport in networks of excitatory and inhibitory neurons. Phys. Rev. E Stat. Phys. Plasmas Fluids Relat. Interdiscip. Topics 50 , 1594–1606 (1994).
Zerlaut, Y. & Destexhe, A. Enhanced Responsiveness and Low-Level Awareness in Stochastic Network States. Neuron 94 , 1002–1009 (2017).
Mehring, C., Hehl, U., Kubo, M., Diesmann, M. & Aertsen, A. Activity dynamics and propagation of synchronous spiking in locally connected random networks. Biol. Cybern. 88 , 395–408 (2003).
Article PubMed MATH Google Scholar
Crook, S. M., Ermentrout, G. B., Vanier, M. C. & Bower, J. M. The role of axonal delay in the synchronization of networks of coupled cortical oscillators. J. Comput. Neurosci. 4 , 161–172 (1997).
Golomb, D. & Ermentrout, G. B. Continuous and lurching traveling pulses in neuronal networks with delay and spatially decaying connectivity. Proc. Natl Acad. Sci. USA 96 , 13480–13485 (1999).
Golomb, D. & Ermentrout, G. B. Effects of delay on the type and velocity of travelling pulses in neuronal networks with spatially decaying connectivity. Network 11 , 221–246 (2000).
Osan, R. & Ermentrout, B. Two dimensional synaptically generated traveling waves in a theta-neuron neural network. Neurocomputing 38 , 789–795 (2001).
Article Google Scholar
Roxin, A., Brunel, N. & Hansel, D. Role of delays in shaping spatiotemporal dynamics of neuronal activity in large networks. Phys. Rev. Lett. 94 , 238103 (2005).
Article ADS PubMed CAS Google Scholar
Roxin, A., Brunel, N. & Hansel, D. Rate Models with Delays and the Dynamics of Large Networks of Spiking Neurons. Progr. Theoret. Phys. Suppl. 161 , 68–85 (2006).
Article ADS Google Scholar
Kriener, B., Helias, M., Aertsen, A. & Rotter, S. Correlations in spiking neuronal networks with distance dependent connections. J. Comput. Neurosci. 27 , 177–200 (2009).
Article MathSciNet PubMed PubMed Central Google Scholar
Kriener, B., Helias, M., Rotter, S., Diesmann, M. & Einevoll, G. T. How pattern formation in ring networks of excitatory and inhibitory spiking neurons depends on the input current regime. Front. Comput. Neurosci. 7 , 187 (2013).
PubMed Google Scholar
Senk, J. et al. Conditions for wave trains in spiking neural networks. Phys. Rev. Research 2 , 023174 (2020).
Article ADS CAS Google Scholar
Ermentrout, B. Neural networks as spatio-temporal pattern-forming systems. Rep. Prog. Phys. 61 , 353–430 (1998).
Bressloff, P. C. Traveling waves and pulses in a one-dimensional network of excitable integrate-and-fire neurons. J. Math. Biol. 40 , 169–198 (2000).
Article MathSciNet CAS PubMed MATH Google Scholar
Pinto, D. & Ermentrout, G. Spatially Structured Activity in Synaptically Coupled Neuronal Networks: I. Traveling Fronts and Pulses. SIAM J. Appl. Math. 62 , 206–225 (2001).
Article MathSciNet MATH Google Scholar
Liley, D. T. J., Cadusch, P. J. & Dafilis, M. P. A spatially continuous mean field theory of electrocortical activity. Network 13 , 67–113 (2002).
Coombes, S., Lord, G. J. & Owen, M. R. Waves and bumps in neuronal networks with axo-dendritic synaptic interactions. Physica D 178 , 219–241 (2003).
Article ADS MathSciNet MATH Google Scholar
Atay, F. M. & Hutt, A. Stability and Bifurcations in Neural Fields with Finite Propagation Speed and General Connectivity. SIAM J. Appl. Math. 65 , 644–666 (2004).
Venkov, N. A., Coombes, S. & Matthews, P. C. Dynamic instabilities in scalar neural field equations with space-dependent delays. Physica D 232 , 1–15 (2007).
Veltz, R. Interplay Between Synaptic Delays and Propagation Delays in Neural Field Equations. SIAM J. Appl. Dyn. Syst. 12 , 1566–1612 (2013).
Meijer, H. G. E. & Coombes, S. Travelling waves in models of neural tissue: from localised structures to periodic waves. EPJ Nonlinear Biomedical Physics 2 , 3 (2014).
Visser, S., Nicks, R., Faugeras, O. & Coombes, S. Standing and travelling waves in a spherical brain model: The Nunez model revisited. Physica D 349 , 27–45 (2017).
Article ADS MathSciNet CAS PubMed PubMed Central MATH Google Scholar
Gong, P. & van Leeuwen, C. Distributed dynamical computation in neural circuits with propagating coherent activity patterns. PLoS Comput. Biol. 5 , e1000611 (2009).
Article MathSciNet PubMed PubMed Central CAS Google Scholar
Keane, A. & Gong, P. Propagating waves can explain irregular neural dynamics. J. Neurosci. 35 , 1591–1605 (2015).
Huang, C. et al. Circuit Models of Low-Dimensional Shared Variability in Cortical Networks. Neuron 101 , 337–348.e4 (2019).
Kumar, A., Rotter, S. & Aertsen, A. Conditions for propagating synchronous spiking and asynchronous firing rates in a cortical network model. J. Neurosci. 28 , 5268–5280 (2008).
Brunel, N. & Hakim, V. Fast global oscillations in networks of integrate-and-fire neurons with low firing rates. Neural Comput. 11 , 1621–1671 (1999).
Brunel, N. & Wang, X.-J. What Determines the Frequency of Fast Network Oscillations With Irregular Neural Discharges? I. Synaptic Dynamics and Excitation-Inhibition Balance. Journal of Neurophysiology 90 , 415–430 (2003).
Palmigiano, A., Geisel, T., Wolf, F. & Battaglia, D. Flexible information routing by transient synchrony. Nat. Neurosci . https://doi.org/10.1038/nn.4569 (2017).
Mitchell, J. F., Sundberg, K. A. & Reynolds, J. H. Spatial attention decorrelates intrinsic activity fluctuations in macaque area V4. Neuron 63 , 879–888 (2009).
Zohary, E., Shadlen, M. N. & Newsome, W. T. Correlated neuronal discharge rate and its implications for psychophysical performance. Nature 370 , 140–143 (1994).
Abbott, L. F. & Dayan, P. The Effect of Correlated Variability on the Accuracy of a Population Code. Neural Computation 11 , 91–101 (1999).
Averbeck, B. B., Latham, P. E. & Pouget, A. Neural correlations, population coding and computation. Nat. Rev. Neurosci. 7 , 358–366 (2006).
Cohen, M. R. & Maunsell, J. H. R. Attention improves performance primarily by reducing interneuronal correlations. Nat. Neurosci. 12 , 1594–1600 (2009).
Rudolph, M., Pospischil, M., Timofeev, I. & Destexhe, A. Inhibition determines membrane potential dynamics and controls action potential generation in awake and sleeping cat cortex. J. Neurosci. 27 , 5280–5290 (2007).
Morrison, A., Aertsen, A. & Diesmann, M. Spike-Timing-Dependent Plasticity in Balanced Random Networks. Neural Computation 19 , 1437–1467 (2007).
Reinhold, K., Lien, A. D. & Scanziani, M. Distinct recurrent versus afferent dynamics in cortical visual processing. Nat. Neurosci. 18 , 1789–1797 (2015).
Guo, Z. V. et al. Maintenance of persistent activity in a frontal thalamocortical loop. Nature 545 , 181–186 (2017).
Yang, Z., Heeger, D. J., Blake, R. & Seidemann, E. Long-range traveling waves of activity triggered by local dichoptic stimulation in V1 of behaving monkeys. J. Neurophysiol. 113 , 277–294 (2015).
Chemla, S. et al. Suppressive Traveling Waves Shape Representations of Illusory Motion in Primary Visual Cortex of Awake Primate. J. Neurosci. 39 , 4282–4298 (2019).
Rubino, D., Robbins, K. A. & Hatsopoulos, N. G. Propagating waves mediate information transfer in the motor cortex. Nat. Neurosci. 9 , 1549–1557 (2006).
Zanos, T. P., Mineault, P. J., Nasiotis, K. T., Guitton, D. & Pack, C. C. A sensorimotor role for traveling waves in primate visual cortex. Neuron 85 , 615–627 (2015).
Balasubramanian, K. et al. Propagating Motor Cortical Dynamics Facilitate Movement Initiation. Neuron 106 , 526–536.e4 (2020).
Braitenberg, V. & Schüz, A. Cortex: Statistics and Geometry of Neuronal Connectivity . (Springer, 1998).
Markov, N. T. et al. Weight consistency specifies regularities of macaque cortical networks. Cereb. Cortex 21 , 1254–1272 (2011).
Rosa, M. G. P. & Elston, G. N. Visuotopic organisation and neuronal response selectivity for direction of motion in visual areas of the caudal temporal lobe of the marmoset monkey (Callithrix jacchus): middle temporal area, middle temporal crescent, and surrounding cortex. J. Comp. Neurol. 393 , 505–527 (1998).
Voges, N. & Perrinet, L. Complex dynamics in recurrent cortical networks based on spatially realistic connectivities. Front. Comput. Neurosci. 6 , 41 (2012).
Jenkins, R. J. ISAAC. in Fast Software Encryption 41–49 (Springer Berlin Heidelberg, 1996).
Davison, A. P. et al. PyNN: A Common Interface for Neuronal Network Simulators. Front. Neuroinform. 2 , 11 (2008).
Łęski, S., Lindén, H., Tetzlaff, T., Pettersen, K. H. & Einevoll, G. T. Frequency dependence of signal power and spatial reach of the local field potential. PLoS Comput. Biol. 9 , e1003137 (2013).
Buzsáki, G., Anastassiou, C. A. & Koch, C. The origin of extracellular fields and currents—EEG, ECoG, LFP and spikes. Nat. Rev. Neurosci. 13 , 407–420 (2012).
Feldman, M. Hilbert transform in vibration analysis. Mech. Syst. Signal Process. 25 , 735–802 (2011/4).
Download references
Acknowledgements
We thank Katie Williams, Sean Adams, and Mat LeBlanc for their contributions to this project, in addition to the San Diego Supercomputer Center for their support for this project. We would also like to thank Tom Bartol for the discussions on the software implementation. This work was funded by Gatsby Charitable Foundation, the Fiona and Sanjay Jha Chair in Neuroscience, the Canadian Institute for Health Research and NSF (NeuroNex Grant No. 2015276), the Swartz Foundation, NIH Grants R01-EY028723, T32 EY020503-06, T32 MH020002-16A, P30 EY019005, and BrainsCAN at Western University through the Canada First Research Excellence Fund (CFREF).
Author information
These authors contributed equally: Zachary W. Davis, Gabriel B. Benigno, Charlee Fletterman.
These authors jointly supervised this work: Terrence J. Sejnowski, John H. Reynolds, Lyle Muller.
Authors and Affiliations
The Salk Institute for Biological Studies, La Jolla, CA, USA
Zachary W. Davis, Charlee Fletterman, Theo Desbordes, Terrence J. Sejnowski & John H. Reynolds
Department of Applied Mathematics, Western University, London, ON, Canada
Gabriel B. Benigno & Lyle Muller
Brain and Mind Institute, Western University, London, ON, Canada
Gabriel B. Benigno, Christopher Steward & Lyle Muller
You can also search for this author in PubMed Google Scholar
Contributions
Conceptualization: Z.W.D., G.B.B., C.F., T.D., T.J.S., J.H.R., L.M.; data curation: Z.W.D., G.B.B. C.F., T.D., C.S., L.M.; formal analysis: Z.W.D., G.B.B., C.F., T.D., L.M.; funding acquisition: Z.W.D., T.J.S., J.H.R., L.M.; investigation: Z.W.D., G.B.B., L.M.; methodology: Z.W.D., G.B.B., C.F., T.S., C.S., J.H.R., L.M.; supervision: T.J.S., J.H.R., L.M.; visualization: Z.W.D., G.B.B., L.M.; writing—original draft, Z.W.D., G.B.B., C.F., T.D., J.H.R., L.M.; writing—review and editing, Z.W.D., G.B.B., T.J.S., J.H.R., L.M.
Corresponding authors
Correspondence to Zachary W. Davis , John H. Reynolds or Lyle Muller .
Ethics declarations
Competing interests.
The authors declare no competing interests.
Additional information
Peer review information Nature Communications thanks Bastian Pietras and the other, anonymous, reviewer(s) for their contribution to the peer review of this work. Peer reviewer reports are available.
Publisher’s note Springer Nature remains neutral with regard to jurisdictional claims in published maps and institutional affiliations.
Supplementary information
Supplementary information, peer review file, description of additional supplementary files, supplementary movie 1, supplementary movie 2, supplementary movie 3, supplementary movie 4, supplementary movie 5, reporting summary, source data, source data, rights and permissions.
Open Access This article is licensed under a Creative Commons Attribution 4.0 International License, which permits use, sharing, adaptation, distribution and reproduction in any medium or format, as long as you give appropriate credit to the original author(s) and the source, provide a link to the Creative Commons license, and indicate if changes were made. The images or other third party material in this article are included in the article’s Creative Commons license, unless indicated otherwise in a credit line to the material. If material is not included in the article’s Creative Commons license and your intended use is not permitted by statutory regulation or exceeds the permitted use, you will need to obtain permission directly from the copyright holder. To view a copy of this license, visit http://creativecommons.org/licenses/by/4.0/ .
Reprints and permissions
About this article
Cite this article.
Davis, Z.W., Benigno, G.B., Fletterman, C. et al. Spontaneous traveling waves naturally emerge from horizontal fiber time delays and travel through locally asynchronous-irregular states. Nat Commun 12 , 6057 (2021). https://doi.org/10.1038/s41467-021-26175-1
Download citation
Received : 22 November 2020
Accepted : 17 September 2021
Published : 18 October 2021
DOI : https://doi.org/10.1038/s41467-021-26175-1
Share this article
Anyone you share the following link with will be able to read this content:
Sorry, a shareable link is not currently available for this article.
Provided by the Springer Nature SharedIt content-sharing initiative
This article is cited by
Waves traveling over a map of visual space can ignite short-term predictions of sensory input.
- Gabriel B. Benigno
- Roberto C. Budzinski
- Lyle Muller
Nature Communications (2023)
Learning heterogeneous delays in a layer of spiking neurons for fast motion detection
- Antoine Grimaldi
- Laurent U. Perrinet
Biological Cybernetics (2023)
By submitting a comment you agree to abide by our Terms and Community Guidelines . If you find something abusive or that does not comply with our terms or guidelines please flag it as inappropriate.
Quick links
- Explore articles by subject
- Guide to authors
- Editorial policies
Sign up for the Nature Briefing newsletter — what matters in science, free to your inbox daily.

UAW vote at Tennessee plant will test resurgent labor movement
4,000 workers at the Volkswagen facility are eligible to vote.
Carmakers and labor leaders are closely watching for results to be released on Friday in a seismic union election that could supercharge organizing in the nation’s auto sector and demonstrate the strength of a resurgent labor movement through a victory in the South, a region typically resistant to unions.
As many as 4,300 employees at a Volkswagen plant in Chattanooga, Tennessee, are voting on whether to join the United Auto Workers .
Workers began casting ballots this week in the most significant test for the UAW since a strike last fall against the Big 3 U.S. carmakers that delivered substantial gains and a burst of interest from employees at non-union firms.
"Workers in America are fed up with being left behind and unions are the path to dignity on and off the job," UAW president Shawn Fain said in February in a post on X , after having met with workers at the Volkswagen facility.
MORE: Why the UAW vote at Volkswagen is significant for workers across US
The labor agreements at the Big 3 last year prompted some non-union competitors to offer pay increases and other benefits, putting their employees in closer alignment with UAW members. Honda, Nissan and Tesla are among the companies that raised wages for U.S. employees after the UAW deal.
The breakthrough also triggered a wave of UAW organizing, the union says. Over 10,000 non-union auto workers have signed cards in support of the UAW in recent months and organizing campaigns have broken out at more than two dozen facilities, the union said in a statement last month.
The election at the Volkswagen plant in Chattanooga offers a difficult test, even as the union appears poised for growth. The UAW suffered narrow defeats in two elections at the facility over the past decade.
Governors of six southern states, including Tennessee Gov. Bill Lee, issued a joint statement this week criticizing the UAW and voicing general opposition toward unions.
"As Governors, we have a responsibility to our constituents to speak up when we see special interests looking to come into our state and threaten our jobs and the values we live by," they wrote .
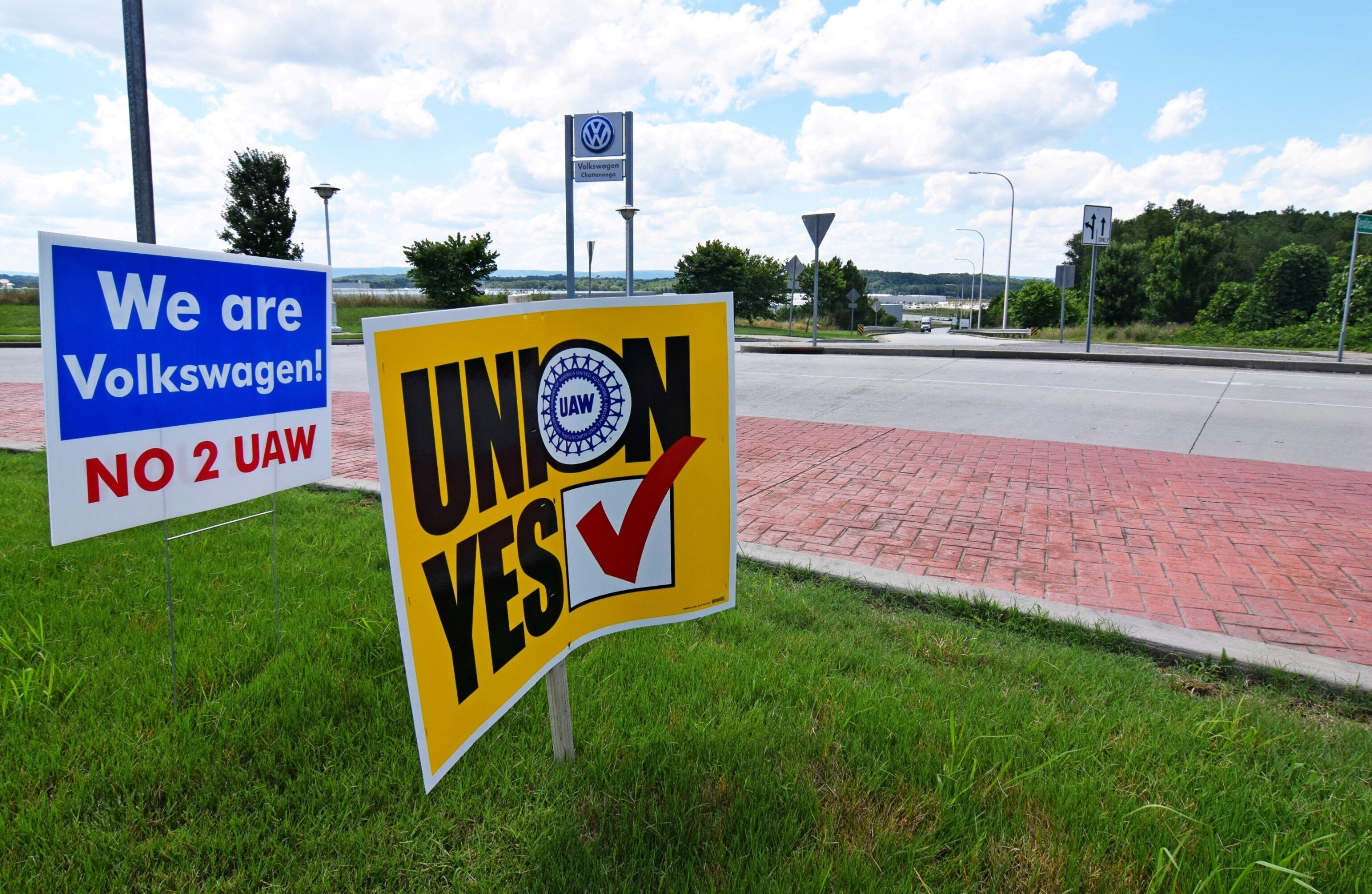
For its part, Volkswagen has remained publicly neutral toward the union drive. In a statement, the company told ABC News that it supports the union election process.
"We respect our workers' right to a democratic process and to determine who should represent their interests," Volkswagen said.
Related Stories
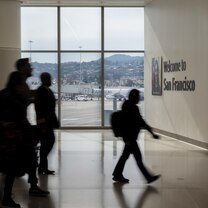
San Francisco sues Oakland over airport name
- Apr 18, 4:19 PM
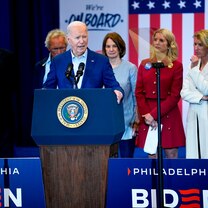
Biden gets Kennedy family endrosement.
- Apr 18, 11:22 AM

More human remains of missing woman found on beach
- Apr 18, 5:57 PM
"We fully support an NLRB vote so every team member has a chance to a secret ballot vote on this important decision. Volkswagen is proud of our working environment in Chattanooga that provides some of the best paying jobs in the area," the company added
A union victory would help bolster the UAW’s membership, which has dropped steadily from a peak of 1.5 million workers in 1970 to 370,000 last year.
MORE: Why is Trump's Truth Social stock plummeting?
The opportunity for membership expansion at the UAW, just months after its high-profile strike against the Big 3, exemplifies a wider trend in the U.S. labor movement.
More than 500,000 workers went out on strike nationwide in 2023, more than doubling the figure recorded a year earlier, according to Cornell University's School of Industrial and Labor Relations .
The uptick in activity, however, failed to translate into union membership gains. Only 10% of U.S. workers belonged to unions last year, little changed from the year prior, U.S. Bureau of Labor Statistics data showed.
The election in Chattanooga will test whether the headline-grabbing labor strikes can translate into a resurgence of union growth, said Stephen Silvia, a professor at American University and the author of "The UAW's Southern Gamble."
"Historically, unionization has proceeded in waves," Silvia said. "Is this a wave? That’s what we’ll see."
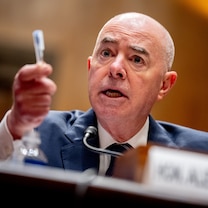
Mayorkas faces GOP after impeachment dismissed
- Apr 18, 2:43 PM
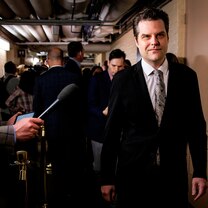
Gaetz attended 2017 party, woman's statement says
- Apr 19, 5:04 AM
ABC News Live
24/7 coverage of breaking news and live events
Iran launches drone attack at Israel, expected to unfold 'over hours'
- Medium Text
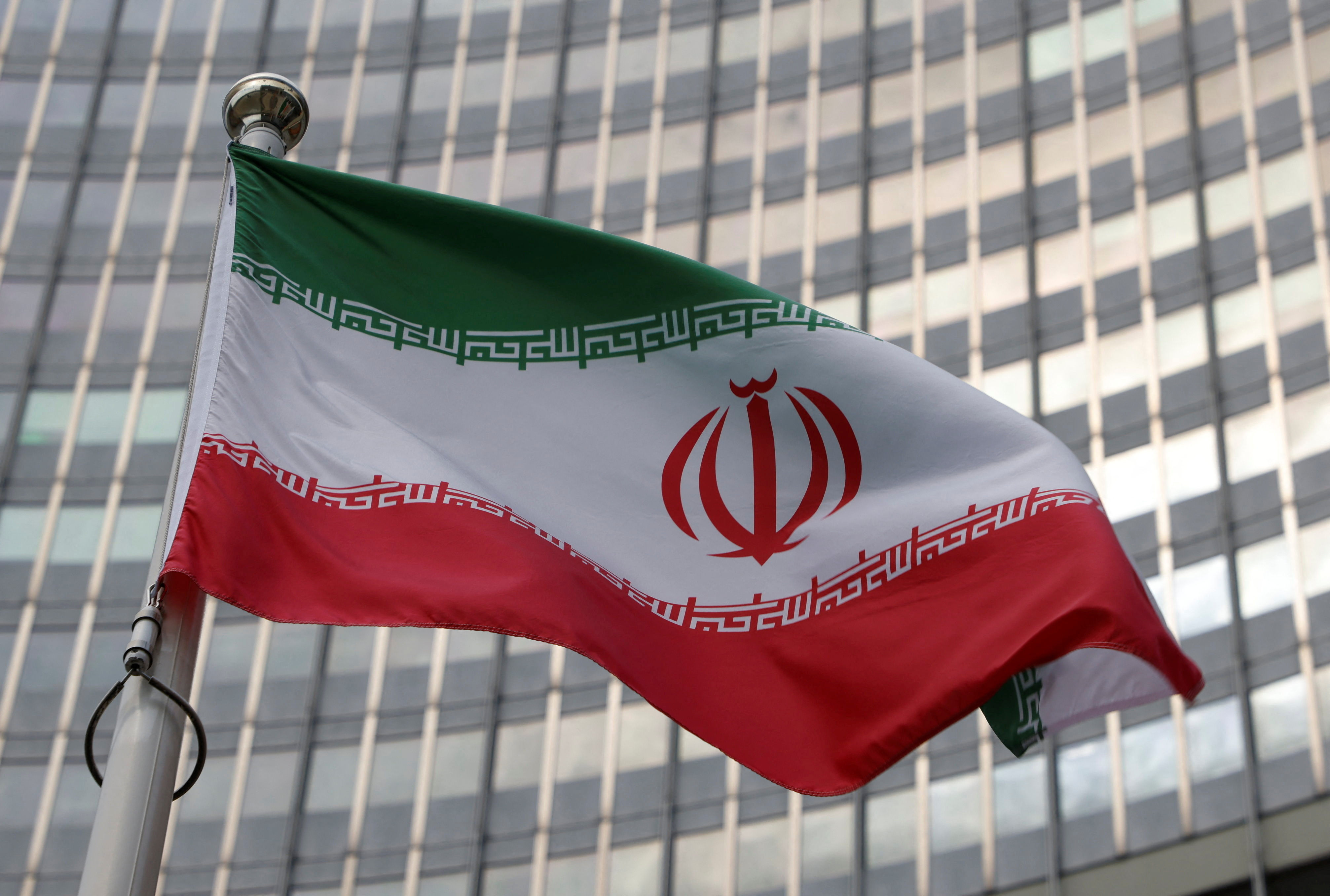
Coming soon: Get the latest news and expert analysis about the state of the global economy with Reuters Econ World. Sign up here.
Reporting by Dan Williams in Jerusalem, Parisa Hafezi in Dubai, Timur Azhari in Baghdad, Eric Beech and Doina Chiacu in Washington; Writing by Angus McDowall; Editing by Jonathan Oatis
Our Standards: The Thomson Reuters Trust Principles. New Tab , opens new tab
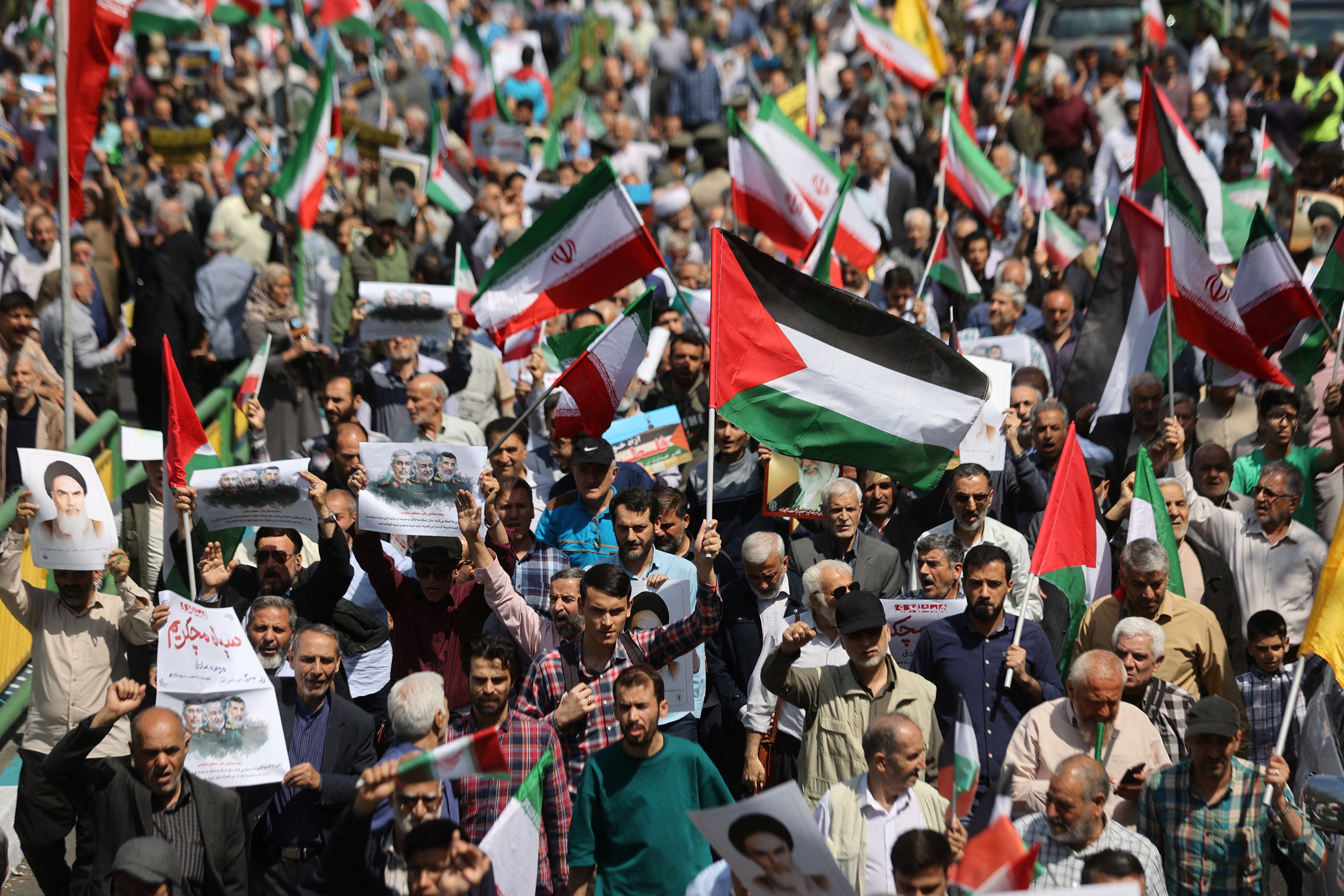
World Chevron
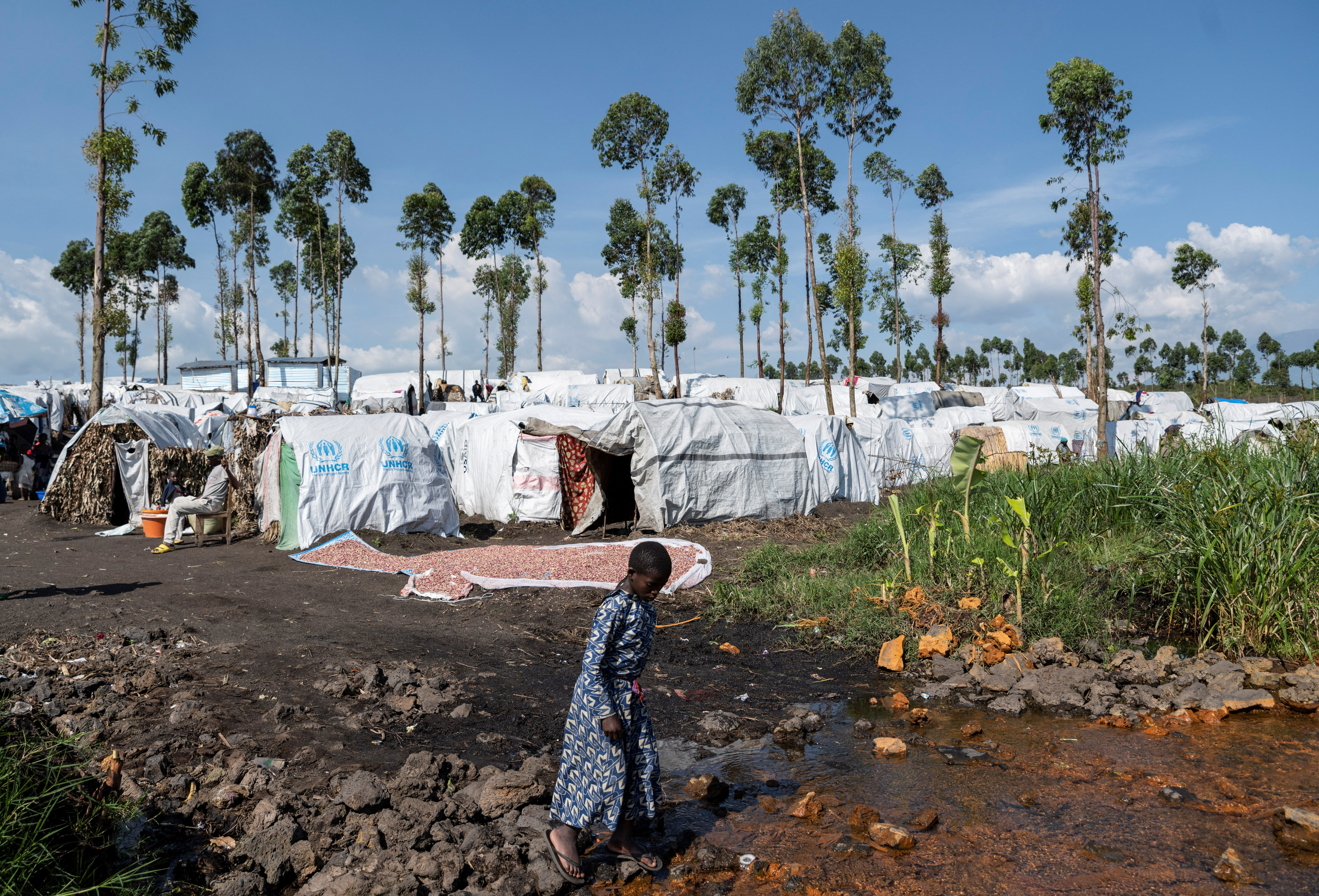
DR Congo should file complaint against Rwanda with ICJ, says Belgian envoy
Democratic Republic of Congo should file a complaint with the International Court of Justice over Rwanda's failure to respect its border, Belgium's ambassador to Congo said on Friday at a meeting to assess the deepening crisis in eastern Congo.
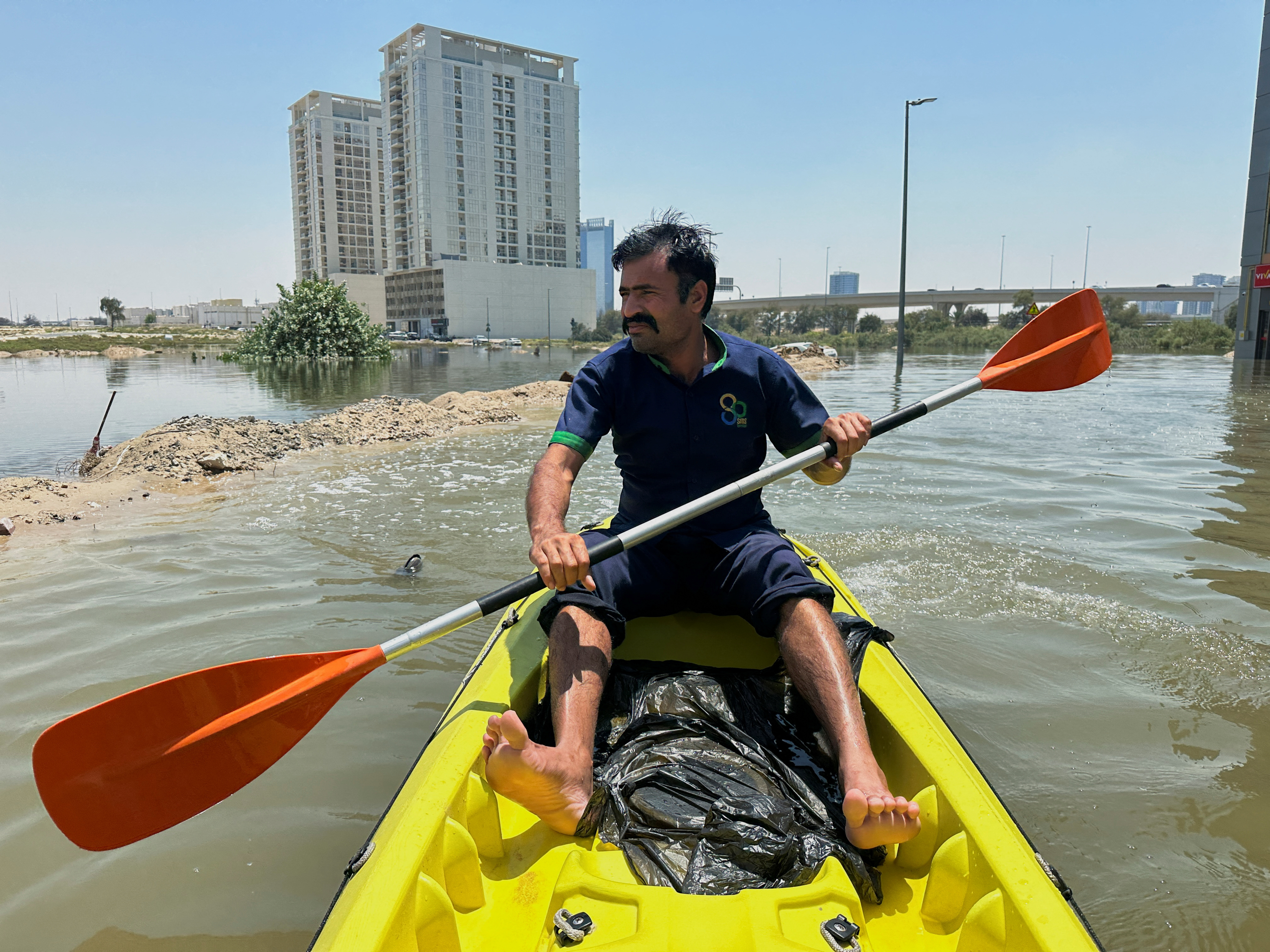
EU proposing post-Brexit joint youth work and study scheme with UK
According to the Commission, they do not foresee the scheme allowing UK citizens to travel around the Schengen area unimpeded, but rather the visa would only be valid for a single state.
By Adam Parsons, Europe correspondent, and Tim Baker, political reporter
Friday 19 April 2024 00:21, UK
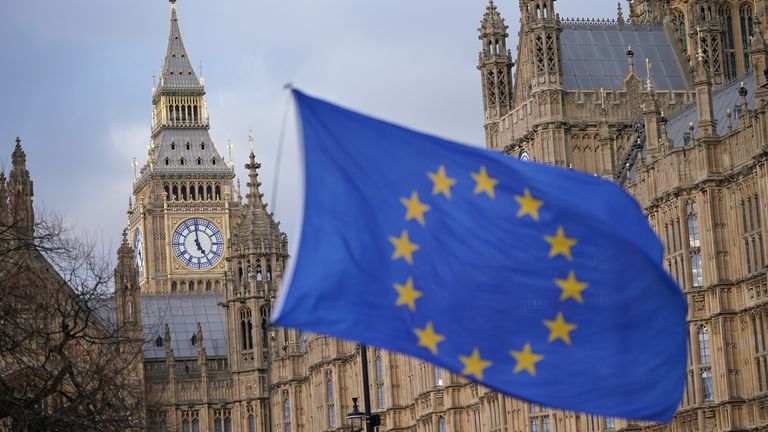
Young people could be able to move more easily between the UK and Europe and stay longer to work, study and train under proposed plans by the European Union
Under the proposal, which has been put forward by the European Commission, new rules would be drawn up to allow for greater movement between the UK and EU countries for people aged between 18 and 30.
Formal negotiations have not yet begun, and a UK source told Sky News no formal proposal had been put forward by Brussels to begin negotiating on.
The proposal will be further discussed by the European Council, which represents all the nations, before negotiations start in earnest.
Politics latest: Police issues Mark Menzies update
The plans proposed by the EU would allow young people to stay in Europe for periods of four years, with the same rules extended to EU citizens coming to Britain.
It would also mean EU students paying the same fees as British ones. Since Brexit , UK universities have charged much higher fees to European students.
More on Brexit
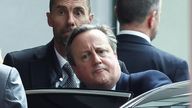
UK, EU and Spain hail 'significant progress' in Gibraltar talks
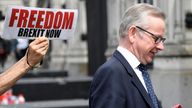
Michael Gove admits to 'moral cowardice' during Brexit campaign
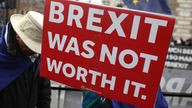
Warnings of higher food prices and empty shelves due to new post-Brexit border fees
Related Topics:
- European Union
Announcing the move, the European Commission said it wanted to take an "innovative" approach to tackling the barriers experienced by young people looking to travel from the EU to the UK and vice versa for longer periods.
"The objective would be to facilitate youth exchanges, making it easier for young citizens to travel, work and live in the UK, with reciprocity for young UK nationals in a member state," said the Commission, in a statement.
"The United Kingdom's withdrawal from the European Union has hit young people in the EU and UK who would like to study, work and live abroad particularly hard," claimed Maros Sefcovic, the Commission's vice-president who oversees relations with the UK.
"Our aim is to rebuild human bridges between young Europeans on both sides of the Channel."
This scheme is separate to the 90-day visa-less travel that UK citizens can take advantage of on the continent.
It added: "It is not about conferring to young UK nationals the benefits of the fundamental freedom of movement enjoyed by EU citizens."
However, visas would not be "purpose-bound" and would allow people to work, travel or study.
Read more: UK to rejoin EU Horizon programme through new agreement New Brexit border post could be demolished
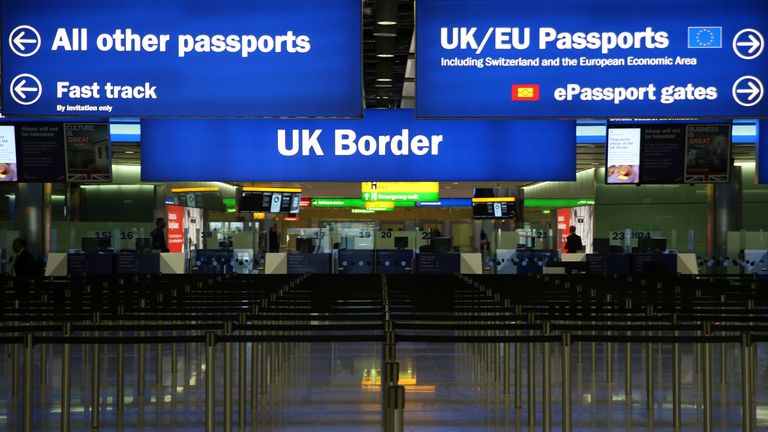
Rather than just being a free exchange, the EU also says it wants people taking part in the scheme to be subject to checks with the bloc or the UK able to reject applications - for example if someone was thought to be a threat to public policy, security or health.
The UK government currently has a series of agreements with 13 individual countries - including New Zealand, South Korea, Andorra, Iceland and India, which provide a similar pathway to what the EU is proposing.
The government has said it is open to the idea of extending those agreements with European countries, but has shied away from doing a pan-EU deal and there will be those who fear that an agreement such as this would be the first step towards the UK being drawn into a "freedom of movement" deal.
The EU said it wants a group deal to "ensure that all member states are treated equally in respect of mobility of young people to the UK" - but the current government seems to prefer its current set of agreements.
The Commission has rejected these fears, with sources insisting there is no intention of either pulling the UK into such a deal, or even offering it.
A Number 10 spokesperson said: "We have spoken about wanting to reduce legal migration and also about wanting to support UK talent and skills and that's why we have a system in place whereby we have a number of agreements with individual EU member states where that works in our interests and we have that rather than a Commission-wide agreement."
Labour denied it had plans for a youth mobility scheme.
Be the first to get Breaking News
Install the Sky News app for free

👉 Tap here to get Electoral Dysfunction on Apple, Spotify or wherever you get your podcasts 👈
Kate Nicholls, chief executive of UKHospitality, a trade body representing the hospitality sector in the UK, welcomed the move.
She said: "Such an agreement would be a huge success for hospitality and tourism and, practically, it would solve challenges for the live music and events sectors."
Related Topics
EU woos Labour with freedom of movement deal
Europeans aged 18 to 30 would be able to work, travel and study in UK and vice versa
The European Union has proposed a freedom of movement-style deal for young Europeans to appeal to a future Labour government .
The youth mobility scheme would allow Europeans aged 18 to 30 to come to Britain for up to four years to work, travel and study. It could pave the way for thousands of European waiters, baristas and au pairs to plug gaps in the British workforce .
Britons would also be able to take part in the reciprocal scheme by moving to the continent under the deal, proposed by the European Commission on Thursday.
Maros Sefcovic, the EU’s Brexit negotiator , said: “The United Kingdom’s withdrawal from the European Union has hit young people in the EU and the UK who would like to study, work and live abroad particularly hard.
“Today, we take the first step towards an ambitious but realistic agreement between the EU and the UK that would fix this issue. Our aim is to rebuild human bridges between young Europeans on both sides of the Channel.”
The offer would probably appeal to Sir Keir Starmer, the Labour leader, who has signalled that he would seek closer ties with the EU if his party wins the election.
Youth mobility schemes
The Home Office has also engaged in discussions with some EU countries after being asked by Rishi Sunak’s Downing Street to agree more youth mobility schemes.
The UK already has reciprocal schemes with 10 non-EU countries including Australia, New Zealand, Canada, Japan and Iceland.
They allow those eligible to travel and work in Britain without needing a sponsor or meeting other requirements, such as salary thresholds, without significantly contributing to net migration.
The European Commission said it was making the offer of an EU-wide deal because the UK had already spoken with a number of member states to discuss youth mobility. If a deal is reached, it would be Brussels’ first youth mobility treaty with a third country.
Under the bloc’s offer, Britain would have to agree to equal treatment on tuition and visa fees, and healthcare surcharges.
In an online Q&A of its proposals, the EU makes it clear that the scheme would not replace existing paths to obtaining visas and that either side would have the ability to reject applications because of threats to public security and health.
It is unlikely that negotiations will get under way immediately, with the EU’s 27 member states still required to agree on the Commission’s proposal.
The forthcoming European Parliament elections and general election are also likely to act as stumbling blocks to the talks beginning.
‘Failed Brexit project’
Industry leaders and Remain campaigners have been pushing for an EU-wide visa scheme to make it easier for young Britons to travel and work in the bloc, and vice-versa.
“This landmark intervention is a welcome acknowledgement of the immense impact that Brexit has had, not only on young Brits but young Europeans as well,” Naomi Smith, the chief executive of Best for Britain, said of the EU offer. “The UK Government must now respond in kind.
“Until a reciprocal EU-UK youth mobility scheme is formalised, our young people will continue to be robbed of the irreplaceable formative experiences their parents and older siblings enjoyed, because of a failed Brexit project they did not vote for.”
Charles Owen, of Seasonal Business in Travel, said: “A reciprocal youth mobility scheme is a win-win for both Europe and the UK, and in particular for struggling businesses suffering from chronic labour shortages, made worse by Brexit.
“Momentum is building to get this deal done and we implore the prime minister to talk to Industry and prepare for the upcoming negotiations appropriately. This is a serious economic and cultural opportunity for all.”
A Labour spokesperson said: “This is a proposal from the EU Commission to EU member states, not to the UK. It has come about because the UK government is reportedly approaching other European countries to try to establish mobility arrangements.
“Labour has no plans for a youth mobility scheme. We have already suggested some tangible ways that we would look to improve the relationship and deliver for British businesses and consumers, including seeking a veterinary agreement to tackle trade barriers, mutual recognition of professional qualifications and improved touring opportunities for artists.
“A Labour Government would seek to improve the UK’s working relationship with the EU within our red lines - no return to the single market, customs union or free movement.”
- European Union,
- Labour Party,
- Facebook Icon
- WhatsApp Icon
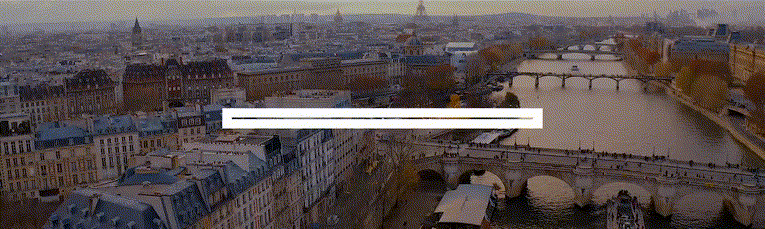
IMAGES
VIDEO
COMMENTS
As we saw earlier (Equation ( 12.1.8 )), the energy per unit volume in a harmonic wave of angular frequency ω and amplitude ξ0 is E / V = 1 2ρ0ω2ξ2 0. If the wave is traveling at a speed c, then the energy flux (energy transported per unit time per unit area) is equal to (E / V)c, which is to say. I = 1 2cρ0ω2ξ2 0.
The magnitude of the wave velocity is the distance the wave travels in a given time, which is one wavelength in the time of one period, and the wave speed is the magnitude of wave velocity. In equation form, this is. v = λ T = λ f. 16.1. This fundamental relationship holds for all types of waves.
A wave is a disturbance that propagates, or moves from the place it was created. There are three basic types of waves: mechanical waves, electromagnetic waves, and matter waves. Basic mechanical waves are governed by Newton's laws and require a medium. A medium is the substance a mechanical waves propagates through, and the medium produces an ...
A wave is just the phenomenon of oscillation of energy, using various properties of a medium such as physical, electro-magnetic, etc. For example, sound waves and light waves are both the carriers of energy, but a sound wave propagates through pressure variations, whereas a light wave travels by making use of electro-magnetic phenomena, which ...
When a wave travels through a medium-i.e., air, water, etc., or the standard reference medium (vacuum)-it does so at a given speed: this is called the speed of propagation. The speed at which the wave propagates is denoted and can be found using the following formula: v = fλ (1.5.1) (1.5.1) v = f λ.
And what we've essentially just generated is a sound wave travelling through air. So this right here is a sound wave. ... Our example of the string, this is called a transverse wave. Because the disturbance, the movement of the medium, is going in a direction transverse to-- or at an axis that's transverse to-- the direction of our movement. We ...
Traveling waves. A wave pulse is a disturbance that moves through a medium. A periodic wave is a periodic disturbance that moves through a medium. The medium itself goes nowhere. The individual atoms and molecules in the medium oscillate about their equilibrium position, but their average position does not change. As they interact with their ...
Traveling waves are observed when a wave is not confined to a given space along the medium. It is however possible to have a wave confined to a given space in a medium and still produce a regular wave pattern that is readily discernible amidst the motion of the medium. In such confined cases, the wave undergoes reflections at its boundaries which subsequently results in interference of the ...
Describing a Wave. A wave can be described as a disturbance in a medium that travels transferring momentum and energy without any net motion of the medium. A wave in which the positions of maximum and minimum amplitude travel through the medium is known as a travelling wave. To better understand a wave, let us think of the disturbance caused ...
wave motion, propagation of disturbances—that is, deviations from a state of rest or equilibrium—from place to place in a regular and organized way. Most familiar are surface waves on water, but both sound and light travel as wavelike disturbances, and the motion of all subatomic particles exhibits wavelike properties. The study of waves therefore forms a topic of central importance in all ...
Figure 2: The parts of the slinky in a longitudinal wave and the wave disturbance travel horizontally. Image credit: Adapted from OpenStax College Physics. Original image from OpenStax, CC BY 4.0. ... These new particles in turn move and bump into other particles, repeating the cycle of movement and imparting momentum, thus allowing the wave to ...
A wave is a disturbance that travels or propagates from the place where it was created. Waves transfer energy from one place to another, but they do not necessarily transfer any mass. Light, sound, and waves in the ocean are common examples of waves. Sound and water waves are mechanical waves; meaning, they require a medium to travel through.
a | The 6-12 Hz hippocampal theta oscillation, which appears during active behavioural exploration and rapid-eye-movement sleep, is a wave travelling from dorsal to ventral hippocampal CA1 (Refs ...
In mathematics, a periodic travelling wave (or wavetrain) is a periodic function of one-dimensional space that moves with constant speed. Consequently, it is a special type of spatiotemporal oscillation that is a periodic function of both space and time.. Periodic travelling waves play a fundamental role in many mathematical equations, including self-oscillatory systems, excitable systems and ...
By talking into one can, you generate a sound wave that travels through the string and reaches your interlocutor. This is possible because the string provides a means for the sound wave's energy ...
Patterning of place field firing by hippocampal travelling waves. a | The 6-12 Hz hippocampal theta oscillation, which appears during active behavioural exploration and rapid-eye-movement sleep, is a wave travelling from dorsal to ventral hippocampal CA1 (REFS 17,18). This flattened map view of the rodent hippocampus shows the positions of ...
This is known as the travelling wave. Define Travelling Wave (image will be uploaded soon) Before understanding what is travelling wave, let's understand waves. Wave can be defined as a disturbance in a medium that travels transferring momentum and energy without any actual movement of the medium. However, the medium must have elastic properties.
Moreover, travelling wave parameters vary across the datasets, with some exhibiting non-constant wave speeds and temporal shifts (e.g., Supplementary Fig. S5 for Gallego et al. 25). Consequently ...
d Traveling waves during movement imagery in the sensorimotor cortex. Alpha rhythmic activity during imagined movement in a representative individual. ... In a traveling wave, the phases of an ongoing oscillation are spatially organized, with a systematic phase shift across space in the cortex. Accordingly, to measure such patterns, we use a 2D ...
A travelling wave, on the other hand, is a wave in which the minimum and maximum amplitude positions move through the medium. In this Physics ... its particles begin to vibrate, transporting energy from one point to another without causing any overall movement. The result is a wave. Crack Agniveer Army Exams with India's Super Teachers. Get 3 ...
EU planning to ditch post-Brexit travel restrictions for UK citizens aged 18 to 30. The European Commission has proposed restoring much of the pre- Brexit freedom that allows British travellers ...
Iran has launched a wave of strikes toward Israel in retaliation for last week's deadly Israeli strike on an Iranian embassy complex in Syria, in an unprecedented move by Tehran that could ...
The probability of a neuron firing a spike at any given millisecond was low, and the peak of a traveling wave only marginally increased spiking probability (2.33% increase), resulting in only a ...
The breakthrough also triggered a wave of UAW organizing, the union says. Over 10,000 non-union auto workers have signed cards in support of the UAW in recent months and organizing campaigns have ...
Iran launched dozens of drones at Israel on Saturday but they will take hours to reach their targets, Israel's military said, an attack that may trigger a major escalation between the regional ...
The plans proposed by the EU would allow young people to stay in Europe for periods of four years, with the same rules extended to EU citizens coming to Britain. It would also mean EU students ...
Europeans aged 18 to 30 would be able to work, travel and study in UK and vice versa. The European Union has proposed a freedom of movement-style deal for young Europeans to appeal to a future ...